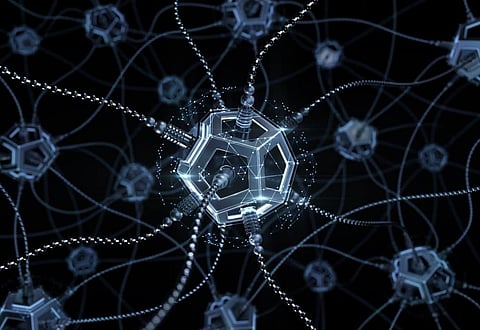
Reinforcement Learning (RL) is a machine learning method that empowers a specialist to learn in an intuitive environment by performing trial and error utilizing observations from its very own activities and encounters. In spite of the fact that both direct and reinforcement learning use mapping among input and output, not at all like supervised learning where input gave to the specialist is basically the right set of activities for playing out a task, reinforcement learning utilizes prizes and discipline as signs for positive and negative conduct.
When compared with unsupervised learning, reinforcement learning is distinctive as far as objectives are taken into consideration. While the objective in unsupervised learning is to discover synonymities and contrasts between data points, in reinforcement learning the objective is to locate a reasonable activity model that would boost the aggregate total reward of the specialist.
Reinforcement learning will be a huge thing in Data science in 2019. While RL has been around for quite a while in the scholarly world, it has barely observed any industry adoption whatsoever. Halfway on the grounds that there have been a lot of low-draping organic products to pick in predictive analytics, yet for the most part as a result of the hindrances in execution, learning and accessible tools. The potential worth of utilizing RL in proactive analytics and AI is huge, yet it additionally demands a more noteworthy range of abilities to ace. In addition to the fact that it involves more complicated algorithms and less advanced tools, it likewise requires precise recreations of real-life conditions. An expanding number of individuals in the industry think about the boundless capabilities of RL, however not many have been willing to make real investments. It's, for the most part, considered excessively uncertain.
Reinforcement learning is the way DeepMind developed the AlphaGo framework that beat a high-positioning Go player and has of the late been winning online Go matches namelessly. It's the means by which University of California Berkeley's BRETT robot figures out how to move its hands and arms to perform physical undertakings like stacking squares or screwing the top onto a container, in only three hours or even in ten minutes if it's told where the objects are kept that it will work with, and where they have to wind up. Engineers at a hackathon assembled a smart trash that can also be considered as AutoTrash which deployed reinforcement learning for sorting compostable and recyclable waste into the correct compartments. Reinforcement learning is the reason Microsoft just purchased Maluuba, which the company intends to utilize it to help in understanding day-to-day language for inquiry and chatbots, as a springboard to general intelligence.
However, business deployments are far rarer. In 2016, Google began utilizing DeepMind's reinforcement learning to understand how to spare power in some of its data centers by figuring out how to enhance around 120 unique settings like how the fans and cooling frameworks run, signifying a 15% enhancement in power utilization proficiency. Further, hardly people noticed, back in January 2016, Microsoft begun utilizing a very particular subset of reinforcement learning called contextual bandits to pick up customized features for MSN.com; something various machine learning frameworks had not been able to do. The contextual bandit framework expanded clickthrough by 25% and a couple of months after the fact, Microsoft transformed it into an open source Multiworld Testing Decision Service based on the Vowpal Wabbit machine learning framework, that you can keep running on Azure.
In contrast to contextual bandits, there isn't only one procedure for reinforcement learning. There isn't an institutionalized stage like the Multiworld Decision Service that you can use on your own issues. But in a lot of places, stages are being developed which can be used by researchers to carry out their experiments.
When we talk so much about AI, reinforcement learning isn't new; the principal reading material covering it dates to 1998. What's new currently, is that we have involvement with a few issues that are surely known, especially in the two territories of contextual bandits and imitation learning. In any case, we additionally require these new experimentation stages like Universe, Project Malmo and DeepMind Lab to give more scientists certain access and to assess solutions in a similar situation with benchmark progress.
A static dataset isn't valuable for assessing more general reinforcement learning. Two unique operators will take two distinct directions through an environment. Rather, analysts require an expansive, different set of conditions that is likewise institutionalized so everybody in the field neutralizes them. Adaptable, diverse stages can serve the similar capacity as a depository for reinforcement learning tasks where we can assess and emphasize on thoughts coming out of research a lot quicker than before when we needed to confine the algorithms to easy assessment problems since more perplexing ones weren't accessible. In the current scenario, we can take ideas to the stages and see regardless of whether they work to perfection.
In reality, each individual has distinctive knowledge, abilities and wants. It's vital over the long haul to see how an AI professional might find out about those objectives and about the quirks and capabilities of the individual it's working with and have the capacity to customize its assistance and activities to enable that specific individual to accomplish their objectives.
Join our WhatsApp Channel to get the latest news, exclusives and videos on WhatsApp
_____________
Disclaimer: Analytics Insight does not provide financial advice or guidance on cryptocurrencies and stocks. Also note that the cryptocurrencies mentioned/listed on the website could potentially be scams, i.e. designed to induce you to invest financial resources that may be lost forever and not be recoverable once investments are made. This article is provided for informational purposes and does not constitute investment advice. You are responsible for conducting your own research (DYOR) before making any investments. Read more here.