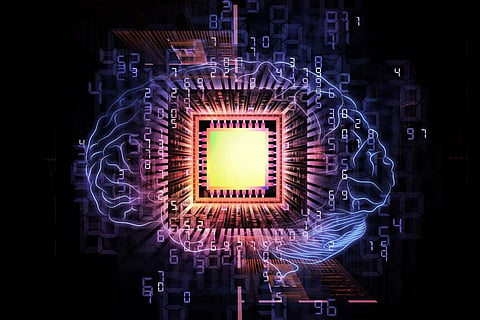
Thanks to advancements in modern technologies, human and artificial brain interactions are becoming more and more synonymous. As traditional computers are slowly being dysfunctional to cater to the data processing demands of the disruptive age, scientists are exploring new ways to resolve this snag. While artificial intelligence (AI) has evolved to answer the new data analysis, processing, and other computational expectation, it still falls deficit in scenarios which do not align with rules and emulated classical logic or self-learning by perception for deriving reasoned conclusions, key traits that defined first and second age of AI. Hence, it is presumed that the next generation of AI will be about drawing parallels to the human thought process, like interpretation and autonomous adaptation by mimicking neurons spiking like the nervous system of humans, otherwise termed as neuromorphic computing.
This term was first coined by American professor Carver Mead back in the 80s which describing computation mimicking the human brain. NIST claims that neuromorphic computing can dramatically improve the efficiency of important computational tasks, such as perception and decision making. This is achieved by facilitating the highest computing speeds while reducing the need for bulky devices and dedicated buildings. In humans, the neurological processes are carried through the chemical or electrical impulse relays across neurons; in neuromorphic computing it is done by using spiking neural networks (SNNs) and neuromorphic chips (hardware).
Recently, a team of researchers at Penn State, attempted to pioneer towards transmitting the neural network structure in the human brain and the analog nature of our brains to computers. As per their findings published in Nature Communications, titled "Graphene Memristive Synapses for High Precision Neuromorphic Computing," the team discovered that graphene-based memory resistors (memristor) show promise for this new computing form. Memristor is a nascent electronic device that exists in conductance/resistance states that depend on the amount of charge that has flowed through them. Since memristor can exist in many different states, it can be used to substantially improve the performance of artificial neural networks – systems that could someday rival and even replace conventional computers.
Saptarshi Das, the team leader and Penn State assistant professor of engineering science and mechanics, says, "We have powerful computers, no doubt about that, the problem is you have to store the memory in one place and do the computing somewhere else."
"The brain is so compact that it can fit on top of your shoulders, whereas a modern supercomputer takes up space the size of two or three tennis courts," explains Thomas Schranghamer, a doctoral student in the Das group and first author on the paper.
"We are creating artificial neural networks, which seek to emulate the energy and area efficiencies of the brain," he continued.
In addition to Das and Schranghamer, the additional author of the paper is Aaryan Oberoi, a doctoral student in engineering science and mechanics.
The team created a graphene-based memristor, which has 16 conductive states that can be reliably stored and readout. In this device, the data and processing capacity are loaded into a graphene layer, by applying a brief electric field to the sheet of graphene, which is a thick layer of carbon atoms. Further, the use of this data and process capacity is shaped according to which region and how intensity the electricity supplied in the process is carried out. This is how, multiple memory states can be kept on a single graphene surface. The team believes this technology can be made available on a commercial scale. With many of the largest semiconductor companies actively pursuing neuromorphic computing, Das believes they will find this work of interest.
Unlike the fixed states in conventional memristors, the team also demonstrated that these graphene-based memristors could be easily programmed to have arbitrary conductance values. This implies that such flexibility can be resourceful while creating ANNs.
Apart from this research, there has been significant development in the field of neuromorphic hardware chips. For instance, Intel's Intel Labs's Loihi, which is its fifth-generation self-learning neuromorphic research test chip, was introduced in November 2017. Loihi is a 14-nanometer chip with a 60-millimeter die size and contains over 2 billion transistors and three managing Lakemont cores for orchestration. It contains 128 cores packs and a programmable microcode engine for on-chip training of asynchronous spiking neural networks (SNNs). TrueNorth chip from IBM also has outstanding features like 4096 cores, where each core contains 256 numerals (about 1 million new rooms and over 250 million synapses).
Join our WhatsApp Channel to get the latest news, exclusives and videos on WhatsApp
_____________
Disclaimer: Analytics Insight does not provide financial advice or guidance on cryptocurrencies and stocks. Also note that the cryptocurrencies mentioned/listed on the website could potentially be scams, i.e. designed to induce you to invest financial resources that may be lost forever and not be recoverable once investments are made. This article is provided for informational purposes and does not constitute investment advice. You are responsible for conducting your own research (DYOR) before making any investments. Read more here.