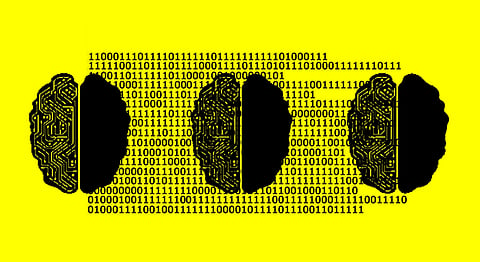
Gartner predicts IoT will connect 20 billion devices by next year, and enterprise's increased adoption of cloud computing, which requires high bandwidth and low latency to connect to applications and data. Eighty-three percent of enterprise workloads will be in the cloud—also by next year—according to a recent study.
Service providers' traditional approach to network management—their present mode of operations—is not particularly well-equipped to manage this explosive growth of on-demand services. Many still rely on static methods to provision bandwidth, which lack the scale and flexibility to meet the requirements of these more dynamic services, resulting in poor Quality of Experience (QoE) for customers. As noted by a report, this is frequently compounded by a manual and siloed approach to both short and long-term network capacity planning, which often leads to overprovisioning the network to manage peak demand, along with high operational costs and wasted resources. In addition, by not taking advantage of real-time analytics and automation, service providers must wait for their network to break down and then take manual corrective action, which further degrades QoE.
And while subscribers continually look for improved services from their service providers, it is not usually matched with a willingness to pay more. As a result, service providers must continually lower cost structures, reduce operating expenses, accelerate time to revenue, and replace older services with compelling new ones—all at unprecedented speeds.
To manage these multiple demands and pressures, service providers must move beyond traditional methods of network and service design and management. Technologies like Software-Defined Networking (SDN) are enabling great strides in meeting some of the challenges; and now with big data analytics and Artificial Intelligence (AI), service providers can make true intelligent automation possible, run their businesses smarter and much more efficiently, and adapt at a pace in line with customer expectations.
The good news is the network itself holds enough information and data that, when collected, analyzed, and acted upon properly, can help service providers proactively plan, strategize, and better execute on their business goals.
Of course, using analytics isn't new for service providers, who have been using it for years to help improve business outcomes. A simple example is using analytics to monitor traffic to identify peak demand, and then manually configure the network for higher capacity during those busy hours. As more devices and more traffic are added to the network, however, humans processing all this data becomes impossible.
Advanced analytics must go beyond simply analyzing patterns and trends and taking reactive actions to enable proactive operations. It must incorporate:
• AI, the ability for the network to perform tasks normally performed by humans that involve perception, understanding, and decision-making
• Machine Learning (ML), a form of AI that develops applications that use statistical techniques to enable systems to 'self-learn' and progressively improve performance on a specific task—to be able to think like a human but at superhuman speed, scale, and accuracy, and then take action
To make sense of the data, analytics typically fall into one of five categories:
Descriptive, which interprets historical data to uncover and understand what has happened in the past
Diagnostic, which uses techniques such as data mining, discovery, and correlation for a root-cause analysis to determine why something has happened
Predictive, which uses historical patterns to predict what might happen in the future
Prescriptive, which shows how different actions will affect the current situation with the goal of providing the best possible option
Adaptive, which leverages prescriptive analytics to determine the best course of action, and then uses an actionable system to execute on it
The depth of automation and the level of AI sophistication increase by progressing from descriptive to adaptive, as does the corresponding value. The actual functional flow from collecting data to making adaptive changes to the network involves multiple steps that include the various analytics methods described. It also requires policy-based action and a reinforcement loop. Using data collected from multiple sources, the analytics system runs a ML algorithm to identify patterns and trends, which are used to generate an ML model. As new data comes into the system, the analytics application uses the ML model to assess what's likely to happen and drives what action to take by interacting with policy systems to make changes on the network. Policy can drive the adaptive process by updating the ML model based on a reward function. The best outcomes are achieved through multiple iterations of the process.
By adopting AI and ML, service providers will be able to analyze higher volumes of more complex data and use the information to deliver faster, more accurate results. The network can be trained to look for patterns that indicate congestion, port failure, or other problems about to occur and generate actionable information across multiple network domains. This will help service providers better manage their network resources to meet current and future demand, predict disruptions before they happen, and take steps to reconfigure or re-provision the network before services are impacted, creating self-optimizing networks.
Taking advantage of AI and ML technologies is only one component of creating a self-optimizing network. For service providers to fully reap the benefits, they must look at the whole solution requirement. This means not merely looking at what specific functions are required to achieve the end-to-end analytics process, as described in the earlier section, but also how these functions are implemented to enable the best possible outcomes.
One of the biggest challenges of using network data intelligently is the nature of that information, which falls squarely into the big data category. Big data is best described in terms of 'multiple Vs', where each V identifies a specific property or characteristic of data. While traditional analytics platforms were generally measured on how well they meet the original three Vs of big data—Volume, Variety, and Velocity—advanced network analytics for intelligent automation provides emphasis on two more: Value and Visualization.
Volume refers to the amount of data that must be handled, which is many orders of magnitude larger than traditional datasets. Over a million devices connect to the network every hour, for example, requiring the network to transport a wide range of services and applications. This number is continually growing, which means planning is required to support even higher volumes of data in the future.
Variety refers to the different types of data that must be collected and analyzed. Today's networks consist of many layers, technology domains, network elements, and systems from many different vendors. Think location data (for mobile users, for example) compared to OSS billing data or network telemetry. It can be very different depending on where it originates, and up to 80 percent of it is unstructured. This means it will not fit neatly into fields on a spreadsheet or in a database as with the structured data of the past.
Velocity refers to the speed with which data is collected, added to the dataset, and analyzed. Traffic constantly traverses the network, which means there is continuously new information to be collected and extracted. For a self-driving network, having the ability to collect, process, and store data, as well as enabling it to be retrieved in real time or near real time, is crucial for deriving meaningful insight and opportunities—ones that may only exist for a brief time.
Value refers to the ability to derive business value from the data. This means accurately translating heterogeneous information from multiple layers and domains of the network into insights that can then be used to drive actions that help achieve specific business goals and generate measurable improvements.
Visualization refers to the ability to accurately communicate the results of data analysis to allow users to quickly assess its value. This is crucial for enabling optimal decisions and actions, and to ensure operators maintain 'control' of the automation programmed into a continuously self-configuring and self-optimizing network. It should be interactive and customizable and easy for varying audiences involved in business operations to understand, so they can collectively drive business decisions.
ML and big data analytics that fully deliver on the multiple Vs can help service providers address a wide range of business and operational initiatives. For example, predictive analytics based on ML can enhance network assurance. Analytics can learn from historical performance metrics and trouble ticketing data, and then combine that knowledge with real-time network telemetry to anticipate future network failures to create a self-healing network. Analytics can also be used to optimize traffic management and capacity planning.
Join our WhatsApp Channel to get the latest news, exclusives and videos on WhatsApp
_____________
Disclaimer: Analytics Insight does not provide financial advice or guidance. Also note that the cryptocurrencies mentioned/listed on the website could potentially be scams, i.e. designed to induce you to invest financial resources that may be lost forever and not be recoverable once investments are made. You are responsible for conducting your own research (DYOR) before making any investments. Read more here.