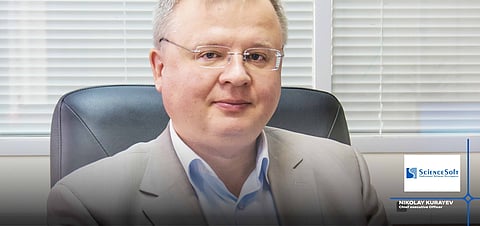
Big data analytics plays a significant role in improving the quality of decision-making and helping organizations achieve efficiencies, improve productivity and growth. A company which enables enterprises to drive digital transformation and use data to gain a competitive advantage is ScienceSoft. In an interview with Analytics, Nikolay Kurayev, CEO of ScienceSoft shares how the company delivers value to clients by harnessing the power of advanced data-driven technologies to transform their business processes, drive innovation and enhance customer experience.
ScienceSoft is an IT consulting and software development company. This means that we can support our customers with professional advisory services, and we are ready to bring all our recommendations and their ideas to life.
Our expertise covers 15+ business directions, including areas such as data analytics, IoT, collaboration and knowledge management, web portals.
We are honored to have our expertise recognized by tech leaders. For example, in 2017, Microsoft awarded us with their Gold Data Analytics Competency. In 2018, we attained another Microsoft Gold Competency – this time, the Data Platform one. The same year, The Silicon Review put us at the top of their list of The 10 Fastest Growing Data Analytics Companies. All these achievements confirm that we are on the right track.
We offer a wide range of data analytics services to satisfy data-related challenges that our customers face. Our service kit includes areas including business intelligence, big data, data science, data management, and data visualization.
We care about effective communication. Our consultants speak the same language as our customers do – the language of business – so, our customers won't find themselves lost in technicalities without a clear understanding of the project's stages and deliverables.
Usually, our customers have to address several challenges where the areas mentioned above are interwoven. For example, the customer wants to implement a big data solution. In this case, ScienceSoft analyzes their short- and long-term business requirements, designs architecture and chooses an optimal technology stack. However, if the customer's big data solution has to do with pattern recognition, data science with its machine learning algorithms will also be there. Additionally, the customer may want an intuitive visualization to easily navigate through huge data volumes.
We understand how inefficient and annoying it is for companies to search for yet another partner for a minor project task and waste their time on re-explaining their status quo and need. That's why we always strive to satisfy the customer's need to the full.
Data analytics is evolving rapidly. As to its trends, I would name big data and data science, though these two are at different stages of their maturity. Data science (disguised under many names, such as machine learning, deep learning, and AI) is still emerging and now at its 'promotion stage' as I call it. At this stage, the value of a particular concept is discussed a lot, though only a few pioneering companies come as far as to bring it on board. The rest of the market players will catch up on its implementation, but this will happen later.
As for big data, it's already an established trend – companies have recognized the value it can bring and are actively engaged in big data implementation and adoption.
Big data has dramatically changed the analytics landscape: tried-and-tested relational databases have finally made room for dedicated big data technologies enabling collection, storage, and processing of huge and ever-growing data volumes. This made both customers and development companies search for the big data-savvy talent, which was scarce at that time (by the way, the problem of the big data talent gap is still acute).
On top of the talent challenge, there are other ones related to the very nature of big data. For example, while designing big data solutions, it's necessary to carefully estimate future data growth. When this part is neglected, a customer is at risk of running out of the storage space in just a couple of months. Another example is available data processing time. A customer won't be happy with the solution that returns insights within a couple of hours, while they are needed within several minutes.
Join our WhatsApp Channel to get the latest news, exclusives and videos on WhatsApp
_____________
Disclaimer: Analytics Insight does not provide financial advice or guidance on cryptocurrencies and stocks. Also note that the cryptocurrencies mentioned/listed on the website could potentially be scams, i.e. designed to induce you to invest financial resources that may be lost forever and not be recoverable once investments are made. This article is provided for informational purposes and does not constitute investment advice. You are responsible for conducting your own research (DYOR) before making any investments. Read more here.