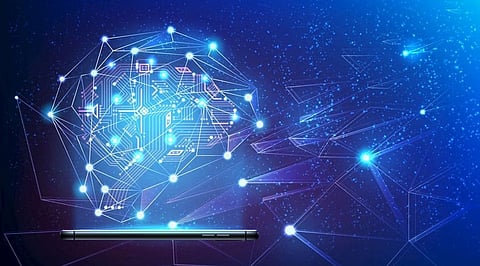
Internet of Things or IoT is slowly transforming the way we collect data and live today. It is also enabling an everyday object to share wireless connectivity with other devices across organizations. This technology is unraveling innovations and concepts across all industrial niches. According to Analytics Insight, IoT is forecast to grow at a CAGR of 10.6% in terms of the global market. It is calculated to expand from US$653.6 billion in 2019 to US$1080.4 billion in 2023.
IoT sensors accumulate large amounts of data through device connectivity via the internet. This data is analyzed and evaluated using tools of artificial intelligence and machine learning. The collected sensor data can be in the form of location, sound or humidity, and different measurements of machines. Once the data insights are obtained, it can be used to compare, calculate, predict and check with existing figures and take relevant follow-up actions. AI also helps to store the bulk of data processed by the IoT devices. Further, together, AI and IoT can propel the development of connected intelligent machines that share information with each other and take well-informed decisions without any human intervention.
It is true that IoT devices and sensors are becoming more ubiquitous. They also minimize the dependence of companies over data scientists and analysts by helping them create a continuous flow of data from their end devices while using less energy, waste fewer products and are light on budget. However, IoT sensors and devices are resourceful as long as they run on power. Without a constant power source, they cannot collect nor transmit data.
To address this issue, researchers from the University of Pittsburgh project have proposed the application of artificial intelligence to extend the lifetime of sensors deployed in IoT. This system will help to cut back on IoT sensors' energy consumption and mitigate battery longevity issues. In this project, the researchers used piggyback sensors, which are powered by energy harvested from the environment, to trigger the main sensors. The piggyback sensors will run unattended and are trained, use AI algorithms, to signal the main devices to turn on only when specific event conditions are met.
Jingtong Hu, the lead researcher on the study and associate professor of electrical and computer engineering at Pitt's Swanson School of Engineering, explained that one of the main challenges of running AI algorithms with energy harvested from the environment is that the energy from the environment is intermittent. "Much like a laptop, if the sensor loses power, you lose the data, so we want to help AI algorithms reach an accurate decision, even with intermittent power," he adds.
Hu and his team, plan to develop a way to save power on the remote sensor device by leveraging energy-harvesting technology, which sources power from the environment, such as solar, thermal, or wind. They will then, add a second, small sensor that can trigger a more robust device, thus saving energy and allowing users to change the battery-less frequently. The smaller sensor shall be powered by energy harvested from the environment. This smaller sensor will run unattended, and with the help of AI, it can be trained to recognize patterns and signal the larger device to turn on during a specific event; thus acting like a watchdog.
According to a blog post on National Science Foundation (NSF) site, Hu and his team have outlined three tasks that shall lay the foundation for conducting intermittent incremental inference on IoT devices powered by energy-harvesting technology. These are:
1. Developing novel power trace aware compression, online pruning and adaptation algorithms to ensure efficient deployment of multi-exit DNNs on intermittently-powered devices.
2. Developing new multi-exit statistical and incremental neural networks (MESI-NN) to further reduce the latency and improve the accuracy and energy efficiency.
3. Designing new neural architecture search algorithms that can automatically search the best MESI-NN architecture. This project will be evaluated with real system and applications such as image classification, keyword spotting, and activity recognition.
The key objective of this proposal is to prolong the lifetime of sensors and devices deployed in remote areas, which will drastically benefit various consumer, business, scientific and national security applications. IoT devices are also used for monitoring and predicting natural disasters. For instance: Sensor technology is currently used to observe gases emitted by active volcanoes in some of the most remote parts of the planet.