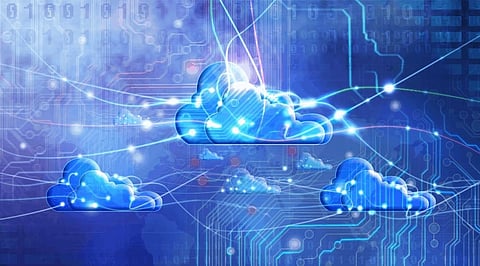
Researchers have been using high-performance computing (HPC) for highly computationally intensive tasks like drug discovery research, quantum mechanics, weather forecasting and more. Basically, it has been accelerating breakthroughs in autonomous driving, seismic engineering, oil and gas production, precision medicine, and financial risk assessment. While HPC can perform better when multiple HPC nodes are connected to form a cluster to offer exascale capabilities, some key hurdles may hinder from utilizing its maximum potential. These are processing vast datasets and storing these data and processed insights, all at the lowest power consumption levels.
It is well known that data has been anointed as the Oil or Currency of the new digital millennium. HPC would need larger memory to accommodate larger datasets along with persistent memory for storing data on the memory bus instead of storage drives that are synonymous with desktops at home or business laptops. Also to transform the data amassed from source points before allocating to storage spaces, one needs data analytics. Data analysis can be achieved using either traditional stand-alone computers or by moving data analytics to the cloud. But given the high volume, low veracity, high velocity, wide variety, and high value of data, knowledge extraction from new-age data is a complex process. When mapped along three independent vectors of volume, velocity, and variety gives us a better understanding of what can be referred to as big data. Besides, many other characteristics exist for big data, such as variability, viscosity, validity, and viability.
The opportunities for utilizing big data are growing in the modern world of digital data. Moreover, disruptive technologies like AI, machine learning (ML), natural language processing (NLP), computational intelligence (CI), and data mining are augmenting the big data analytics practices. Big data analytics describes the process of analyzing massive datasets to discover patterns, unknown correlations, market trends, user preferences, and other valuable information that previously could not be analyzed with traditional tools. Today we have data coming from hybrid sources like social sites, sensors, tweets, posts, blogs, GPS systems, smartphones, portals, online shopping patterns, credit-card usage patterns and countless other sources. These datasets can be grouped as location-aware data, person-aware data, context-aware data and more. This implies that data is currently getting unstructured and scattered. And that would also mean accumulating, processing and storing data is more expensive than ever.
Enter cloud computing. Since a few years, cloud platforms have been acting as an enabler for analytics delivery. This is why organizations are increasingly moving HPC workloads to the cloud. According to Oracle, HPC in the cloud is changing product development and research economics because it requires fewer prototypes, accelerates testing, and decreases time to market. Also, there are many other benefits to cloud platforms than storage space. These include load variability, minimal CapEx and OpEx, high-speed data processing with low or zero latency, quick transition between up-scaling and down-scaling, access to visual aids and dashboards, low cost of security, reduced TCO, integrated SMAC, high data aggregation, better resource cost reduction, and high throughput computing.
The analyst firm, Intersect360 Research has forecast that the HPC market will grow at a 7.1% compound annual growth rate through 2024, to reach US$55.0 billion at the end of the forecast period. And thanks to the delay in on-premise server and storage purchases during COVID, the cloud can further boost the HPC market in the coming years at a fraction of the costs. Additionally, as cloud technologies reach new level of maturity they become more appealing to HPC users. Cloud HPC users can also take advantage of auto-scaling and orchestration, development tools, big data analytics, management software and other advanced features of the public cloud. Moving HPC to cloud enables end-to-end management and traceability and easy data sharing, and collaboration across corporate boundaries, with existing cloud workflows.
As per a new Hyperion Research white paper, "Bringing HPC Expertise to Cloud Computing," one of the major drivers of increased cloud-based HPC usage is the recent availability of hybrid and multi-cloud options offered by many CSPs. These offerings are designed to provide HPC users with a near-seamless environment between on-premises hardware and counterpart cloud resources. Hyperion notes that prior to 2018, the percentage of HPC workloads running in the public cloud had plateaued at approximately 10% of the overall HPC workload portfolio, and this jumped to 20% in 2019.
To maximize output from establishing cloud HPC, big data analysts should monitor utilization and workloads both on-premise and in the cloud and analyze historical workload patterns, scheduling and cloud bursting policies. While cloud allows HPC users to spin up specific architectures for each application; resource requirements may vary as per need of cores, memory, storage, and GPU capabilities. Therefore, it is important to predict these necessities and demands by analyzing resource requests vs. actual resource consumption to figure out the emergency resource requests. It is vital to aim for running only workloads that specifically need to be run in the cloud. At the same time, the main research cluster benefits from cost savings from not having to provide this specialized technology. By having applications request only what they need, more workloads can run simultaneously, delivering higher throughput. Lastly, to enforce cloud automation for carrying out various tasks, HPC users must ensure that the cloud data analytics model is trained on extensive datasets for better outcomes and decisions that do not ask for human intervention.
Join our WhatsApp Channel to get the latest news, exclusives and videos on WhatsApp
_____________
Disclaimer: Analytics Insight does not provide financial advice or guidance on cryptocurrencies and stocks. Also note that the cryptocurrencies mentioned/listed on the website could potentially be scams, i.e. designed to induce you to invest financial resources that may be lost forever and not be recoverable once investments are made. This article is provided for informational purposes and does not constitute investment advice. You are responsible for conducting your own research (DYOR) before making any investments. Read more here.