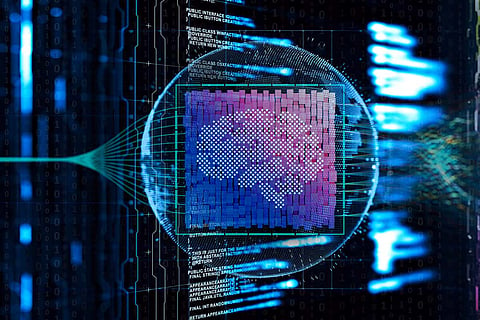
An enterprise consists of large, complex and process-centric systems including think finance, Robo-advisors, accounting, operations, human resources, and procurement which use and produce a hefty amount of structured and unstructured data. There is a need to handle these in new ways to save time and money.
ML blended with solution-specific software can improve the speed, accuracy, and effectiveness of back-office operations of such enterprise and help them revamp their workflow efficiently.
As such, the shift toward AI, and specifically ML, is to improve the process, not just speed. Here are five specific applications of ML that can be used to improve back-office operations:
Account reconciliations is one of the painful and error-prone operations in an enterprise. As they are critical to every business, it is necessary to ensure the proper controls are in place to close the books accurately and on-time. Here ML is an ideal approach for account reconciliations, specifically matching reconciliations. But the challenge is for large and complex data sets, the combinatorial problem of matching is really hard. However, some companies have focused on this problem and solved it with a combination of machine learning and point-solution software as a hosted platform.
Every business at some point deals with invoices. With the advancements of NLP and ML, there are more ways to improve this task at the enterprise level. According to some companies well-implemented accounts payable systems can reduce time and costs by 75 percent, decrease error rates by 85 percent, and improve process efficiency by 300 percent. Also, these companies are extending their NLP and ML capabilities to reduce the burden of invoice management by automatic capturing of information from invoices and triggering the appropriate workflow.
One of the interesting use cases of ML−NLP is the detection of employee attrition. Apart from hiring and retaining, keeping the employees happy implies towards sustainable growth. To detect the attrition risk data source is required. Using the data such as tenure, time since last pay raise/promotion, sick days used, scores on performance reviews, skill set competitiveness with the market, and generally available employment market data, companies can assess and analyze the probability of an employee to leave the office space.
The use of sensors into a variety of equipment including trucks, oil rigs, assembly lines, and trains means the generation of massive data on usage, wear and tear of such equipment. Pairing such data with historical records to know when certain types of equipment need repairing means expensive machinery can be scheduled for downtime and repair based on the number of hours used or a number of miles driven and what the actual usage is.
The companies that spend a lot of money on hard goods for them stock in transit is a key source of opportunity for applying ML to predict the arrival of goods at the destination. With the use of sensors item tracking has improved a lot. Using the data regarding weather, traffic, type of transport, risk probabilities, and historical performance companies can optimize the process timing of the flow of goods.
Join our WhatsApp Channel to get the latest news, exclusives and videos on WhatsApp
_____________
Disclaimer: Analytics Insight does not provide financial advice or guidance. Also note that the cryptocurrencies mentioned/listed on the website could potentially be scams, i.e. designed to induce you to invest financial resources that may be lost forever and not be recoverable once investments are made. You are responsible for conducting your own research (DYOR) before making any investments. Read more here.