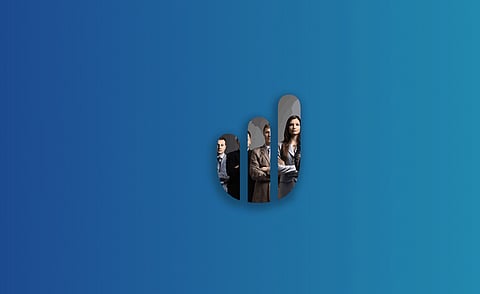
With the rise in data generation, the demand for skilled data analysts and managers have risen in the past few years. In 2018, LinkedIn reported that job shortage for data science talent deepened from July 2015 to July 2018. Even if the job opportunities are there, no person possesses all the analytical and data science skills, therefore, it is important for managers to seek candidates with right temperament rather than narrowing down the specific skills. Amid this situation, some believe that a data science professional should be the jack of all trades and master of one – means – the candidate can embody a hybrid of general skills with mastering one skill in-depth to stay confident.
Organizations nowadays picture their employees with handling responsibilities like data governance, data management, programmatic skills for creating models and awareness of organizational agility requirements.
It has become quite essential to know which analytical skills to choose. Well, it is not suggested to have a knowledge of a specific tool or programming language although having familiarity with such skills is indeed essential. But a lot of tools and programming language are flooding the market with most of them having a similar variation of functional features.
However, the real asset in selecting candidates is to look beyond a specific skill set to examine how and when that skill is applied. This will probably reveal a skill fluidity and depict how they work with others to solve problems.
Additionally, scaling analytics implies orienting professional commitment with strategy. Also, an enticing learning environment will increase capabilities correlating to the need for diverse talent. As the interest in increasing STEM (science, technology, engineering, and math) representation among females and minority professionals is high, it can enhance team diversity. This can bring more perspectives on data analysis to avoid social mistakes experienced by ML initiatives. Instead of focusing on technical solutions it is critical to support focusing on potential insights.
Such solid environment also does a positive sentiment that can encourage morale while facing complex challenges such as developing ML model. The team rich in diversity is capable of better internalizing new knowledge and skill, while organizations enjoy a system that helps to retain that knowledge when erosion occurs.
The business management considers the requirement to maintain the analytic capability to stay relevant and competitive. They are now understanding that advantages achieved exist on a continuum. So a candidate must be hired on his general ability to reason and to interact with other people against which knowledge set they possess.
Join our WhatsApp Channel to get the latest news, exclusives and videos on WhatsApp
_____________
Disclaimer: Analytics Insight does not provide financial advice or guidance. Also note that the cryptocurrencies mentioned/listed on the website could potentially be scams, i.e. designed to induce you to invest financial resources that may be lost forever and not be recoverable once investments are made. You are responsible for conducting your own research (DYOR) before making any investments. Read more here.