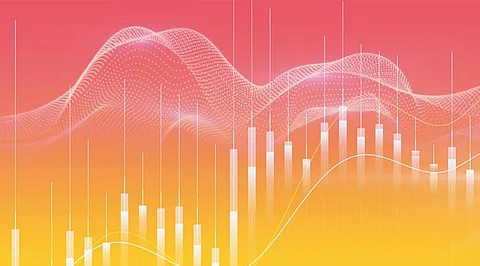
You can read about the potential of data science everywhere. Data is a source of concern for everyone. Businesses are interested in learning how data may help them cut costs and boost their profits. Data science has piqued the interest of the healthcare business, which wants to know how it may help them forecast illnesses and deliver better treatment to its patients.
Data science is being utilized in this way to give an in-depth understanding of the stock market and financial statistics. We purchase, sell, and hold stocks. All of this is done in order to make money.
There are a lot of terms and ideas in Data Science that many individuals are unfamiliar with. We are here to put a stop to it all. A fundamental grasp of statistics, math, and programming is required for data science. Let's go through some financial and stock market-related data science principles.
Algorithms are commonly employed in data science and coding. An algorithm is a collection of instructions for completing a task. You may have heard that algorithmic trading is becoming increasingly popular in the stock exchange. Trading algorithms are used in algorithmic trading, and these programs include criteria such as buying stocks only when it has dropped exactly 5% that day, or selling after the stock has lost 15% of its value since it was originally purchased.
These algorithms can all operate without the need for human involvement. Trading bots are often known as such since their trading strategies are essentially mechanical and they trade without feeling.
This isn't your average workout. Training a machine learning technique in data science and machine learning entails using selected data or a subset of the data. For training and testing, the complete dataset is generally divided into two halves – training data or training set.To create accurate predictions, the deep learning model will need to learn from previous data.
If we wanted to use a machine learning technique to predict the future values of a certain stock, we'd feed it stock prices from the previous year or so and ask it to forecast the following month's prices.
We'd want to understand how well our model performs once we've trained it with the training dataset. This is where the remaining 20% of the data is found. This information is sometimes referred to as testing data or a test dataset. We would examine our model's projections to our assessment set to validate the performance of the model.
Let's imagine we're using one year's worth of stock market data to train a model. The months from January to October will be used to train, while November and December will be used to test. After training our system on values from January to October, we'll use it to anticipate the next 2 months. These forecasts will then be compared with the actual prices from November and December. As we play about with our model, we want to lower the amount of variance between the forecasts and the actual data.
Data is frequently shown in a tabulated form in data science, such as an Excel sheet or a DataFrame. Anything may be represented by these data points. The columns are very significant. Let's assume one column has stock prices, while the other columns provide P/B Ratio, Volume, and other financial information.
The stock values will be our aim in this situation. The features will take up the remaining columns. The goal variable is referred to as the predictor variable in data science and statistics. The characteristics are referred to as independent variables. The goal is what we want the ML model to predict future results for, and the characteristics are what the ML model uses to do so.
Data Science may be utilized to provide us with a new view of the stock market and financial data. During trading, some basic concepts are followed, such as sell, purchase, or hold. Our major objective is to generate a lot of money. Trading platforms are getting more and more popular. To assess if it is worthwhile to invest in a certain stock and undertake stock market research, you must first comprehend some essential principles in Data Science.
Data science is primarily reliant on data modeling and projecting future results. A time series model is being used in the stock market to forecast the increase and drop of share values.
Data science is all over the place. Stock markets, product analysis, and so on. Stock investment analysis is a topic that may be thoroughly investigated using programming. If we add the R language, which has a large literature, as well as the packages and functions developed/imported at this moment. Beginning with simple equations like (1) averaging and (2) linear regression, dealing with historical information on the stock values of a publicly traded firm and employing a mix of machine learning techniques to forecast the future stock price of this organization. All of these factors combine to make stock values very volatile and difficult to anticipate accurately.