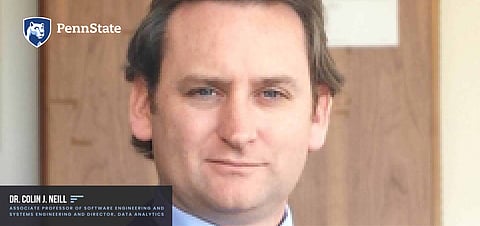
Analytics is a vast educational space in which the data deluge is hitting every discipline and the opportunity for insight from that data is exciting everyone. Recognizing that, Penn State University designed the Data Analytics program to easily expand into specific application areas and disciplines by creating degree options. The students from Penn State's Software and Systems Engineering Division take statistics, data mining, and predictive analytics coursework, but they can then focus on designing and building analytics systems, using analytics for business, or analytics in marketing. Every student can subsequently tailor their curriculum with electives in topics including Python programming, deep learning, social network analytics, data visualization, social demography, and advanced statistics.
Pedagogically, Penn State is grounded in a student-centered instructional approach, a flipped classroom as it is often referred. So instead of heavy didactic instruction, the university focuses on experiential and active learning. In practice, this means, each lesson works as a roadmap for the student guiding them through the material at an appropriate pace and staged with formative self-study activities, short multimedia elements for exposition, and individual and group practical assignments utilizing real-world data as much as possible to ensure that students really master the material. This is all facilitated by a faculty expert in the discipline and engaged in the student's learning through a number of modes of communication including email, chat, discussion forums, and tele/video conferencing.
In a fast-paced discipline where there is a constant change of tools and platforms deployed in the program — there's always a new update to the Apache Hadoop ecosystem. But right now, the lists of tools include most of that ecosystem – Hive, HBase, Pig, etc. In addition, the Data Analytics program includes a lot of Oracle tools; IBM's Big Data Analytics; Python toolkits (Pandas, SciKit-Learn); NoSQL databases such as MongoDB and Cassandra; and visualization tools such as Tableau and Gephi for its students.
On the machine learning and artificial intelligence side, the program covers supervised and unsupervised learning, data mining and classification, neural networks, deep learning networks, and super game playing to name a few. Tools used include KNIME, WEKA, Keras, NumPY, and AlphaGo.
Finally, on the statistics, front students learn about descriptive analytics, regression, multiple regression, ANOVA, time-series analysis and statistics for social science using R, SAS, SPSS, and Minitab.
Penn State certainly does not consider its program training limited to these tools, but it is quite impossible to separate the techniques from the tools in practice, and students appreciate gaining hands-on experience in using the platforms that are used commercially.
Professor Dr. Colin J. Neill is the Founding Director of the master's degrees in Data Analytics at Penn State.He leads a team of faculty from across the Smeal College of Business, the Eberly College of Science, the College of Engineering, and the School of Graduate Professional Studies in the development of the program. The course was launched online through Penn State World Campus in 2016 and residentially in 2017 with over 400 students having enrolled so far and around 100 students who have graduated.
Professor Dr. Colin J. Neill's own journey in this area started as a graduate student in the mid '90s in the Real Time Artificial Intelligence Research Group of the University of Wales Swansea. His Ph.D. supervisor, Professor Michael Rodd, founded the group to investigate ways of making artificial intelligence useful in real-time, mission-critical applications. The group developed approaches for using neural networks and expert systems for things like control systems for railways, instrument landing systems for aircraft, and machine vision inspection systems. AI fell out of favor for some time though and Neill's own research moved into software and systems engineering. "It has been fun to see the re-emergence of machine learning with the advent of big data analytics and it is exciting to witness the research the university's Big Data Lab is currently pursuing, he said". One project Professor Dr. Colin J. Neill is especially excited about is using network analytics approaches to identify critical elements in large-scale systems – elements that, if compromised, could threaten the entire system. The university has found this true in software systems, heterogeneous engineered systems, and even organizational systems.
The Data Analytics program itself has been phenomenally successful and grown at a rate that has surprised everyone. This has allowed the university to expand its faculty strength in the discipline and launch a research-oriented master's degree to complement the initial professionally-oriented program. The most satisfying achievements, however, are those of its students. One of the students, Heather Myers, won the Tableau Student Viz Contest in 2017 which is an international competition with over 250 submissions from around the world. Teams of the university's students have also been successful in hackathon-type competitions including securing the second rank at the 2018 ASA Datafest by working on a 14 million row dataset from Indeed.com, and winning the SAP's Veterans Challenge Use Case Award at Code4PA in September 2018. These achievements demonstrate not only that the program is able to attract excellent students but also that its curriculum is preparing students to solve thorny, real-world problems.
When the degree program was proposed there were about two dozen similar programs across the US. Just 3 or 4 years later, there are at least twice as many now, which speak to the need in the marketplace for data-savvy professionals. A word of caution to the prospective students, though, to really do their due diligence in examining programs, as in Professor Dr. Colin J. Neill's opinion a large number of the degree programs emerging now are trying to capitalize on the analytics term, but really only providing a preparation in what the buzz terms mean, rather than in how to design, build, and use analytics systems.
INFORMS and the American Statistical Society, the two professional societies, most closely aligned with data science and analytics, are clear about the technical and analytical skills required for a data analytics professional and the program is designed around those needs – computational statistics, machine learning and data mining, technology platforms for data collection, cleaning, storage and retrieval, and critically, the ability to frame business problems as analytics problems.
The big data phenomenon is described by 5 Vs — volume, velocity, variety, veracity, and value. Data is generated in greater volume and faster than ever seen before, think of the constant streams of data coming from sensor networks that sample their environment thousands of times a second or the volumes of tweets generated following a socially meaningful event, for example. Add in that the industry is now interested in greater varieties of data than ever before with images, videos, audio recordings as well as natural language expressed as text, tweets, etc. These all require new technologies and platforms for the storage, retrieval and processing of the data that's why Hadoop, MapReduce, and NoSQL, are in vogue. In addition, there is a need to explore new ways to assess the quality of the data, to clean it by identifying errors and correcting them or processing it knowing that the level of quality we would like is not attainable at those volumes and velocities. Finally, Penn State does this with the sole intent of uncovering meaningful insights that aren't otherwise obvious or even knowable to create value. These are all new challenges which require specialized programs that embrace them holistically.
Penn State uses real data as much as possible. "In fact, considering the scale of the datasets we must use for the student experience to be meaningful, we have no alternative – it would be close to impossible to artificially generate those datasets with all the peculiarities found in real data, said Dr. Colin. "So, we use public datasets such as those made available by research agencies like the NSF and NIH, as well as state and federal government agencies – the Code4PA contest our students won, for example, used data on the opioid crisis made available by the PA Open Data Portal." In addition, the university's faculty collaborate with private industry who share their data including fostering partnerships with United Airlines, GSK, CitiBike and OSIsoft to name a few.
Students at the program not only love the data because it is real but also that the problems it creates for them determines what pre-processing must be performed, selecting the tools and techniques for analysis, building models from real data and assessing the quality of those models, and finally generating insights that no-one has uncovered before. It is that sense of discovery that draws students into the discipline in the first place. It's exciting for them and the university.
Join our WhatsApp Channel to get the latest news, exclusives and videos on WhatsApp
_____________
Disclaimer: Analytics Insight does not provide financial advice or guidance. Also note that the cryptocurrencies mentioned/listed on the website could potentially be scams, i.e. designed to induce you to invest financial resources that may be lost forever and not be recoverable once investments are made. You are responsible for conducting your own research (DYOR) before making any investments. Read more here.