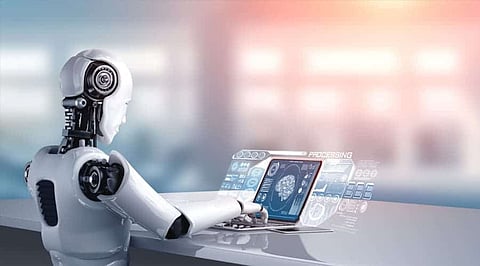
Machine learning is frequently seen as the silver bullet for numerous industries' various issues. Machine learning advancements have appeared to all more rapidly and precisely read radiology checks, recognize high-risk patterns, and diminish supplier's administrative burden.
As organizations venture up the utilization of ML-empowered frameworks in their everyday operations, they become progressively dependent on those frameworks to help them make crucial business decisions. Sometimes, the ML systems work independently, making it particularly significant that the automated decision-making works as intended.
Human bias is surely an unavoidable truth in machine learning. In data science, it for the most part alludes to a deviation from expectation, or a mistake in the data, yet there is something else to bias than that. Once in a while, our viewpoints are not as expansive as we might want to think and accordingly, the huge volumes of data used to train algorithms are not in every case adequately variegated or diverse. As a general rule, there is real human bias in algorithms and data, which basically searches for patterns in the data we feed it
The power of supervised learning, one of the core ways to deal with machine learning, specifically relies vigorously upon the quality of the training data. So it shouldn't be astonishing that when biased training data is utilized to teach these frameworks, the outcomes are biased ML systems. Biased ML systems that are deployed can cause issues, particularly when utilized in automated decision-making systems.
Despite all these biases, there are ways organizations can remove bias in machine learning models
Discover Sources of Data
Discovering sources of data is one approach to address and prevent bias. Further, check how the various types of bias could affect the data being utilized to train the AI model. Have you chosen the data without bias? Have you ensured there isn't any predisposition emerging from mistakes in data capture or observation? Is it true that you are trying not to utilize a historic data set corrupted with bias? By posing these inquiries you can assist with distinguishing and possibly take out that bias.
Pick the correct learning model
Have you wondered why all ML models are unique? Each problem requires a unique solution and gives varying data resources. There's no single model to follow that will prevent bias, yet there are boundaries that can educate your team as its building.
Organizations need their data scientists to distinguish the best model for a given circumstance. Plunk down and talk them through the various procedures they can take when assembling a model. Evaluate ideas before focusing on them. It's smarter to discover and fix weaknesses now, regardless of whether it implies taking longer, than to have regulators discover them later on.
Cleaning Data
From numerous points of view, the most ideal approach to remove bias in ML models is to minimize bias in organizations. The data sets used in language models are excessively enormous for manual examination, however, cleaning them is beneficial. Also, training people to settle on less biased choices and perceptions will help make data that does likewise. Employee training along with an evaluation of historic information is an incredible method to improve models while likewise indirectly addressing other workplace issues.
Employees are educated about normal bias and sources of them. The training models can be pruned or rectified, while the employees ideally become more cautious in their own work later on.
Find Precise Representative Data
Before gathering and amassing data for machine learning model training, companies should initially attempt to comprehend what a representative data set should resemble. Data scientists should utilize their data analysis abilities to comprehend the nature of the population that will be demonstrated alongside the characteristics of the data used to make the ML model. These two things should match to assemble a data set with as meager bias as possible.
Proper Measurement Metrics
Machine learning is complex, and these models exist within bigger human cycles that have their own complexities and difficulties. Each piece in a business process may look worthy, yet the aggregate actually shows bias. A review is an infrequent, deeper assessment of either a part of a business or how a model travels through the entire cycle, effectively searching for issues.
KPIs are values that can be checked to see whether things are moving the correct way, for example, the number of women promoted every year. Audited models might be atypical, and KPIs change normally, yet searching for potential issues is the initial move toward tackling them.