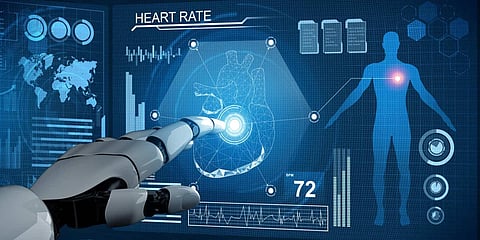
Every year, nearly one out of eight U.S deaths is caused due to heart failure. One of acute heart failure's most common causes is the presence of excess fluid in the lungs. This condition is known as 'pulmonary edema.'
A patient's excess fluid level often indicates the doctor's course of action, but such determination requires clinicians to rely on subtle features in X-rays that sometimes lead to inconsistent diagnoses and treatment plans.
A group of researchers at MIT's Computer Science and Artificial Intelligence Lab (CSAIL) has developed a machine learning model that can analyse the X-ray to quantify how severe the edema is, on a four-level scale ranging from 0 means healthy to 3 which is very bad. The system determined the level accurately, and correctly diagnosed level 3 cases 90% of the time.
Working with Beth Israel Deaconess Medical Centre (BIDMC) and Philips, MIT researchers now plan to incorporate the model into BIDMC's emergency-room workflow this fall.
Ruizhi Liao, PhD student, co-lead author of a related paper with fellow PhD student Geeticka Chauhan and MIT professors Polina Golland and Peter Szolovits, says, "This project is meant to augment doctors' workflow by providing additional information that can be used to inform their diagnoses as well as enable retrospective analyses."
The team claims that better edema diagnosis would help doctors manage not only acute heart issues but also other conditions such as sepsis and kidney failure that are strongly associated with edema.
Liao and his colleagues also took an existing public dataset of X-ray images and created new annotations of severity labels that were agreed upon by a team of four radiologists, as part of a separate journal article. These consensus labels may serve as a universal standard to benchmark future machine learning development.
A pivotal aspect of this system is that it was trained not only on more than 300,000 X-ray images but also on the corresponding text of reports about the X-rays that were published by radiologists. The team was astonished that their system achieved such success using these reports, most of which didn't have labels explaining the exact severity level of edema.
Tanveer Syeda-Mahmood, the chief scientist for IBM's Medical Sieve Radiology Grand Challenge, says, "By learning the association between images and their corresponding reports, the method has the potential for a new way of automatic report generation from the detection of image-driven findings."
Chauhan's efforts concentrated on helping the system make sense of the text of the reports that could often be as short as a sentence or two. Different radiologists write with different tones and use a range of terminology so that the researchers had to make a set of linguistic rules and alternatives to ensure that data could be analysed consistently across reports. It was in addition to the technical challenge of designing a model which can train both the image and text representations jointly in a meaningful manner.
"This model can turn both images and text into compact numerical abstractions from which an interpretation can be obtained," explains Mr Chauhan. "We trained it to distinguish between the representations of the X-ray images and the text of the radiology reports to improve the image interpretation."
He adds, "These correlations will be valuable for improving search through a large database of X-ray images and reports to make retrospective analysis even more effective."
The paper by Chauhan, Liao, Golland and Szolovits in collaboration with MIT Assistant Professor Jacob Andreas, Professor William Wells of Brigham and Women's Hospital, Xin Wang Philips, and Seth Berkowitz and Steven Horng of BIDMC was presented on October 5 at the International Conference on Medical Image Computing and Computer-Assisted Intervention (MICCAI).
MIT Despande Centre for Technological Innovation, the MIT Lincoln Lab, the National Institute of Health, Philips, Takeda, and the Wistron Corporation supported the work in part.
Join our WhatsApp Channel to get the latest news, exclusives and videos on WhatsApp
_____________
Disclaimer: Analytics Insight does not provide financial advice or guidance. Also note that the cryptocurrencies mentioned/listed on the website could potentially be scams, i.e. designed to induce you to invest financial resources that may be lost forever and not be recoverable once investments are made. You are responsible for conducting your own research (DYOR) before making any investments. Read more here.