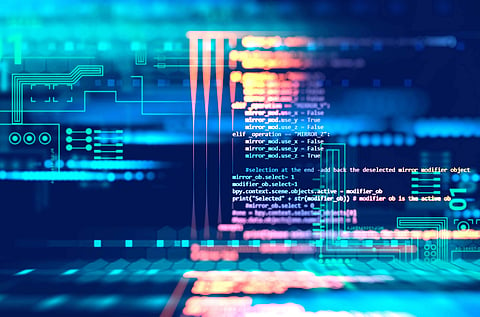
ModelOps (model operations) generally refers to the development of an analytics model that moves from the lab to IT production. It is a DevOps variation and follows DevOps principles to ensure IT compliance, security and manageability. As DevOps is designed to focus on application development, ModelOps focuses on analytics. This is vital for predictive analytics, allowing the continuous delivery, and efficient development and deployment of models.
While a large number of organizations are relying on machine learning models to derive fresh insights and information from voluminous data, SAS noted, these machine learning models are not limited by the number of data dimensions they can effectively access and use vast amounts of unstructured data to identify patterns for predictive purposes. But model development and deployment are not an easy task. Only about 50 percent of models are ever put in production and those that are taken at least three months to be ready for deployment. This time and effort equal a real operational cost as well as mean a slower time to value.
According to Stu Bailey, Co-Founder and Chief AI Architect of ModelOp, ModelOps is a capability that focuses on getting models into 24/7 production. It's a capability that must be owned by the CIO's organization or the technology center of a large organization.
Since the businesses' adoption of Artificial Intelligence and Machine Learning is increasing rapidly, analytical assets and models are also gaining momentum. Although most organizations concede the growing significance of a data-driven culture in their enduring digital transformation, implementing changes based on that data is challenging. Meanwhile, model development these days is becoming more ubiquitous for solving business problems. In fact, as per IDC estimations, only 35 percent of organizations have fully deployed analytical models in production.
ModelOps describes how analytical models are cycled from the data science team to the IT production team at a regular frequency of deployment and updates. The ModelOps team assists in fuelling communication between data scientists, data engineers, application owners and infrastructure owners and coordinates proper handoffs and execution so that models can advance to the so-called last mile. ModelOps responsibilities include workflow automation, version control, promotions, compute resource management, monitoring and scaling and tuning, among others.
Moreover, ModelOps focuses on expediting dynamic collaboration and improved productivity for analytics and IT operations teams, regardless of which analytic language is used, which data is accessed, or where the model will be deployed. In order to bring analytics from the lab into use, a ModelOps approach includes accessing data from a trusted source and maintaining privacy and security standards; Avoiding rework by keeping a deployment scenario in mind when creating models; Retaining data lineage and track-back information for governance and audit compliance; Accomplishing ModelOps rapidly while ensuring close collaboration between data scientists and IT; and Focusing on monitoring when deploying models so that analysts can monitor and retrain models as they degrade.
Without ModelOps capability, there's no owner on the operational side or appropriate role for operationalizing models in the 24/7 framework, Stu Bailey said. On the other side, no one model or method comes without challenges. As models can degrade as soon as they are deployed, SAS noted, of course, some things will affect the performance of your models more than others, including data quality, time to deployment, and degradation.
Join our WhatsApp Channel to get the latest news, exclusives and videos on WhatsApp
_____________
Disclaimer: Analytics Insight does not provide financial advice or guidance. Also note that the cryptocurrencies mentioned/listed on the website could potentially be scams, i.e. designed to induce you to invest financial resources that may be lost forever and not be recoverable once investments are made. You are responsible for conducting your own research (DYOR) before making any investments. Read more here.