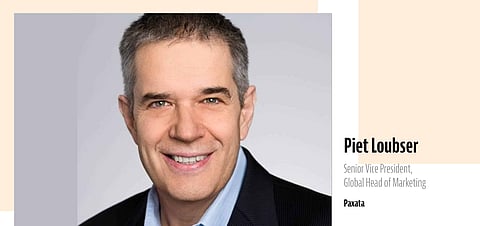
Business intelligence (BI) solutions have been around for decades with their promise of helping organizations perform ad hoc analysis, etc. The move towards agile and self-service analytics has seen tools like Tableau, Power BI, Arcadia Data, and many others experiencing considerable growth, as these newer generation of tools offered enhanced self-service analytics and a far superior user experience than traditional tools like SAP BusinessObjects or IBM's Cognos.
The not-so-obvious key to agile self-service analytics, however, has little to do with the visualization agility but, rather, how to get the data into a format to do the analysis. And in a world where data silos and cloud adoption are exploding, and big data is in the mainstream, it comes as no surprise that we are still spending around 80 percent of our time on the data collection and data preparation aspects and only 20 percent on insights development and visualization.
The traditional method of solving the data preparation task was to ask someone in IT where you would then be placed on a long list of similar requests and have to wait your turn. The emergence of self-service data prep tools has gained tremendous speed as users sought to regain their agility and, as a result, these solutions are now not only recognized as a market segment, but also as a critical part of any data strategy. In fact, Forrester supports this and offers their own perspective on these markets in the following research reports: Forrester Wave: Big Data Fabric, Q2 2018 and Forrester Wave: Data Preparation Solutions, Q4 2018.
At the heart of any successful self-service data prep solution is the user experience and its ability to empower the business consumer or data analyst to do it by themselves. By removing the need to call upon the scarce IT developers, these business consumers have the context of the meaning of the data and they understand what they are trying to achieve with the data they are preparing. They can interact with the data visually, ideally at scale, and see all the data rather than rely on samples only. Then, the tools will typically use embedded algorithms and artificial intelligence (AI) to automatically profile and guide on ways to improve, clean, shape, and combine the datasets. Think of it as offering technical know-how to the casual users who do not have that depth of knowledge. The upside of self-service data prep is that it now truly empowers self-service and agile analytics or data science initiatives. It also speeds the end to end process, as it involves more people in the process than the select few elite technologists or data scientists.
While the embedded algorithms are useful, it is still done step by step and usually with the help of a user selecting data column by column. For instance, when you select the State column, it can profile that there are 84 different values in the column, which for the U.S.is a problem. The algorithms can now automatically cluster the similar values and suggest a way to standardize on the preferred list of states.
The good news is, with the advances in AI and machine learning (ML), we can now move beyond helping a single small sub-task and intelligently create a series of steps to provide guidance on complete task; end-to-end. In the context of data prep, the system can let you pick the main datasets you wish to work with and propose an entire recipe of how to shape, clean, combine, and enrich the data. Rather than relying on the individual to build a recipe step by step, they now review the pre-built recipe and adjust based on specific needs and publish the results. In this way, the system takes control and automatically drives towards a proposed outcome to enable:
• Working at full data scale: Needless to say, one of the challenges with using AI or ML techniques is that they need to know everything they can to make the best recommendation. In the case of data prep, working on a sample of the entire dataset implies the machine's recommendations are going to be limited to what it discovers in the sample. This makes the full power of AI or ML a meaningless endeavor.
• Collaboration and sharing to empower organizational learning: Data and agile analytics are becoming the ultimate corporate team sport. For true organizational learning to take place, we have to combine the machine learning aspects with the human learning side. For example, the machines and the users need to be able to augment each other to ultimately generate better knowledge and insights. Having a data prep solution that lives across multi-departments and/or organizations can provide more effective insights and intelligent self-driving recommendations than installed desktop software packages operating in isolation.
• Use among every person, process, device, and application: The biggest challenge in unleashing the power of self-service and ultimately self-driving data prep is the inclination from teams to think and act in silos. It does not help to create small data/insight-rich groups in our organizations while others are stuck in a world where they wait to be served by ever shrinking IT departments.
Similar to our experience with so-called self-driving cars, this journey will take some time to mature and reach its full value. The upside in the data space is that the incentive to use data to drive better decisions, customer experiences, or patient outcomes, and/or deliver new products and services are on the mind of every executive whether in business or in government. The winners and losers of the next decade will be determined by those who can master their data faster and better than their competitors.
Join our WhatsApp Channel to get the latest news, exclusives and videos on WhatsApp
_____________
Disclaimer: Analytics Insight does not provide financial advice or guidance on cryptocurrencies and stocks. Also note that the cryptocurrencies mentioned/listed on the website could potentially be scams, i.e. designed to induce you to invest financial resources that may be lost forever and not be recoverable once investments are made. This article is provided for informational purposes and does not constitute investment advice. You are responsible for conducting your own research (DYOR) before making any investments. Read more here.