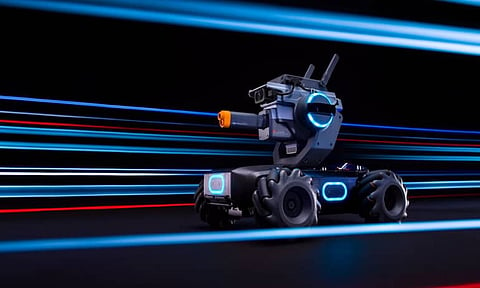
The possibility that robots can figure out how to directly see the affordances of actions on objects (i.e., what the robot can or can't do with an item) is called affordance-based manipulation, explored in research on learning complex vision-based manipulation skills including grasping, pushing, and tossing. In these frameworks, affordances are represented as thick pixel-wise action-value maps that gauge how great it is for the robot to execute one of a few predefined movements in every area.
For instance, given an RGB-D picture, an affordance-based grasping model may construe grasping affordances for each pixel with a convolutional neural network. The grasping affordance value at every pixel would represent the success rate of performing a corresponding motion primitive, which would then be executed by the robot at the situation with the highest value.
A Google and MIT team research whether pre-trained visual representations can be utilized to improve a robot's object manipulation performance. They state their proposed strategy — affordance-based manipulation, can empower robots to figure out how to pick and grasp objects in under 10 minutes of experimentation, which could lay the foundation for profoundly adaptable warehouse robots.
Affordance-based manipulation is basically an approach to reframe a manipulation task as a computer vision task, but instead of referencing pixels to object names, rather associate pixels to the value of actions. Since the structure of computer vision models and affordance models are so comparable, one can use techniques from transfer learning in computer vision to empower affordance models to learn quicker with less information. This methodology re-purposes pre-trained neural network weights (i.e., feature representations) gained from huge scope vision datasets to instate network weights of affordance models for robotic grasping.
In computer vision, numerous deep model structures are made out of two sections: a "backbone" and a "head". The backbone comprises of loads that are responsible for early-stage image processing, e.g., separating edges, recognizing corners, and recognizing colors, while the head comprises network weights that are utilized in latter-stage processing, for example, distinguishing high-level features, perceiving contextual signals, and executing spatial reasoning. The head is regularly smaller than the backbone and is additionally more task explicit. Consequently, it is a common practice in transfer learning to pre-train (e.g., on ResNet) and offers backbone weights between tasks, while arbitrarily introducing the weights of the model head for each new task.
At first, there weren't noteworthy performance gains compared to training the affordance models without any preparation. However, after moving loads from both the backbone and the head (which comprises weights utilized in latter-stage processing, such as recognizing contextual cues and executing spatial reasoning) of a pre-trained vision model, there was a considerable improvement in training speed. Grasping progress rates arrived at 73% in only 500 experimentation grasp attempts and bounced to 86% by 1,000 attempts. What's more, on new objects unseen during training, models with the pre-trained backbone and head summed up better, with getting grasping progress rates of 83% with the backbone alone and 90% with both the backbone and head.
According to the authors, to better get this, they visualize the neural activations that are activated by various pre-trained models and a combined affordance model trained without any preparation utilizing a suction gripper. Strikingly, they find that the intermediate network representations gained from the head of vision models utilized for the division from the COCO dataset initiate on objects in manners that are like the converged affordance model. This lines up with the possibility that moving as much of the vision model as could be expected (both backbone and head) can lead to more object-driven exploration by utilizing model weights that are better at getting visual highlights and localizing obj
Join our WhatsApp Channel to get the latest news, exclusives and videos on WhatsApp
_____________
Disclaimer: Analytics Insight does not provide financial advice or guidance on cryptocurrencies and stocks. Also note that the cryptocurrencies mentioned/listed on the website could potentially be scams, i.e. designed to induce you to invest financial resources that may be lost forever and not be recoverable once investments are made. This article is provided for informational purposes and does not constitute investment advice. You are responsible for conducting your own research (DYOR) before making any investments. Read more here.