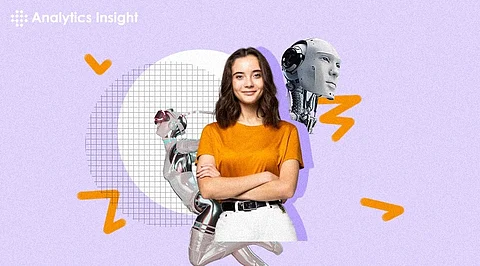
The role of feedback loops in training AI is crucial. They are a tool for adjusting and improving performance over time. These loops enable AI models to learn from their mistakes, adapt to situations that change internally, and ultimately increase their accuracy and efficiency in various applications that introduce the visual barrier.
To achieve user trust, an AI system not only needs to deliver correct decisions, but it needs to be understood. This is important because not seeing how AI systems arrive at decisions can lead to ineffective feedback.
Let's take a closer look at the importance of feedback loops in AI training.
Feedback loops facilitate repetitive learning in which AI models receive input, provide output, and receive feedback on the accuracy of their predictions. They then use this feedback to refine the model's parameters, and its internal representations are updated to improve performance incrementally. It is one of the roles of feedback loops in training AI, which is implemented to enhance performance.
By analyzing feedback signals, AI models can distinguish mistakes and regions for change, empowering them to refine their expectations and move forward with their decision-making forms. Reaction procedures through continuous emphasis, AI frameworks can decrease errors and make strides in efficiency
In dynamic situations where data dispersion can alter over time, input circles play a pivotal part in empowering AI models to adjust and improve. Taking input from continuous intuition with the environment, our environment, counting Artificial intelligence frameworks, will be able to alter their techniques and decision-making forms in real-time, guaranteeing strong execution in advancing scenarios.
Analytics software has seen advancements in AI capacities, presently consolidating a wide range of tools and features planned to capture and understand client feedback in continuously more complex ways. Assumption investigation devices, for example, utilize machine learning and natural language processing (NLP) to recognize passionate connotations in literary feedback.
Creating AI feedback loops in intellect is one of the roles of feedback loops in training AI. This gives you an intelligent understanding of the viability of your AI framework and patterns in client input. Visual aids such as charts and charts in estimation examination help track changes in shopper assumption over time, giving a clear and instinctive view of information trends.
Adding AI feedback loops to your exchange shapes can lift the viewpoints of your operations altogether. For example, AI-advancing gadgets like Salesforce's Einstein GPT and HubSpot's CRM utilize primary information centers to donate correct assessment, personalized communication, and capable communication without physically entering data.
Feedback information also plays a crucial part in tending to ethical considerations and reducing predisposition in AI training. By suitably seeking input from unmistakable accessories and analyzing the impact of AI choices on particular estimation groups, organizations can recognize and address inclines and tolerability in AI-powered applications.
Conclusion: The role of feedback loops in training AI is a noteworthy component. By saddling the control of input, AI frameworks can refine their calculations, optimize their effectiveness, and execute more correct and impactful courses of action over a wide range of spaces and applications.