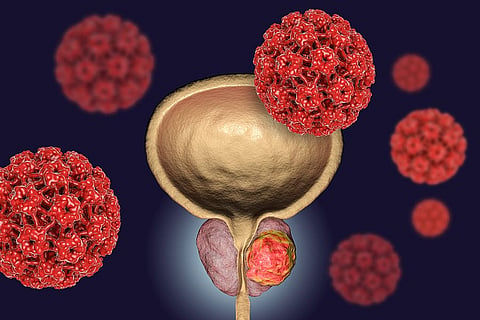
Yearly, around 20 million men are prostate-explicit antigen screened, and 1.3 million experience an intrusive biopsy to analyze about 200,000 new cases, half of which end up being indolent. Around 30,000 men die because of prostate disease (PCa) yearly. Significantly, an estimated US$8 billion is spent on pointless biopsies. Accordingly, an integrative analysis and predictive model of anticipation are expected to help recognize just deadly and aggressive types of the disease.
Prostate cancer growth is predicted to be the main reason for new disease for men and the second most successive reason for death after lung cancer. It is likewise cancer that is difficult to identify, and little injuries can involve only a small amount of 1% of the tissue surface.
Researchers have built up another artificial intelligence (AI) framework to enable radiologists to improve their capability to analyze prostate disease. The framework, called FocalNet, recognizes and predicts the forcefulness of the illness by assessing magnetic resonance imaging (MRI) scans, and does as such with about a similar dimension of precision as experienced radiologists.
FocalNet is an artificial neural system, which utilizes an algorithm that contains more than a million trainable factors, as indicated by analysts, including Ruiming Cao from the University of California, Los Angeles.
The research group from KTH and Karolinska Institutet, driven by Joakim Lundeberg, educator of molecular biology at KTH, utilized information procured from around 6,750 tissue tests with spatial transcriptomics, a technique that combines tissue with quantitative analysis of the active genes, which has been created by KTH and Karolinska Institutet at SciLifeLab.
As indicated by Lundeberg, the utilization of spatial data makes a major contribution. Analysis of prostate tumor gene activity in a tissue segment significantly builds the granularity, compared with regular tumor examination.
According to him, they have exhibited that testing distinctive pieces of a similar prostate tumor indicates amazing contrasts on the gene activity level of the malignancy cells at each site just as the encompassing non-tumor cells, for example, cells identified with inflammation reaction prone to be connected to result of the patient.
One more research from to help take care of the issue, specialists from Cornell University and the Memorial Sloan Kettering Cancer Center, a cancer treatment and research foundation in New York City, built up a deep learning-based methodology that all the more precisely identifies disease. The analysts believe that utilizing the center's biopsy dataset, the team built up a cutting-edge framework that can be considered clinically significant.
The scientists expressed in their research paper that as of not long ago, studies depended on datasets in the order of a couple of many slides which are insufficient to prepare a model that can work at scale in the clinic. Here, they have assembled a dataset comprising of 12,160 slides, two orders of magnitude bigger than past datasets in pathology and identical to 25 times the pixel tally of the whole ImageNet dataset. Given the measure of their dataset, it is workable for them to train a deep learning model under the Multiple Instance Learning (MIL) suspicion where just the overall slide finding is fundamental for training, keeping away from all the costly pixel-wise comments that are typically part of supervised learning approaches.
Given the present endeavors in digitizing the pathology workflow, approaches like theirs can be very successful in structuring decision support systems that can be adequately deployed in the clinics.
Further, Lundeberg believed that having this solid source of data empowers unattended AI techniques to recognize genetic patterns that can't be seen by the unaided eye. By having an immense tissue genetic analysis, it can fill in as a reason for an AI-based clinical assessment of destructive tissues and give understanding into gene expression in the tumor's miniaturized scale condition. He believes that artificial intelligence just encourages us to make computerized tissue life systems.
Emilie Berglund, a doctoral understudy at KTH, stated that early cure of essential prostate malignancy is proficient; in any case, separating those that will advance to aggressive cases and who will benefit by what treatment is as yet hazardous.
Join our WhatsApp Channel to get the latest news, exclusives and videos on WhatsApp
_____________
Disclaimer: Analytics Insight does not provide financial advice or guidance. Also note that the cryptocurrencies mentioned/listed on the website could potentially be scams, i.e. designed to induce you to invest financial resources that may be lost forever and not be recoverable once investments are made. You are responsible for conducting your own research (DYOR) before making any investments. Read more here.