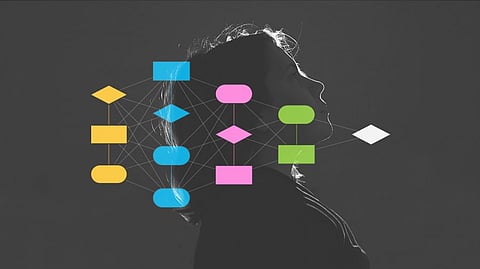
Several types of information technology will likely reduce the frequency of medication errors. However, insufficient data exist for many technologies and most available data come from adult settings. Computerized physician order entry with decision support substantially reduces the frequency of serious inpatient medication errors in adults. Other specific inpatient information technologies may be beneficial even though less evidence is available now. These include computerized medication administration records, robots, automated pharmacy systems, barcoding, smart intravenous devices, and computerized discharge prescriptions and instructions. In the outpatient setting, adherence is essential, so are personalized web pages and World Wide Web-based information.
Can algorithms recognize unusual medication errors more accurately than humans? Not necessarily! A study co-authored by researchers at the Université Laval and CHU Sainte, Justin in Montreal, discovered that one model physicians used to screen patients performed poorly on some orders. The study offers a reminder that unvetted artificial intelligence (AI) and machine learning (ML) may negatively affect medical outcomes.
The co-authors looked at a model deployed in a tertiary-care mother-and-child academic hospital between April 2020 and August 2020. This model was trained on a dataset of over 28 lakh medication orders from 2005 to 2018. These had been extracted from a pharmacy database and pre-processed into more than 10 lakh profiles. Before the data collection, the model was retrained each month with ten years of the most recent data from the database to minimize drift that happens when a model loses its predictive power.
The model's profile prediction was provided to the pharmacists who indicated they agreed or disagreed with each prediction. In all, more than 12,471 medication orders and 1,356 profiles were shown to 25 pharmacists from seven of the academic hospital's departments, mostly from obstetrics-gynaecology.
The researchers' team has reported that the model exhibited poor performance concerning medication orders, about an F1-score of 0.30, while the model's profile predictions achieved a 'satisfactory' version, with an F1-score of 0.59.
One reason for the model's performance issues might be a lack of representative data. Research has revealed that biased diagnostic algorithms may perpetuate inequalities. Scientists recently experimented and found that almost all eye disease datasets come from patients across North America, China, and Europe. That means eye disease diagnosing algorithms are less certain to work well for racial groups from underrepresented countries.
In another research, a team from Stanford University claimed that most US data for studies associating medical uses of AI come from New York, California, and Massachusetts.
Therefore, this study's co-authors say that they don't believe that the model could be used as the only decision-support tool. However, they also think it could be mixed with rules-based approaches to detect medication order issues independent of standard practice. Theoretically, serving pharmacists with a prediction for every order should be better because it explicitly identifies which prescription is atypical, unlike profile predictions that only inform the pharmacist that something is atypical within the profile.
The co-authors wrote that their focus groups indicated a lack of trust in pharmacists' predictions, but they were satisfied to use them as a safeguard to make sure that they did not miss unusual orders, which lead them to believe that even moderately improving the quality of these predictions in future work could be beneficial.
Join our WhatsApp Channel to get the latest news, exclusives and videos on WhatsApp
_____________
Disclaimer: Analytics Insight does not provide financial advice or guidance. Also note that the cryptocurrencies mentioned/listed on the website could potentially be scams, i.e. designed to induce you to invest financial resources that may be lost forever and not be recoverable once investments are made. You are responsible for conducting your own research (DYOR) before making any investments. Read more here.