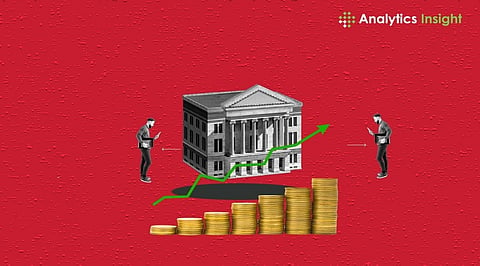
Banking is a competitive and dynamic sector that demands ongoing innovation and consumer satisfaction. One of the most difficult issues for banks is identifying and retaining lucrative clients who are likely to subscribe to their deposit products, such as term deposits, savings accounts, and certificates of deposit. These solutions help banks create consistent and recurring revenue while also increasing client loyalty.
However, forecasting which consumers would subscribe to a deposit product is difficult since it is dependent on several factors, including customer demographics, behavior, preferences, and financial status. Furthermore, clients may alter their views or move banks due to market conditions, offers, or recommendations.
As a result, banks must harness the power of machine learning (ML) and analytics to create prediction models that can effectively categorize consumers as future subscribers or non-subscribers and give insights into the variables that impact their decisions. Machine learning (ML) is a type of artificial intelligence that enables computers to learn from data and make predictions or decisions without explicit programming. Analytics is the act of processing, analyzing, and interpreting data to provide insights and inform decision-making.
Predicting bank deposit subscriptions using machine learning and analytics is a beneficial and viable option for banks looking to gain a competitive advantage while also increasing customer happiness and loyalty. Using machine learning and analytics, banks may not only boost revenue and profitability but also improve client relationships and trust.
Join our WhatsApp Channel to get the latest news, exclusives and videos on WhatsApp
_____________
Disclaimer: Analytics Insight does not provide financial advice or guidance on cryptocurrencies and stocks. Also note that the cryptocurrencies mentioned/listed on the website could potentially be scams, i.e. designed to induce you to invest financial resources that may be lost forever and not be recoverable once investments are made. This article is provided for informational purposes and does not constitute investment advice. You are responsible for conducting your own research (DYOR) before making any investments. Read more here.