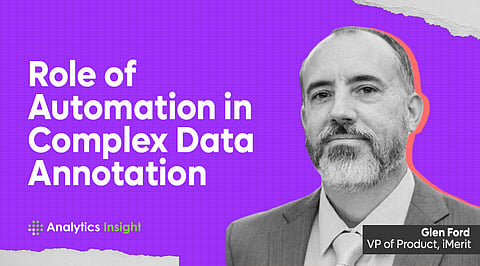
Unstructured data accounts for about 80% of the data generated by the average business – emails, presentations, audio, video, documents, and images. Data annotation and labelling services play a vital role in building specific technologies for both computer vision annotation and natural language annotation.
The most common, oldest, and simplest approach to data labelling is, of course, a fully manual one. A human user is shown a series of raw, unlabelled data (such as images or videos), and is tasked with labelling it according to a set of rules. As the field progressed, AI models were introduced as the need for data to make real-world predictions grew. For example, for a car to drive itself, you need huge volumes of data to train the AI and ML models to understand the environment better. These models need training to precisely read the surroundings, road conditions, traffic signals, people and animal movements and a lot more.
Data annotation provides more context to datasets; it enhances the performance of exploratory data analysis as well as machine learning (ML) and artificial intelligence (AI) applications to upscale a business. Businesses from agriculture, autonomous mobility, defence, mining, insurance and many other sectors, use data annotation services to gather data and derive insights for better decision making. AI/ML models can affiliate with existing applications for processing unstructured data and triggering a response to optimize workflows.
Conventionally, skilled talent ably collects unstructured data and converts it into structured data sets for feeding AI/ML systems. Automation of labelling adds another dimension to the process and makes the job of an annotator easier and more efficient. Automation, in this case, includes applying ML to annotate, label and enrich datasets. Automation and humans in the loop combine to build a more productive and efficient process of data annotation. This combination of human and machine intelligence provides companies with greater context, quality, and usability. Specifically, you can expect:
Data annotation plays a key role in making sure AI or ML projects are scalable. Training an ML model requires it to recognise and detect all objects of interest in raw inputs for accurate inferences. Depending on the project requirements, various techniques and types of data labelling can be applied.
The human intelligence required during data annotation is indispensable. ML and AI can increase overall productivity by always having a human in the loop. For example, at the very beginning, a new model tries to annotate an image. With a human in the loop, any initial errors made by the model can be fixed, thus enriching ML's ability to annotate data. Similarly, the model can be taught pre-labelling, where the model or AI takes the first pass and the human corrects it. There may also be instances of machine-catching inaccuracies committed by humans based on similarities to other people's work. ML pre-labelling models continue to advance and improve throughput on human labeling, while also increasing quality. More types of automation are emerging all the time.
A recent trend shows customers reconciling and managing datasets after the annotation process and even before it. Visual similarity search powered by ML helps data scientists discover and focus on the best data to send for human labeling. For example, when the annotator finds some interesting case, like a stop sign covered in snow that needs to be annotated with a certain classification that the data scientist hadn't anticipated, similar instances can be searched for. New instances of the edge case can even be synthesized, boosting the resulting signal gain. These techniques multiply the impact of edge case annotation.
Data annotation is a critical success factor behind AI and ML algorithms. Highly accurate ground truth directly impacts algorithmic performance. Automation of this process is critical for high precision quality at scale.
Glen Ford – VP of Product, iMerit