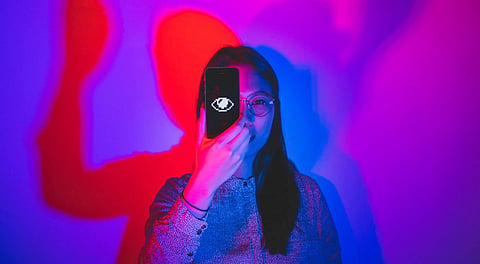
-------
Gartner predicts that by 2022, 80% of cell phones available will have on-device AI, and that is up from only 10% in 2017. AI and data processing in the cloud won't leave, yet on-device AI is what is making connected devices, including autos, HD cameras, cell phones, wearables, and other IoT gadgets, more smarter and faster.
With on-device AI, voice assistants are progressively intelligent and valuable, and vehicles are more secure without the microsecond slack when interfacing with the cloud. Security is upgraded, robotics can take creative jumps, and healthcare services and results are improved. At the point when your AI power really rests in your grasp, unwavering quality turns into a super-power, since it never again relies upon system accessibility or bandwidth.
A part of AI, machine learning (ML) utilizes advanced algorithms in models that can gain from information and distinguish significant patterns. By revealing connections, ML enables organizations to settle on better decisions without the requirement for human input.
Today, ML is fueling a wide range of uses, a significant number of which are mobile, as cell phone users move to a foreseen 3.8 billion by 2021. Models run from unique fingerprint recognition and photograph sorting to increasingly creative use cases.
An AI-driven social robot for senior natives utilizes ML to comprehend the preferences, conduct, and identity of its owner. In light of these communications, the robot can naturally connect more seasoned grown-ups to stimulating digital content, for example, music or audiobooks, just as suggest activities, remind the client about up and coming appointments, or interface with family and companions through social media platforms. Also, in contrast to most AI frameworks, which require voice actuation, the robot proactively speaks with its client. For instance, if a senior native has been sitting for an all-encompassing timeframe, the robot can naturally prescribe calling a friend or going for a stroll.
An intelligent camera framework distinguishes crowds of reindeer through ML algorithms as they approach train tracks in remote parts of Norway where the creatures are regularly unnecessarily slaughtered. By handling data on the device itself, the system can caution train administrators progressively to lessen speeds when the creatures are available, subsequently anticipating mishaps and train delays.
The manner in which AI has turned into the competitive differentiator for organizations that want to remain ahead in their industry as well as disrupt it, on-device AI is quickly offering a bit of leeway for organizations that need to snare customers on the propelled abilities the innovation offers.
Around 66% of cell phone users are grabbing their gadgets like clockwork; more than 20% are on their telephones at regular intervals. Purchasers as of now depend intensely on AI applications that have turned out to be basic day by day devices, from virtual assistants like Alexa and Siri to the traffic forecast and travel planning power of Google Maps.
Also, presently on-device AI implies that cell phone assistants are getting more splendid constantly, with logical discussions, improved noise suppression, and immediate, on-the-fly language interpretation. With video and picture social cash on stages like Twitter and Instagram, shoppers are pulled in to the stepped-up cell phone cameras with refined on-device computer vision applications that enable them to do considerably more, and users are updating their gadgets just to get their hands on these applications.
Hardware vendors are observing and progressively equipping devices with ML-skilled chips. Thus, these gadgets are catching and processing information real-time, giving immediate situational analysis, recognizing patterns, and supporting speedy AI-empowered decision making.
Edge AI gadgets are for the most part running ML interference workloads at hand—where certifiable information is compared with a trained model. The models they use are for the most part worked in the cloud because of the overwhelming compute requirement of building an AI model. However, even with AI training, we are beginning to see edge devices utilized as trainers as they learn in certifiable situations.
The planning couldn't be better. We've achieved a critical mass of compute resources in the cloud. Around 29 billion connected gadgets are anticipated by 2022, of which about 18 billion will be identified with the Internet of Things. In the meantime, the average purchaser will claim 13 connected devices by 2021 as self-ruling vehicles populate our streets and sensors spread from manufacturing plant floors to rural ranches, each competing for valuable compute power.
These are early days for smart gadgets. We're in the initial period of the AI and machine learning upheaval. However, use cases are quick advancing from voice recognition and photograph filters to life-sparing gadgets, driving the demand for remarkable compute power. Moving ML workloads to the edge can help improve performance and productivity. But, cautiously thinking about which programming approach is ideal, and on which platform, is the thing that will, at last, ensure staying in the race.
Join our WhatsApp Channel to get the latest news, exclusives and videos on WhatsApp
_____________
Disclaimer: Analytics Insight does not provide financial advice or guidance. Also note that the cryptocurrencies mentioned/listed on the website could potentially be scams, i.e. designed to induce you to invest financial resources that may be lost forever and not be recoverable once investments are made. You are responsible for conducting your own research (DYOR) before making any investments. Read more here.