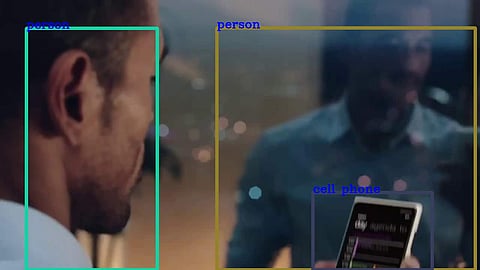
As it stands now, computer vision algorithms are being used in the analysis of medical images, enabling self-driving cars, and powering face recognition. However, training models to recognize actions in videos have become extremely expensive and it has also raised concerns about the technology's carbon footprint and its inaccessibility in low-resource environments.
Considering visual recognition as the strongest skill of deep learning, researchers have shrunk state-of-the-art computer vision models to work on low-power devices. Researchers at the MIT-IBM Watson AI Lab have developed a new technique to train video recognition models on a phone or certain other devices that have quite limited processing capacity. Conventionally, such algorithms used to process video by splitting it up into image frames and leveraging recognition algorithms on each of them. Further, it pieces together the actions shown in the video by analyzing how the objects change over subsequent frames. This method necessarily requires the algorithm to "remember" what it has seen in each frame and its order as well. The method is unnecessarily inefficient.
However, in the new approach, the algorithms extract basic sketches of the objects in each frame and surface them on top of one another. Instead of remembering what happened when the algorithm can get an impression of the passing of time by analyzing how the objects shift through space in the sketches.
Song Han, an assistant professor at MIT's Department of Electrical Engineering and Computer Science (EECS) said – "Our goal is to make AI accessible to anyone with a low-power device. To do that, we need to design efficient AI models that use less energy and can run smoothly on edge devices, where so much of AI is moving."
While testing the model, researchers discovered that the new approach trained video recognition models three times faster than the state of the art. The new model is also efficient in quickly classifying hand gestures with a small computer and camera running on limited energy devices.
This new approach could be helpful in reducing lag and computation costs in existing commercial applications of computer vision. In the autonomous automobile industry, for example, it can make self-driving cars safer by speeding up their reaction to incoming visual information. The technique could also unlock new applications that previously were impossible. The technology can enable phones to help diagnose patients or analyze medical images.
Dario Gil, director of IBM Research quoted – "Compute requirements for large AI training jobs are doubling every 3.5 months. Our ability to continue pushing the limits of the technology will depend on strategies like this that match hyper-efficient algorithms with powerful machines."
With new advancements and as more and more AI research gets translated into applications, it is predicted that the need for smaller models will increase. Notably, the MIT-IBM research paper is part of a growing trend to shrink state-of-the-art models to a more manageable size.
Join our WhatsApp Channel to get the latest news, exclusives and videos on WhatsApp
_____________
Disclaimer: Analytics Insight does not provide financial advice or guidance. Also note that the cryptocurrencies mentioned/listed on the website could potentially be scams, i.e. designed to induce you to invest financial resources that may be lost forever and not be recoverable once investments are made. You are responsible for conducting your own research (DYOR) before making any investments. Read more here.