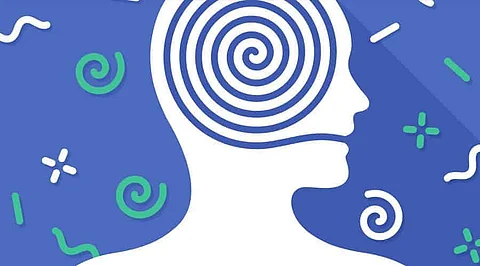
The AI-model will function as an early warning system for healthcare professionals regarding Alzheimer's disease.
The COVID 19 outbreak has highlighted the urgency in the early detection of diseases and drug discovery. Everyday technology is ramping up the pace of healthcare innovations. Diseases those were unable to get detected using a traditional approach, now have treatment due to technology. Undoubtedly, the past century has observed some great healthcare advancement through technology. However, despite new dawn in medical innovations, medical society has still grappling to early diagnose and treat certain progressive and neurodegenerative diseases.
Alzheimer's disease is a neurodegenerative and progressive disease that causes brain cells to degenerate and die. Being the common cause of dementia, it declines thinking, behavioral and social skills and disrupts a patients' ability to work independently. Reports suggest that an estimated 44 million people are living with Alzheimer's disease globally. In the US, an estimated 5.5 million people of all ages have Alzheimer's disease. The World Health Organization (WHO) has listed Alzheimer's disease to be the most common cause of Dementia in geriatric patients.
The early signs of Alzheimer's disease are the inability to remember recent events and acute memory impairments. Since stress ensues occasional memory lapses, the early detection of Alzheimer's disease is often misinterpreted and becomes challenging. The disease is only detected when the patients' situation worsens, thus affecting their ability to function independently.
Over the years' studies has been conducted for early detection of Alzheimer's disease. Many clinical trials involving analysis of the PET Scans or invasive procedure failed to provide a positive outcome for early detection of Alzehmiers' disease. Since the early detection of Alzheimer's has become a challenge, the drugs developed for the disease only decline the development of late symptoms.
However, a collaborative effort by IBM and Pfizer promises to be the potential breakthrough for earlier detection of Alzheimer's disease. The duo has created an AI-model that can detect Alzheimer's based on the linguist patterns of the patient.
The AI-model used Natural Language Processing to analyze one-to-two minute speech samples from a brief, clinically administered cognitive test. The language data which includes historical data of over three generations and 60 years' was provided by the Framingham Heart Study as the training dataset for the machine learning model. This data was quintessential to reliably detect the patterns over long periods. The study named "Linguistic Markers Predict Onset of Alzheimer's Disease" is published in the Lancet Journal of eClinical Medicine.
The current study differs from the previous studies as it involves the collection of samples when the subject was in a cognitive healthy state. Additionally, unlike the prior studies that focused on high-risk patients', this study focused to assess the disease in the general population, so as to achieve a broader aspect of the study. The researchers analyzed the transcriptions' of participants' language samples with Natural Language Processing, to tap subtleties and discourse that might have changed over time and could have possibly missed. The data was collected from the original participant's of the Framingham study, as well as their offspring and spouses to verify the model's prediction with real-life results.
The researchers analysed the verbosity, lexical richness, and repetitiveness by using metrics such as number of words, number of unique words, and frequencies of repetitions. The published paper cites analyzing misspellings, punctuation, and uppercasing to assess writing performance and style. Language-modeling analysis was performed to model the distributions of word sequences. Syntactic complexity was assessed through analysis of parse trees. Semantic content was assessed through analysis of participants' mention of information content units. Finally, propositional idea density analysis was used to quantify syntactic and semantic complexity.
The researchers believe that this model will function as an early warning system for healthcare professionals to do more extensive study. It must be noted that as the study is conducted in non-Hispanic white populations, the results might differ based on the socio-economic aspect. However, researchers promise 70% accuracy in predicting the early onset of Alzheimer's disease.