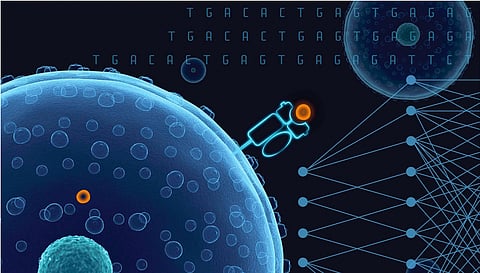
The evolution of AI in recent years has emerged out as a great competitive advantage for businesses. However even if business leaders are familiar with the term, they often stumble over measuring its success or ensuring if they are making the right decisions regarding its implementation or how they can implement AI for better impact. All answers can be explored through a question, whether they are embracing the centralized or decentralized approach for incorporating AI into their systems. And according to Ople.ai's founder and CEO, Pedro Alves, both approaches have their own pros and cons.
As noted by Venture Beat, the first step of any data science project is to define a problem within the business and develop a data set for that problem. Then, according to Alves, one enters the data science technical chasm which is the level at which the data scientist finishes all the technical steps, including feature engineering and algorithm selection, and steps back. Once the AI model is built, the end business user begins to use the solution.
Alves adds, it's within this process the roadblocks happen and the biggest problem with both centralized and decentralized AI is communication. According to him, in a centralized approach, there's great communication within the technical area, but not so much with the business team that's actually going to use the product. Whereas in the decentralized approach there's not a lot of communication within that technical chasm, but there's good communication at the beginning and the end of the process.
When it comes to challenges faced by both, in a centralized approach specifically, the team is isolated from all other groups they're serving within an organization, and they're not well-embedded into the space that these internal customers occupy or the nuances of the problems that each of these business units are facing. This leads to the lack of real communication which creates a problem in building useful data science or useful AI. In the case of a decentralized approach, every team is equipped with its own data scientists, but communication is again the issue. As noted by Venture Beat, in this situation, it's the communication between each group of data scientists within the business as a whole. One gains the advantage of embedding AI capabilities directly within each team, increasing the communication in each individual space. Still, they lose the cohesive approach towards data science for their entire organization.
In more simplified way, we can say that when data scientists work together they tend to operate at their highest level but if isolated they are unable to capture the synergy between projects that might have been developed if they were connected closely with other scientists, teams, and departments.
Amid this, to some companies, the hybrid approach might sound like the best solution but it's not for all of them. It rather works as a band-aid, and could not be considered as an ideal approach as it may not work for all companies but some.
Having a hybrid approach means that companies are trying to solve the problem by building a centralized team to be the driver of technology and innovation for the company, and at the same time, using decentralized teams in the various departments within the company, in order to improve communication between each departmental team and the data scientists. However, it creates a platform for the embedded departmental AI teams to use while empowering them from a technical perspective only.
According to Alves, whether one chooses to go with a centralized or decentralized solution, the way to create processes and scientist-to-business communication is with a platform. If the company has a platform that automates the technical component of a data science project, which is where data scientists spend 99% of their time, suddenly they have time to have conversations within different teams. Instead of two to three months, projects can now be completed in hours to days, while letting centralized departments handle more volume.
Moreover, when it comes to decentralized AI, the same platform solves the problems, but from a different perspective. If all the technical parts are being automated by the platform, the company will have a much easier time hiring a data scientist because the requirements for knowledge and experience are lower. When the same platform is being used across the company, the best practices and best processes can be achieved through the platform.
Furthermore, Alves adds, "No matter which way you go, the automation of the process will solve the problems one way or another. If you're truly automating things in a way that's useful, easy to use, and of high enough quality that a great data scientist would appreciate, you're solving the problem."
*Based on the insights from Venture Beat
Join our WhatsApp Channel to get the latest news, exclusives and videos on WhatsApp
_____________
Disclaimer: Analytics Insight does not provide financial advice or guidance. Also note that the cryptocurrencies mentioned/listed on the website could potentially be scams, i.e. designed to induce you to invest financial resources that may be lost forever and not be recoverable once investments are made. You are responsible for conducting your own research (DYOR) before making any investments. Read more here.