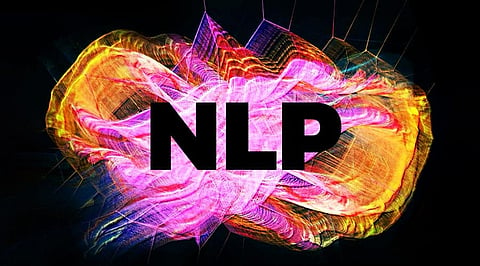
As per the 2020 NLP Industry Survey, over three-quarters of natural language processing (NLP) users rely on a cloud NLP service. Cloud NLP workloads are on the rise. However, there are limitations to using the technology in the cloud," claims Ben Lorica, one of the authors of the study.
According to Lorica and Paco Nathan's 2020 NLP Industry survey, in the current scenario, this is a great time to use NLP technology to process and analyze text. John snow Labs, developer of the open-source Spark NLP library that's used in the healthcare industry, sponsored the survey.
The budgets for NLP use cases are growing quite a bit for starters. The capabilities, accuracy, and scalability of NLP models and services among many are based on neural networks; have increased, states Lorica, the Principal of Gradient Flow Research and Chair of the upcoming NLP Summit.
Lorica says, "NLP is a lot more accurate and a lot more scalable. You have a lot more options as far as tools, and the number of people who are familiar with these tools has grown so much, as opposed to when I was using these things ten years ago."
As compared to two years ago, it has become easier for non-experts to choose an NLP model or service and start using it for document clarification, named entity recognition, sentiment analysis, and building a knowledge graph. These were the top four NLP use cases as per the survey.
Cloud-based NLP services also grow along with NLP technologies and applications. Conducted in July and August among 571 individuals from 50 countries, the survey reveals that 77% of respondents used at least one of the four leading cloud NLP services, and Google Cloud was the most popular.
The survey also shows that 64% of technical leaders were availing one or more cloud NLP service, and 65% of respondents who were in production with NLP were using one cloud service.
Although the survey indicates that the cloud is a hotbed of NLP activity, it comes with caveats. Respondents of the study flagged several concerns about using cloud NLP services like cost, customisation, accuracy, and security.
The top and foremost concern of cloud NLP is the cost. "Whenever you touch a document, parse a sentence or word, you're liable to pay. In other words, "if you're playing around, and exploring things may take you a while to figure out what you're doing," says Lorica.
The second concern is that cloud NLP services are somewhat generic. They typically are called via an API that delivers a result based on the text provided by the consumer, though s/he can't tune them. That restricts their usefulness for more advanced use cases.
Lorica cites, "If you wish to use NLP technologies for healthcare, it turns out they have different ways of talking. So, ER doctors may use other verbs than radiologists." She adds, "Everyone has a different level of tuning that they need to do, even for these advanced models, which you've been reading about. I don't know how you do that in the cloud as the cloud NLP models might not be easy to tune for you."
The good news is that original NLP practitioners have a slew of tunable open-source NLP models to pick from. The most popular NLP library was Spark NLP, followed by spaCy, Allen NLP, nltk, Hugging Face, Gensim, and Standford CoreNLP.
Lorica sees the potential for organisations with the state of NLP today to get started with cloud NLP services to see how text processing and analysis can be used and probably switch to run their own NLP models either on premise or on cloud-based IaaS when they touch the tuning or cost.
Join our WhatsApp Channel to get the latest news, exclusives and videos on WhatsApp
_____________
Disclaimer: Analytics Insight does not provide financial advice or guidance on cryptocurrencies and stocks. Also note that the cryptocurrencies mentioned/listed on the website could potentially be scams, i.e. designed to induce you to invest financial resources that may be lost forever and not be recoverable once investments are made. This article is provided for informational purposes and does not constitute investment advice. You are responsible for conducting your own research (DYOR) before making any investments. Read more here.