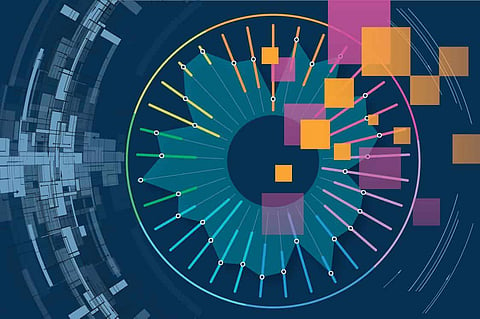
Researchers at Massachusetts Institute of Technology (MIT) and Qatar Computing Research Institute (QCRI) have developed a model powered by AI. The AI model is designed to tag road features in digital maps using satellite imagery. This AI-driven RoadTagger model combines a convolutional neural network (CNN) and a graph neural network (GNN) to automatically envisage the number of lanes and road types concealed by obstructions, improving GPS navigation, especially in countries with limited map data.
The model helps drivers in incorporating information about parking spots, while mapping bicycle lanes that can assist cyclists to negotiate busy city streets. Providing updated information on road conditions, the RoadTagger model can also improve planning for disaster relief.
Unlike other GPS navigation systems, RoadTagger makes use of an amalgamation of neural network architectures to automatically predict the number of lanes and road types, including residential or highway, even when roads can be blocked by trees or buildings.
Sam Madden, a professor in the Department of Electrical Engineering and Computer Science (EECS) and a researcher in the Computer Science and AI Laboratory (CSAIL) says, "Most updated digital maps are from places that big companies care the most about. If you're in places they don't care about much, you're at a disadvantage with respect to the quality of map. Our goal is to automate the process of generating high-quality digital maps, so they can be available in any country."
When testing RoadTagger on occluded roads from digital maps of 20 US cities, the model reckoned lane numbers with 77 percent accuracy and inferred road types with 93 percent accuracy. Also, the researchers are planning to enable the model to foresee other features, such as parking spots and bike lanes.
The model relies on CNN and GNN, where GNNs form relationships between connected nodes in a graph, CNNs take as input raw satellite images of target roads. RoadTagger is based on an end-to-end model, meaning it is fed only raw data and automatically generates output, without human intervention. This combined architecture of CNN and GNN signifies a more human-like intuition, researchers noted.
Reportedly, the GNN breaks down the road into nearly 20-meter segments, or tiles. That each tile is a separate graph node, associated by lines along the road. And for each node, the CNN extracts road features and shares that information with its instant neighbors. Road information then promulgates along the whole graph, with each node receiving some information about road attributes in every other node. And if a certain tile is obstructed in an image, RoadTagger utilizes information from all tiles along the road to predict what's behind the obstruction.
"Humans can use information from adjacent tiles to guess the number of lanes in the occluded tiles, but networks can't do that," Sam said. "Our approach tries to mimic the natural behavior of humans, where we capture local information from CNN and global information from the GNN to make better predictions."
Join our WhatsApp Channel to get the latest news, exclusives and videos on WhatsApp
_____________
Disclaimer: Analytics Insight does not provide financial advice or guidance. Also note that the cryptocurrencies mentioned/listed on the website could potentially be scams, i.e. designed to induce you to invest financial resources that may be lost forever and not be recoverable once investments are made. You are responsible for conducting your own research (DYOR) before making any investments. Read more here.