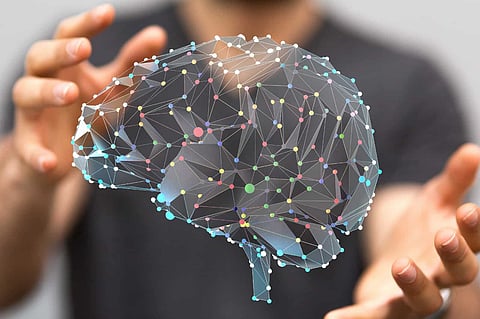
For years, science fiction movies have portrayed technological marvels that involved artificial intelligence (AI) and automation. Now that it is part of our lives, organizations need to understand that it's not all about fancy algorithms and models, it's about making it easier to use.
AI, particularly machine learning can drive tangible business value for a broad spectrum of industries. Despite the momentous volume of data available, organizations are still struggling to deploy these technologies to solve real business problems. Simply put, the challenge for most organizations isn't that machine learning doesn't work, but that they actually struggle to use it.
Like other new technologies, AI and machine learning hold a lot of unrealistic expectations and limitations. In this article, we will discuss the same to help organizations better understand what may be holding back their efforts. Along the way, we will also highlight solutions to overcome these limitations and create a new wave of opportunities for organizations.
Technology service providers work on multiple software projects. A common hurdle they often face is understanding the root cause of errors in different models. Ironically, for most companies, the problem is not the algorithm, it's the data which is being used in the model. If you have access to better data than the competition, then you can surely build a better product than them.
As organizations try to build machine learning models, they get surprised to learn that the most hyped big data tools — deep learning, AutoML, etc. are still not sufficient to make it work. Instead, they realize that embedding machine learning in products and processes represents a fundamentally different approach. An approach which allows domain experts to come in at the right level and use tools to support effective model testing.
Machine learning models do not have a tendency to relate their experience from one use case to another, unlike humans. This means, whatever a model has achieved for a use case remains applicable to it only. As a result, companies fail to develop a generalized structure that can be applied to multiple problems of similar use cases.
Machine learning is going through a paradigm shift from the current practice of creating models in recent years. The progress in machine learning has accelerated rapidly as a new approach based on "deep learning" has been adopted. Machine vision, speech recognition, and natural language processing are some of the breakthrough applications powered by deep learning algorithms.
As technology advances, understanding the growth of machine learning capabilities becomes critical for organizations. So, how can organizations narrow the new technological gap? Let's explore the four most important aspects to consider while building products using the next generation of machine learning systems.
Process Improvement: An efficient data insight process increases agility and delivers great customer experience. Organizations can make better use of human efforts by creating models in various stages from raw data representation to final deployment. Different resources could be involved in this process to develop a fool-proof concept and deploy the necessary models.
Involving Domain Experts: Domain knowledge is the foundation of any data analytics work. Domain experts determine key variables in the model, ask right questions and direct the analysis towards business objectives. This ultimately ensures that the models developed by data scientists and engineers generate maximum business value.
Recognizing Patterns: A crucial part of the machine learning process involves extracting patterns from raw data. Understanding hidden patterns in data lead to a better way of expressing a solution to complex problems. In other words, pattern recognition can be a powerful engine for producing actionable results.
Testing Models Intelligently: Software testing is one of the most critical factors that determine the success of a machine learning system. Project managers need to put their models through different rounds of validation and testing before deploying them. It offers them the advantage to simulate previous states of the data, add missing data and perform their own test suites for several points in time.
Machine learning has begun to deliver on the potential created by data-driven organizations. In order to extract more value from it, organizations must become agile and focus not just on technology, but on the process. They need to bridge the gap between cutting-edge technologies and the ability to actually implement models to solve real-world problems.
Machine learning is poised to have a transformational impact on organizations as a benchmark for doing business. Organizations which rapidly anticipate and respond to opportunities will seize the advantage in the AI and machine learning-enabled landscape. If you still think your organization can let the technology progress and then be a successful fast follower, think again!
Join our WhatsApp Channel to get the latest news, exclusives and videos on WhatsApp
_____________
Disclaimer: Analytics Insight does not provide financial advice or guidance. Also note that the cryptocurrencies mentioned/listed on the website could potentially be scams, i.e. designed to induce you to invest financial resources that may be lost forever and not be recoverable once investments are made. You are responsible for conducting your own research (DYOR) before making any investments. Read more here.