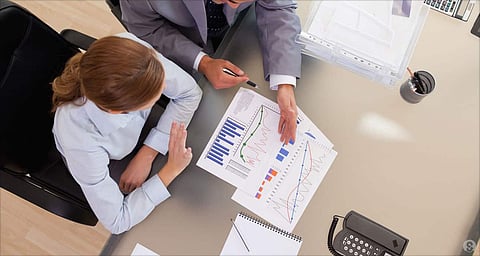
Humans are good at correlating things in almost every aspect of their lives. It comes by nature and is completely inevitable. We make decisions in life mostly based on correlations. However, the strength of association and what one imagines is not always true or what we perceive. In analytical terms, the Pearson's correlation coefficient (r) could be positive, zero or negative. That means, there may be a strong relationship between two variables, may not have any association between things or may be completely negative.
In analytics, a data scientist should not make judgments based on pure pre-conceived notions. It has to be completely laden with hypothesis testing. At the same time, it should not be without logic. Let's see an example. Mr. X died at the age of 99. He drank whiskey all his life. Mr. Y died at the age of 96. He did yoga all his life. So, what does this conclude? Does whiskey give a three-year edge over yoga? Not necessarily.
Let's take one more example. As ice cream sales increase, the rate of drowning deaths increases sharply. So, ice cream causes drowning. Maybe. However, in this example, there is one more factor that is linked to both the variables which is "time". During the summer months, people are more likely to engage in activities involving water, such as swimming. The increased drowning deaths are simply caused by more exposure to water-based activities, not ice cream. There are numerous examples where there is actually no correlation. We fail to identify the same and it eclipses the outcome. As a result, we get incorrect results.
In today's data-driven world, we are so busy in arriving at the conclusion that we underestimate the power of logic and domain knowledge. We use different tools and feel that they would answer all our questions without even giving a thought to the results. Of course, tools and techniques will give you different ways to get the outcome. But the heart of strategic decision making is the knowledge about the subject and the logic, and both are hard to beat.
Below is an example which would help us understand the importance of logic. Let's try to find an outlier from the blood pressure readings of different patients and categorize them in Acceptable, Low, High and Error: 120/90, 150/110, 40/25, 50/30, 50/35, 50/30,70/50, 90/60, 180/120. Here, we would jump to assign the figures with high, acceptable and low values. But when it comes to finding the error, we may scratch our head in confusion. Probably we would say the data has no errors. But if you look at it carefully, the figure 40/25 is an outlier. And practically, if the blood pressure of the patient gave this reading, the patient is dead by now.
The success in analytics involves much more than statistics and algorithms. A model would crunch many rows and columns of data and predict a response based on different algorithms. But what if the data does not make any sense. In order to check the quality of the prediction, one requires a good knowledge of the industry. A data scientist should ask as many questions as possible to satisfy his desire for the results. And when we delve deeply into the questions, we understand the power of domain knowledge. A successful data science project must connect data and results with domain expertise to uncover hidden insights and drive excellent results.
Join our WhatsApp Channel to get the latest news, exclusives and videos on WhatsApp
_____________
Disclaimer: Analytics Insight does not provide financial advice or guidance. Also note that the cryptocurrencies mentioned/listed on the website could potentially be scams, i.e. designed to induce you to invest financial resources that may be lost forever and not be recoverable once investments are made. You are responsible for conducting your own research (DYOR) before making any investments. Read more here.