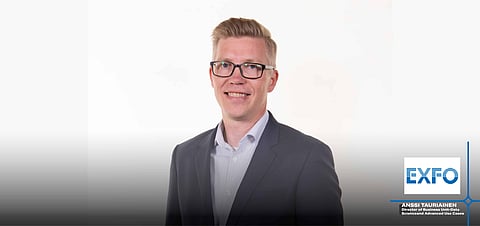
By Anssi Tauriainen, Director of Business Unit – Data Science and Advanced Use Cases, EXFO
Autonomous cars are vehicles controlled with little or no human intervention.
Six levels are used to describe the autonomy of these cars, where level 0 is a fully human-controlled vehicle, and level 5 requires no human intervention at all. Today, the most advanced commercial vehicle models feature level 3 automation, which enables the driver to take their eyes off the road.
Autonomous cars can sense their surroundings and have the intelligence to react accordingly. To do so, autonomous cars rely on internal and external sensors including radar-, sonar- or LiDAR-based systems, computer vision, and GPS, which produce a huge amount of data. It must be processed mostly within the car because current LTE networks respond too slowly and do not have the capacity to transfer the data for outside processing.
There is a lot of external data that could make these vehicles even more aware of the surrounding conditions. For example, a real-time sensor and accident and hazard data from neighbouring cars would help to avoid collisions.
The Need for Predictive Assurance
5G will provide much-needed high-speed, ultra-responsive connections. But before 5G can serve autonomous cars, the service assurance function needs further development.
Autonomous cars require consistent connectivity and stable performance to work reliably, and they need to be aware of when the network conditions are unstable.
Experience with LTE and 3G networks tells us that this is a challenge. Big data and streaming technologies have only just recently enabled a shift towards real-time assurance, providing an instant view of network activity. Ever-growing computing power has enabled a transition from a KPI-based approach to call tracing, which allows monitoring of true service quality on an individual user session level.
However, real-time visibility is not enough. Autonomous cars also need instant, automated communications and predictions in order to adapt to new conditions. The implementation of predictions and a high-level of automation require the usage of more advanced technologies such as artificial intelligence.
Principles of AI-Based Predictive Assurance
Our experience with hundreds of mobile customers enables us to highlight the main principles for the design of predictive assurance systems.
• Geolocation is essential
The majority of connectivity issues take place in the radio network and are usually caused by incorrect RAN settings related to antenna directions or power levels, or by external interference. Accurately geolocated data can fix these issues and eliminate blind spots.
• Use of several assurance methodologies
Call tracing is effective only when the network carries traffic. That is not the case for example in rural areas or when there are sleeping cells in the network. That's why other assurance methodologies such as active testing agents are useful. They can actively test the connectivity when there is no other traffic, revealing sleeping cells and blind spots.
• Use of AI
AI is needed for two important tasks that heuristic systems cannot perform today:
Prediction: machine learning has been proven capable of producing highly reliable predictions.
Self-learning automation: the assurance system needs to execute the right action immediately. Fixed rules are not reliable because 5G networks are dynamic and self-organizing. The system needs to self-adapt and accept human input in the learning process.
• Design of the right machine-learning model
Correlation and prediction are often confused. While a correlation can be used to forecast the next values, it does not imply causation. There can also be hidden variables impacting the correlation.
A prediction based on a single data source has similar limitations. Instead, forecasts based on multiple data sources, such as RAN, core, backhaul and transmission are more reliable.
Many algorithms are effective only for homogenous data, so a combination of several methodologies is essential.
About Anssi Tauriainen:
Anssi brings over 20 years of experience in the telecommunication industry. Prior to joining EXFO through the acquisition of his former company, Anssi was the Founder and CEO of Aito Technologies, a leading provider of customer experience analytics for mobile network operators (MNOs). Previous experience also includes multiple positions at Nokia Networks, including Head of Business Management, Project Manager, R&D Engineer and SW Engineer. He holds a Master's Degree in Computing science, mathematics and telecommunications from University of Jyväskylä and is currently conducting a PhD in research in Communication technology in Helsinki University of Technology.
Join our WhatsApp Channel to get the latest news, exclusives and videos on WhatsApp
_____________
Disclaimer: Analytics Insight does not provide financial advice or guidance. Also note that the cryptocurrencies mentioned/listed on the website could potentially be scams, i.e. designed to induce you to invest financial resources that may be lost forever and not be recoverable once investments are made. You are responsible for conducting your own research (DYOR) before making any investments. Read more here.