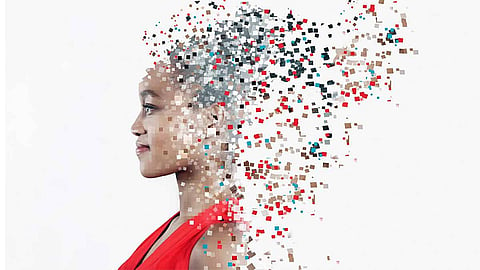
As we know, digital transformation has taken over the world, the worldwide investment on AI technology is said to hit $35.8 billion in the running year, according to IDC. The spending will aid the critical initiatives undertaken for business survival.
While looking at the different side of the aspect, we observed that most of the organizations working on AI and ML project are running into problems, depicts a report by Dimensional Research. Around 96% of companies that is almost 8 out of 10 companies face problems with data quality, data labeling which are necessary to train AI and construct model confidence.
The report presented by Dimensional revealed a different aspect of the AI centric projects. The study was conducted on behalf of Alegion, which surveyed nearly 227 technology professionals. These professionals were actively involved in AI/ML projects. The report discovered that an organization faces a hard time while keeping up with a large set of data and AI and ML systems.
Nathaniel Gates, CEO, and co-founder of Alegion said, "The single largest obstacle to implementing machine learning models into production is the volume and quality of the training data. This research reinforces our own experience, that data science teams new to building ROI-driven systems try to tackle training data preparation in house, and get overwhelmed."
Reportedly, the system can witness issues while processing a large volume of data but to get the AI system started, in contrast, an ocean of data is required. The data science team at such organizations are bound to deliver successful projects operating over a lot of data. The projects are also expected to process specific quantities of information while working on the system.
The study also shows the responses of some professionals who have put forward a few ways to combat this challenge. Around 76% of them said that they try to label and elucidate training data on their own. Also, 63% of them said that they try to build their own labeling and elucidating automation technology. Additionally, 71% of the teams confirmed that they outsource training data and other ML project activities.
Join our WhatsApp Channel to get the latest news, exclusives and videos on WhatsApp
_____________
Disclaimer: Analytics Insight does not provide financial advice or guidance. Also note that the cryptocurrencies mentioned/listed on the website could potentially be scams, i.e. designed to induce you to invest financial resources that may be lost forever and not be recoverable once investments are made. You are responsible for conducting your own research (DYOR) before making any investments. Read more here.