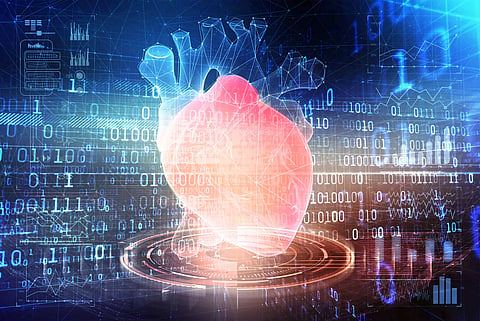
A team from MIT's Computer Science and AI Laboratory introduced a new system for better health prediction. The system is based on a machine learning model, capable of estimating patient's risk of cardiovascular death, from the electrical activity of his/her heart. The system has been termed as RiskCardio which focuses on patients who have survived an acute coronary syndrome (ACS). The ACS refers to a range of medical condition where there's a reduction or blockage of blood to the heart. The tool produces score using just the first 15 minutes of patient's raw ECG signals and then put him into different risk categories according to it.
The patients tagged in the top quartile with high risk by RiskCardio were nearly 7 times more likely to die due to cardiovascular death as compared to other quartiles.
Divya Shanmugam, lead author on a new paper about RiskCardio said, "We're looking at the data problem of how we can incorporate very long time series into risk scores, and the clinical problem of how we can help doctors identify patients at high risk after an acute coronary event. The intersection of machine learning and healthcare is replete with combinations like this — a compelling computer science problem with potential real-world impact."
Previous ML models used domain-specific knowledge like – external patient information like age or weight, or using knowledge and expertise specific to the system while RiskCardio uses just the patients' raw ECG signal, with no additional information.
Usually, when a patient visits a hospital seeking treatment for ACS, it takes a long time as at first physician would estimate the risk of cardiovascular death or heart attack using medical data and lengthy tests followed by a course of treatment. However, RiskCardio has goals to improve that first step of estimating risk. In order to simplify the initial step, the system separates a patient's signal into sets of consecutive beats, with the idea that variability between adjacent beats is indicating downstream risk. Notably, the system has been developed and trained using data from the study of past patients.
While training the model, the team initially separated each patient's signals into the collection of adjacent heartbeats and then assigned them a label – if the patient died of cardiovascular death or not – to every heartbeats sets. The researchers trained the model to label patients as 'risky' or 'normal' as per the outcomes.
In the case of a new patient, the researchers' team generated a risk score by averaging the patient's forecast from each set of adjacent heartbeats. Interestingly, the first 15 minutes of a patient's ECG signals are enough to estimate if he will die due to cardiovascular risk within 30, 60, 90 or 365 days.
However, simple it may seem but to calculate risk score from ECG signals is not an easy task. In fact, the signals generated are very long and with the increase in the number of inputs, to interpret the relationship between them becomes tougher.
The MIT researchers hope to create a dataset inclusive to account for varied ages, ethnicities, and genders. Additionally, they plan to examine medical circumstances where there is a pool of poorly labeled or unlabelled data and evaluate how the ML system processes and handles such information to accounting for obscure cases.
Shanmugam further said, "Machine learning is particularly good at identifying patterns, which is deeply relevant to assessing patient risk. Risk scores are useful for communicating patient state, which is valuable in making efficient care decisions."
Join our WhatsApp Channel to get the latest news, exclusives and videos on WhatsApp
_____________
Disclaimer: Analytics Insight does not provide financial advice or guidance. Also note that the cryptocurrencies mentioned/listed on the website could potentially be scams, i.e. designed to induce you to invest financial resources that may be lost forever and not be recoverable once investments are made. You are responsible for conducting your own research (DYOR) before making any investments. Read more here.