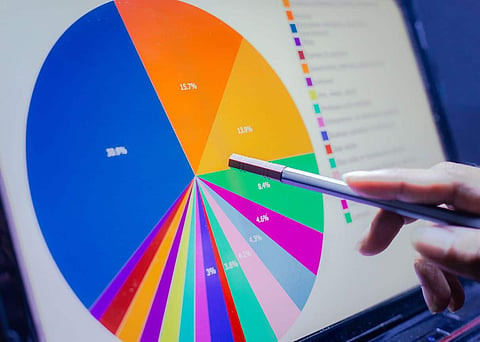
Predictive Analytics is presently one of the most significant big data trends. Predictive Analytics is a subset of Business Intelligence (BI) and Business Analytics (BA). BI and BA are often used interchangeably, although there are differences in the question and methodology. In essence, business analytics represents a more advanced level of evolution of BI. However, business intelligence is often used as a generic term for all forms of data analytics in the enterprise.
Knowing what's going to happen today – predictive analytics can make sales strategies or business models more successful. The concept is already part of everyday business life but what is the future of predictive analytics in the coming years?
Data is the new gold. But unlike the precious metal, information is worthless if you lock them in a closet and let them lie. An increase in the value of the rapidly growing renewable raw material occurs only through preparation, analysis, and use. Anyone who knows how to draw the right conclusions from the results will find it easy to make decisions that will bring the company forward.
Is the data already available in companies suitable for predictive analytics? Various studies show that data quality problems still exist, especially in large companies. Large enterprises use too many systems that make data harmonization difficult. On the other hand, data quality is not yet anchored in the department. Many do not realize why some fields in the systems need to be filled out because they do not know what to do with them afterward.
It is precisely the department that has both the data and the questions that allow an analysis. Playing the data ball of IT is therefore not very useful. What it takes is the realization that data science is a team sport that requires other skills. It needs people who are familiar with both data preparation and the technical background of data. These are the prerequisites for being able to obtain added value from the analysis results.
Some SMEs can now afford analysis capabilities that, formerly, only governments could provide. The technical costs have indeed collapsed and allow most companies to have computing power, storage capacity, and software or development platforms necessary to achieve accurate predictive analytics.
One solution gaining now lots of traction is in-memory computing technology which enables storing data in-memory itself thus enabling completing complicated transactions in matter of seconds.
In particular, the drop in the cost of storage avoids having to ask the question "I keep, or I throw" for each type of data, thus allowing everything to be kept. As a result, most companies can engage in predictive analytics without breaking the bank.
Achieving predictive analytics is first and foremost not only a question of data, but also tools. You have to model behaviors, which can take the form of a curve from correlation calculations to a much more sophisticated algorithm. Predictive analytics is booming not only in many more businesses but also in new areas. Typically, the preventive maintenance of mechanical parts in the transport relies more and more on this kind of treatments to stop making interventions at regular intervals but when it is relevant.
Big data has contributed to the generalization of predictive analysis. The classical analytics content itself with studying the past to understand what has taken place. Because the cost of technical solutions, especially storage, have fallen, the volumes reviewed can be much more critical and thus the analysis can be predictive or even prescriptive.
There is indeed a nuance between predictive analysis ("this is what will happen") and the customary ("what to do"). The latter is not content to indicate the expected evolution but also to suggest what solution to adopt.
With the dominance of social networks and mobile broadband access, most of the data that is shared and shared around the world is generated by the users themselves: blogs, social networks, image sharing, music, and video sharing sites.
In parallel, smartphones have become personal control hubs for all connected objects (activity trackers, connected home, connected car, etc.). It is estimated that on average one individual has 2.5 devices. This huge mass of structured and unstructured data is growing exponentially and can be understood as the generic term big data.
Faced with the excitement of big data, CIOs had to look differently at their policy of storing data from a multitude of sources. Today business data comes from sensors installed in factories and production tools, connected objects, web and mobile traffic, social media. But taken in isolation, all these data have only a value "by weight", that is to say marginally. Value is created only with contextualization when we manage to group them, put them in relation and decrypt them to make sense.
While companies were already manipulating and exploiting their data with business intelligence techniques, the new informal and unstructured data types quickly revealed the shortcomings of these traditional tools in terms of power, speed, flexibility and to learn from big data. This break explains the arrival of advanced analytical tools that analyze previously unimaginable volumes of data to provide precise answers to the questions asked.
The exploitation of big data answers three significant challenges for each company: improving operational/industrial performance, economic performance, customer knowledge.
Historically, statistics were used to perform simple counts and analyze frequencies. Then data mining began to relate different phenomena while taking into account larger volumes of data, but mainly accessible and structured.
Today, using algorithms, machine learning, artificial intelligence, and data science, the predictive analysis makes it possible to exploit all types of data to implement new models of interpretation and prediction, to derive forecasts on future developments and make recommendations on the actions to be taken. Let's mention the most common examples:
• Predictive Maintenance: Analyze the signals of the production line to anticipate failures and change parts before the failure occurs.
• Fraud detection: detect fraud or failure to act before it happens.
• Analysis of customer behaviors: understand their motivations, identify the best ways to prospect and retain them to offer each of the personalized services at the right time by the most appropriate channel.
Predictive analytics is now used in many enterprises with countless success, not just in the security area. The smart grid of the future, for example, provides load forecasts and predicts the current requirement to bring the power consumption and the fluctuating power generation (solar and wind power plants) in line. For credit scoring, banks estimate the likelihood or risk that a client could not have the funds for the future repayments of a granted loan.
An example from the business is predictive maintenance. Various sensors communicate data on the status of a plant such as power, revolutions, temperature, and utilization to a cloud platform. The solution analyzes characteristics of the usage status, wear, and various condition from multiple sources, identifying error and fault patterns and substandard components.
The service can respond promptly and proactively avert expensive machine failure by installing a new replacement part or preferring maintenance. Web shops could analyze the previous buying behavior of a customer and propose other products to the customer.
There will be many instances of predictive analytics that can be used in the future. Predictive analytics is a continuous and iterative process. As the predictive models used continue to advance and adapt as they progress, analytics predictions become more and more accurate. To fully utilize the accumulated potential of their data and gain a competitive advantage, companies must quickly integrate new analytical methods into their overall strategy.
Join our WhatsApp Channel to get the latest news, exclusives and videos on WhatsApp
_____________
Disclaimer: Analytics Insight does not provide financial advice or guidance. Also note that the cryptocurrencies mentioned/listed on the website could potentially be scams, i.e. designed to induce you to invest financial resources that may be lost forever and not be recoverable once investments are made. You are responsible for conducting your own research (DYOR) before making any investments. Read more here.