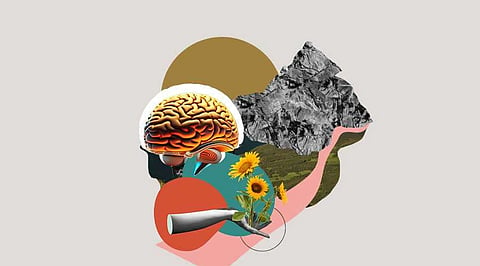
Synapses are the connections that enable nerve cells in the deep brain to communicate with one another. Johns Hopkins University researchers have developed a method that employs artificial intelligence and ML to visualize and track changes in synapses' strength in real animals. The method, detailed in Nature Methods, might help researchers better understand how learning, aging, injury, and illness affect these connections in human brain
Dwight Bergles, Ph.D., compares learning about how an orchestra performs to observing individual performers over time. He holds the Diana Sylvestre and Charles Homcy Professorship in the Solomon H. Snyder Department of Neuroscience at the Johns Hopkins University (JHU) School of Medicine. According to him, the synapses in the brains of living animals are treated in this unique way.
The study's co-authors included Richard Huganir, Ph.D., a Bloomberg Distinguished Professor at Johns Hopkins University and the head of the Solomon H. Snyder Department of Neuroscience, as well as Adam Charles, Ph.D., M.E., and Jeremias Sulam, Ph.D., all assistant professors in the department of biomedical engineering. The Kavli Neuroscience Discovery Institute at Johns Hopkins University houses all four researchers.
Synaptic junctions, or "junctions," are where nerve cells exchange chemical messengers to transmit information. According to the authors, acquiring new abilities and being exposed to new settings are only two examples of how varied life events may alter synapses in the brain, either increasing or weakening the connections that allow for learning and memory. Although it is difficult, understanding how these little changes occur across the trillions of synapses in our brains is essential to understanding how the brain functions normally and how sickness affects it.
The high density of synapses in the brain and their small size, characteristics that make them extremely difficult to visualize even with new state-of-the-art microscopes, have forced scientists to search for better ways to visualize the shifting chemistry of synaptic messaging.
Charles says that they needed to extract the signal portions they needed to see from challenging, blurry, noisy imaging data.
To achieve this, Bergles, Sulam, Charles, Huganir, and their coworkers utilized machine learning, a foundation for computing that enables the flexible development of autonomous data processing tools. Scientists used machine learning to improve the quality of pictures of thousands of synapses since it has been effectively used in numerous fields of biomedical imaging. The system must first be "trained," showing the algorithm what high-quality photos of synapses should look like to be a vital tool for automated detection that can outperform human speeds.
The scientists conducted their studies using genetically modified mice whose glutamate receptors, the chemical sensors at synapses, fluoresced green when exposed to light. In these mice, the quantity of fluorescence a synapse produces is a measure of its strength because each receptor emits the same amount of light. This is because each receptor produces the same amount of light.
Since individual clusters of glutamate receptors at synapses were difficult to view clearly, let alone individually identify and track over time, imaging in the intact brain yielded low-quality images as was predicted. The researchers used photos of brain slices acquired ex vivo from the same kind of genetically altered animals to train a machine learning system to transform them into photographs of greater quality. Because these photos weren't obtained from living animals, creating poor-quality photographs comparable to those acquired from live animals and considerably higher-quality images utilizing a different microscopy approach was feasible.
Join our WhatsApp Channel to get the latest news, exclusives and videos on WhatsApp
_____________
Disclaimer: Analytics Insight does not provide financial advice or guidance. Also note that the cryptocurrencies mentioned/listed on the website could potentially be scams, i.e. designed to induce you to invest financial resources that may be lost forever and not be recoverable once investments are made. You are responsible for conducting your own research (DYOR) before making any investments. Read more here.