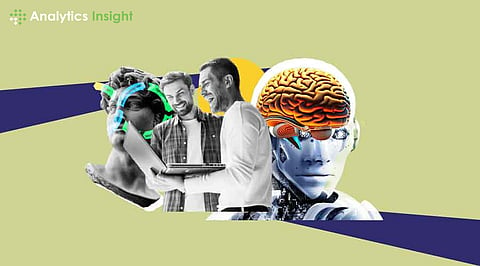
As generative AI continues to expand, the technological landscape is witnessing breakthrough technologies across various industries. Generative AI has transformed the technological landscape that was earlier unimaginable. Today, generative AI has become an important part of our daily tasks. Here, we will explore the genAI advancements and breakthrough technologies to watch:
Generative AI are deep-learning models that are capable of generating top-notch content and images. Generative AI is trained on vast datasets. Artificial Intelligence (AI) works to replicate human smarts in unconventional computing activities such as identifying images, processing natural language, and translating between languages.
Generative AI represents the subsequent phase in AI development.
It can be taught to understand human languages, coding languages, art, chemistry, biology, or any intricate topic. It leverages previously learned data to deal with new challenges.
In the realm of artificial intelligence, the evolution of generative technologies has ushered in a new era of creativity, efficiency, and practicality across various industries. Key advancements like StyleGAN, StyleGAN2, Contrastive Language-Image Pretraining (CLIP), Vision Transformers (ViTs), hybrid models, and the integration of edge computing and on-device AI are revolutionizing how we perceive and utilize AI-driven solutions today.
StyleGAN and its successor, StyleGAN2, represent monumental strides in generative adversarial networks (GANs). These networks are designed to produce exceptionally high-fidelity and realistic images, surpassing previous GAN models by leaps and bounds.
One of their groundbreaking features is the concept of style vectors, which allow for fine-grained manipulation of image attributes such as facial expressions, hair color, and background details. This capability has transformed fields ranging from digital art and logo design to healthcare imaging, where precise visual representations are crucial.
CLIP stands at the forefront of multimodal learning, bridging the gap between natural language and visual data. By training on vast datasets containing paired images and textual descriptions, CLIP leverages contrastive learning to understand and generate visual concepts based on textual input.
Its variant, StableRep+, enhances this capability with added language supervision, setting new standards in AI training efficiency and generative model performance. CLIP's applications span from creative content generation to complex visual tasks in healthcare diagnostics, demonstrating its versatility and efficacy in diverse real-world scenarios.
Vision Transformers (ViTs) mark a significant departure from convolutional neural networks (CNNs) in computer vision tasks. Processing images as sequences of patches using attention mechanisms, ViTs excel in tasks such as image classification, object detection, and visual reasoning.
Their architecture allows for efficient processing of large-scale image data while capturing intricate visual relationships across different patches. Ongoing research, such as the development of fast ViT architectures through generative search, aims to optimize memory usage and improve efficiency further, making ViTs pivotal in advancing both generative AI and traditional computer vision applications.
Hybrid models represent a fusion of generative and predictive AI methodologies, offering robust solutions to complex challenges across various sectors. In medical imaging, for instance, hybrid models combine the creativity of generative AI with the predictive capabilities of traditional AI, enabling accurate diagnostics and personalized treatment plans.
This synergy not only enhances decision-making processes but also revolutionizes data-driven insights and problem-solving approaches in industries ranging from finance to automotive engineering.
Merging Generative AI with edge computing as well as on-device AI is pioneering the new generation of localized real-time applications of AI. Instead of generating the models on the cloud, such architectures can be directly implemented on edge devices like smartphones and IoT devices, which can lead to better security, lesser latencies, and optimized resource usage.
This capability enables breakthroughs in Facebook’s augmented reality filters, unstable chatbots, self-governing cars, and intelligent home appliances where prompt decision-making and customized solutions are inevitable. The list includes memory redesigned specifically for mobile devices, which are developed by Micron Technology and Qualcomm for perfect AI algorithm execution among edge devices.
It also means that the shifts described in this paper are not simply theoretical, but offer a practical result in various industries. In the health sector, generative AI accelerates the rate at which medical images can be analyzed and this enhances early diagnosis of diseases and planning for treatment procedures.
AI is employed in retailing to boost capabilities of recommending systems, and retail interfaces to improve on customers’ experience and the ability to manage stocks. Artificial intelligence and machine learning algorithms are useful for logistics industries and transportation in regard to predicting demand and identifying the most effective and fast routes to create the best results in terms of time and money invested.
The future of generative AI is bright for a long time, and it will keep evolving and deliver more new and useful innovations and uses. New and upgraded hardware including latest processors and efficient memories will support the AI models implementation and extension from the edges to the core and clouds. The study of these approaches and methods to combinations of machine learning frameworks will enhance the understanding of AI applications in the solution of real-world issues with unmatched precision and speed.
Advancements in generative AI, witnessed by landmarks like StyleGAN, Vision Transformers (ViTs), and CLIP, are preparing the stage so that AI enhances human creativity and at the same time transforms industries by enhancing decision-making and personalization. That is why, as these innovations develop, the integration of these technologies in daily usage scenarios implies the existence of smarter, more efficient, and connected global society.
What are StyleGAN and StyleGAN2, and how do they revolutionize image generation?
StyleGAN and StyleGAN2 are advanced generative adversarial networks (GANs) known for their ability to create high-fidelity, realistic images. They achieve this by introducing the concept of style vectors, which enable precise manipulation of image attributes such as facial features and backgrounds. These networks have significantly improved the quality and diversity of generated images compared to earlier GAN models, making them pivotal in fields like digital art, advertising, and healthcare imaging.
How does Contrastive Language-Image Pretraining (CLIP) bridge the gap between textual and visual data?
CLIP leverages contrastive learning to train on large datasets containing pairs of images and corresponding textual descriptions. By learning from these paired inputs, CLIP can associate semantic meanings with visual elements, enabling it to generate and understand images based on textual prompts. This multimodal approach has revolutionized tasks such as image recognition, content creation, and even medical diagnostics, where accurate visual understanding from textual descriptions is crucial.
What role do Vision Transformers (ViTs) play in advancing computer vision tasks?
Vision Transformers (ViTs) represent a paradigm shift from traditional convolutional neural networks (CNNs) by processing images as sequences of patches using attention mechanisms. This approach allows ViTs to capture complex spatial relationships within images, making them highly effective for tasks like image classification, object detection, and visual reasoning. Their ability to handle large-scale image data efficiently and accurately has positioned ViTs as a cornerstone in modern computer vision research and applications.
How do hybrid models integrate generative and predictive AI methodologies, and what are their advantages?
Hybrid models combine the strengths of generative AI, which focuses on creating new content, with predictive AI, which excels in making data-driven forecasts and decisions. By merging these methodologies, hybrid models can generate innovative solutions while also providing insightful predictions and recommendations. In sectors like healthcare, hybrid models enhance diagnostic accuracy and treatment planning by synthesizing patient data with predictive analytics, thereby optimizing patient care and resource allocation.
What benefits do edge computing and on-device AI bring to generative AI applications?
Edge computing and on-device AI empower generative AI applications by enabling real-time processing and personalization directly on devices like smartphones, IoT devices, and edge servers. This approach enhances data privacy, reduces latency, and improves computational efficiency by minimizing reliance on cloud infrastructure.
In practical terms, this means faster response times for interactive applications such as augmented reality filters, chatbots, and autonomous vehicles. Companies are leveraging these technologies to develop innovative solutions that cater to personalized user experiences and efficient data management across various industries.
How is edge computing transforming the deployment of generative AI technologies?
Edge computing is revolutionizing the deployment of generative AI technologies by enabling them to operate locally on devices such as smartphones, IoT devices, and edge servers, rather than relying solely on cloud infrastructure. This approach significantly reduces latency, enhances data privacy, and improves overall computational efficiency.
For generative AI applications, such as real-time image and video processing, edge computing ensures faster response times and more seamless user interactions, even in environments with limited or intermittent connectivity to the cloud. Moreover, edge computing supports personalized and context-aware AI experiences, enabling applications like autonomous vehicles, augmented reality, and interactive chatbots to function autonomously and efficiently without continuous reliance on centralized servers.
This paradigm shift towards edge deployment of generative AI not only improves performance and user experience but also opens up new possibilities for innovative applications across industries, from healthcare and retail to smart cities and industrial automation.