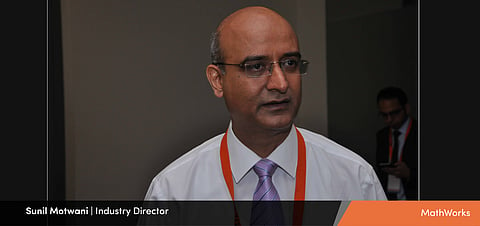
MathWorks is the leading developer of mathematical computing software MATLAB and Simulink. MATLAB, the language of technical computing, is a programming environment for algorithm development, data analysis, visualization, and numeric computation. Simulink is a graphical environment for simulation and Model-Based Design for multidomain dynamic and embedded systems.
Engineers and scientists worldwide rely on these product families to accelerate the pace of discovery, innovation, and development in automotive, aerospace, electronics, financial services, biotech-pharmaceutical, and other industries. MATLAB and Simulink are also fundamental teaching and research tools used in global universities and learning institutions. Founded in 1984, MathWorks employs more than 3500 people in 15 countries, with headquarters in Natick, Massachusetts, USA.
Engineering and IT teams are using MATLAB to build today's advanced big data analytics, artificial intelligence, cloud computing, IoT, and Robotics systems. MATLAB provides a wide variety of ways to access data from various sources, including business data from databases, data warehouses, and Hadoop, to engineering data from sensors, data historians, and industry-specific protocols.
MATLAB Integrates Workflows
Major engineering and scientific challenges require broad coordination across teams to take ideas to implementation. Every handoff along the way adds errors and delays. MATLAB helps automate the entire path from research to production.
MATLAB Is Trusted
Engineers and scientists trust MATLAB to send a spacecraft to Pluto, match transplant patients with organ donors, or just compile a report for management. This trust is built on impeccable numerics stemming from the strong roots of MATLAB in the numerical analysis research community. A team of MathWorks engineers continuously verifies quality by running millions of tests on the MATLAB code base every day.
MATLAB Is Designed for Engineers and Scientists
Everything about MATLAB is designed specifically for engineers and scientists:
• Function names and signatures are familiar and memorable.
• The desktop environment is tuned for iterative engineering and scientific workflows.
• Documentation is written for engineers and scientists, not computer scientists.
Sunil Motwani is the Industry Director at MathWorks India office and manages sales for commercial customers in the country. He has been at MathWorks since 2008 from the time it started operations in India. Prior to joining MathWorks, Sunil worked at Hewlett Packard & Agilent Technologies managing sales of test instruments for various industry segments in India including Aerospace & Defense, Communications, Semiconductor, Automotive and Industrial Automation.
Sunil has over 25 years of experience in sales of technology products across various regions within India having been based at Mumbai, Delhi, Hyderabad & Bangalore during this time. He holds a Bachelor's Degree in Electronics Engineering from Visvesvaraya National Institute of Technology (VNIT), Nagpur and a Post-Graduate Diploma in Software Technology from National Centre of Software Technology (NCST), Mumbai.
Sunil has been responsible for building market expansion strategies, partnerships, domain expertise and engineering capabilities across the MathWorks' operations in India. He sees a huge potential emerging in the market for engineering data analytics that drives applications like predictive/prescriptive analytics, fleet analytics, autonomous systems (automated driving, UAVs, Robotics), IoT etc. "Data science platforms make it possible for the engineering teams that develop and maintain the equipment to leverage their wealth of knowledge about how the equipment should operate. This idea of empowering the engineers, or domain expert, is often more appealing than hiring data scientists who have little knowledge of how the equipment operates", he says.
Sunil feels the significant challenge for the company has been helping customers get data from the source into the hands of end users, which is a common barrier for engineers who need data to formulate requirements for new products, troubleshoot field problems, and come up with new technologies. With more and more streaming data, the industry is faced with a data science challenge. We need to ensure that the speed of data analysis is keeping pace with data intake and, equally important, provide the capability to zoom into and extract insight from stored data throughout the engineering community.
To address this new challenge, one often looks for those who have computer science skills, knowledge of statistics, and domain expertise relevant to their specific engineering problems. However, domain expertise is often overlooked, yet it is essential for making judgment calls during the development of an analytic model. "Instead of searching for elusive data scientists, we're now working with engineering teams by helping their engineers to do data science with a flexible tool environment like MATLAB, which enables engineers to become data scientists," he added.
Sunil foresees there are three key trends to track when it comes to growth in big data analytics, AI, machine learning and deep learning.
Impacts of predictive analytics systems on industries like manufacturing and medical devices
• It's well-known at this point that data analytics technologies can bring significant business benefits in areas such as predictive maintenance. However, the system architecture for such applications remains an open question. Customers are hesitant to share their data with vendors, logging all of the data from a machine is often impossible given the volume of data created, and responses to events may be needed in milliseconds – much too short of a time to wait for a response from an Internet server. All of these will drive innovation at "the edge", or on the equipment itself.
• Medical Devices: Predictive analytics systems will allow for more informed and personal relationships between patients and physicians and more effective diagnoses at point-of-care. It is quite possible that predictive analytics will also drive the progress of both preventative and therapeutic care with the data collected from wearables and shared on personal devices.
• As it becomes easier and easier to apply machine learning techniques, more products and services will incorporate machine learning models. Embedded systems, typically used for controls and diagnostics, will incorporate machine learning models that can detect previously unobservable phenomena. In 2018, we'll continue to see machine learning models being incorporated in new places, especially in edge nodes and embedded processors.
• While deep learning continues to look promising, there is still a lot of design and tuning necessary to train a useful deep network. Techniques such as automated hyperparameter tuning appear well-positioned to reduce this work, which should ramp-up the pace of adoption of deep learning.
Domain Experts Take on Data Science
• As engineering and IT teams become more integrated, there will be an increased demand for domain experts that understand the core products and services of the business. Empowering domain experts to apply data science methodologies will enable the rapid integration of big data and machine learning technologies into the services and operations of the broader organization, establishing a significant competitive advantage when offering the products and services that customers are demanding.
More importantly, the ability to create analytics that processes massive amounts of business and engineering data enables designers in many industries to develop intelligent products and services. MathWorks foresees engineers will be challenged to build confidence in using new methods and tools to overcome an ever-increasing amount of data and the growing complexity of software. While teams working on disruptive technologies can successfully mine data to build intelligent systems, a select few are beginning to see the advantages of adopting workflows derived from a common software environment that incorporates data science tools and techniques with physics-based simulation models.
Join our WhatsApp Channel to get the latest news, exclusives and videos on WhatsApp
_____________
Disclaimer: Analytics Insight does not provide financial advice or guidance. Also note that the cryptocurrencies mentioned/listed on the website could potentially be scams, i.e. designed to induce you to invest financial resources that may be lost forever and not be recoverable once investments are made. You are responsible for conducting your own research (DYOR) before making any investments. Read more here.