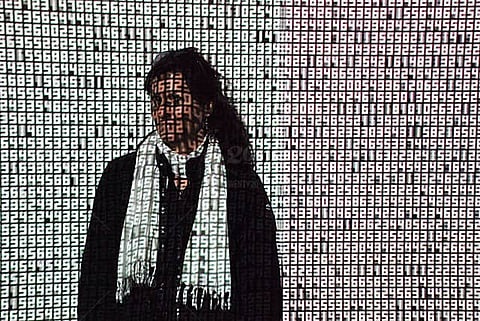
In this age of digital revolution, organizations deal with large volume of data and information emanating from various sources. To maintain a competitive advantage, gain insights and make informed decisions, it becomes necessary to make proper use of the data. However, with increased data generation, the issue of data quality and veracity also becomes important. That's why data quality management (DQM) has become one of the topmost priorities of business executives today.
DQM deals with the acquisition of data, implementation of data processes, distribution of data and overall monitoring of the acquired data. DQM prepares organizations to face the modern digital age challenges effectively. The key to data-driven analysis is provided by DQM.
Big data has accentuated the pace of the digital revolution. Moreover, with increased automation, there has been a large influx of unstructured data. These open up new avenues of potential revenue sources to be exploited. To harness these pool of unstructured data, a far-reaching DQM policy is required. Unstructured data sources are aggravating the need for a better DQM.
Moreover, the benefits of DQM has a trickle-down effect on the company's overall performance. High-quality data will help to understand the customers better and gauge the impact of targeted marketing strategies. Better quality data will lead to the more accurate targeting of potential customers, which in turn will increase customer acquisition and retention.
However, like other breakthrough technologies along with new applications, the digital age has brought with it the ominous effects of poor data quality. Analysis and Insights derived from poor-quality data are of little value to the decision-makers. In fact, instead of facilitating decision-making, such analysis may result in misleading decisions. Moreover, analysis of poor-quality data is a waste of resources.
Poor data quality leads to ineffective optimization of resources, poor decision-making, deceptive perception about customers and lower prospective ROI on businesses. A Gartner study reveals that the surveyed organizations incurred a loss of on an average US$14.2 million owing to poor data quality. So, the cost of poor data quality is very high. The root of all these problems is substandard data quality and the solution is data quality management. With data-driven culture in the top priority list of organizations, data quality management becomes essential today.
The following aspects of DQM should be borne in mind while designing a DQM strategy.
The Role of Individuals
The DQM department needs efficient individuals to successfully implement the whole process. Several DQM roles in all hierarchies will require cognitive and industrious minds. The higher management monitoring the DQM processes will also be required to be prompt and proficient.
Data Profiling
Data profiling is one issue in DQM that requires special attention. Data profiling involves reviewing data in detail, comparison and statistical analysis of the data, and finally reporting the quality of data. This initiates the process of DQM. Data auditors should be deployed to monitor and validate the quality of data.
The quality of any data is affected by its source, method of collection, entry storage, and management. With manual data entry, the chance of lower data quality improves. Moreover, unstructured data has imposed new problems. Along with DQM, data governance is also necessary which allows to filter out the unnecessary information not concerning the business objective.
Defining the Parameters of High-Quality Data
The quality parameters of the data should be clearly defined in alignment with the business objectives and requirements. This will avoid any confusion arising due to the abstractedness of the data quality. This will also prevent misclassification of data. Data inconsistencies should also be reviewed and studied carefully. The data should be cleaned and analyzed thoroughly by all levels and the quality of the data should be assessed meticulously before concluding its nature. Data quality design needs to be done carefully.
Data Reporting
Data reporting should be done by defining metrics and grouping the data based on requirements. Once the data is mapped to respective metrics, it can be reported and presented using dashboards. In most of the cases, there'll be a question on the quality of the data. So, remedial measures to improve the data quality will also be required.
Given the volume of data generated, collected and analyzed by organizations, increasing amount of resources need to be invested in DQM. DQM has been ranked as a top priority by executives seeking to exploit comparative advantage. It is essential to ensure that high-quality data projects accuracy, completeness and integrity. So, for any DQM initiative to succeed, a strong coordination between management, people and technologies would be required.
Join our WhatsApp Channel to get the latest news, exclusives and videos on WhatsApp
_____________
Disclaimer: Analytics Insight does not provide financial advice or guidance. Also note that the cryptocurrencies mentioned/listed on the website could potentially be scams, i.e. designed to induce you to invest financial resources that may be lost forever and not be recoverable once investments are made. You are responsible for conducting your own research (DYOR) before making any investments. Read more here.