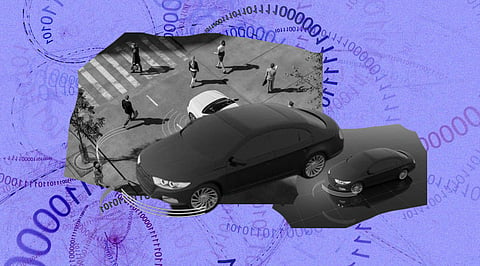
Although this finding would seem to have a lot to do with academia, it might have a big influence on how we now drive on the roads. The architecture underlying self-driving cars, which are now being developed by several major firms in the automotive and technology industries—Google, Volvo, Tesla, and Audi, to name a few—is based on a specific machine learning approach called deep learning. Although self-driving cars are currently being tested, due to safety concerns, they have not yet been considered acceptable for general sale. The fundamental difficulty is that autonomous vehicles must be taught how to respond to a variety of situations that are challenging to anticipate in the actual world. Even when gathering this data is technically possible, it is typically exceedingly expensive. To train self-driving cars effectively, the use of synthetic data may be beneficial.
By using rules, statistical models, simulations, or other approaches, synthetic data is created digitally. There are several benefits to using this type of data, especially when it comes to computer vision. Since real-world data (such as photographs and videos) may contain sensitive personal information that must be kept private and is sometimes challenging to get at a reasonable cost, synthetic data may be more effective and efficient than real-world data. Synthetic data-generating machine learning models can forecast events and produce visuals that are difficult, if not impossible, to locate and foresee in the actual world. We refer to these models as generative models.
Ali Jahanian, a research scientist and the paper's lead author, states that while researchers "were especially pleased when proved that this method sometimes does even better than the real thing," he also cautions about the current risks associated with the use of synthetic data, such as privacy concerns, biased data, and source data disclosure. The innovation of these generative models, in Jahanian's opinion, is their ability to predict outcomes under conditions they were not exposed to when being trained. This unique capability would enable such robots to respond appropriately even under circumstances that the engineers who taught them had not anticipated and gathered in the actual world.
Around the world, car accidents continue to be a leading cause of mortality and suffering. For instance, the number of motor vehicle deaths and injuries in the United States each year is around 35,000 and over 2 million, respectively. While these numbers are little in contrast to the COVID epidemic or cancer, they nevertheless seem like a lot of needless suffering.
In reality, during the past few years, tremendous progress has been made in minimizing these fatalities and injuries. Here are the figures for Germany's road traffic deaths during the past several years. The European Union is positively regulating road traffic. New safety measures that are being gradually made necessary in the EU account for a substantial portion of it. Additionally, new restrictions are the immediate reason for this article.
We are unable to cover every facet of safety monitoring, so let's focus on sleepiness detection in more depth. The regular occurrence of falling asleep behind the wheel is a crucial factor in both new legislation and real auto accidents. Not even being fully asleep is necessary: A microsleep episode, which lasts for 5 to 10 seconds, is more than enough to cause an accident. How, therefore, can a smart automobile detect when you are going to nod off and alert you in time?
Electroencephalography (EEG), which involves gauging your brain's electrical activity, is of course the gold standard for identifying brain states like sleep. Recent studies have used deep learning to analyze EEG data, and it seems that even very straightforward approaches based on convolutional and recurrent networks are sufficient to accurately identify sleep and tiredness. For instance, a recent study by Zurich scientists Malafeev et al. (2020) demonstrates outstanding outcomes in the identification of microsleep episodes with a straightforward design. However, this type of information won't be accessible in a real automobile unless all drivers are mandated to wear headgear with EEG electrodes. Although EEG is frequently used in this sector to gather and categorize real datasets, another signal is required for accurate sleepiness identification.
In this case, two genuine signals are both significant. To identify problematic steering patterns, a system must first be developed that can track the steering angle and velocity using a basic sensor. For instance, it may be a warning that a motorist is growing tired or preoccupied if they are scarcely steering at all for a while before suddenly pulling the wheel back into place. Leading producers like Volvo, Bosch, and others provide solutions on steering patterns.
However, steering patterns are only one potential indication and a very subtle one. Furthermore, if automatic lane-keeping aid is installed as another part of the same EU requirements, steering becomes largely automated and these patterns stop operating. The notion of using computer vision to identify tiredness on the driver's face would be far more straightforward.
Join our WhatsApp Channel to get the latest news, exclusives and videos on WhatsApp
_____________
Disclaimer: Analytics Insight does not provide financial advice or guidance. Also note that the cryptocurrencies mentioned/listed on the website could potentially be scams, i.e. designed to induce you to invest financial resources that may be lost forever and not be recoverable once investments are made. You are responsible for conducting your own research (DYOR) before making any investments. Read more here.