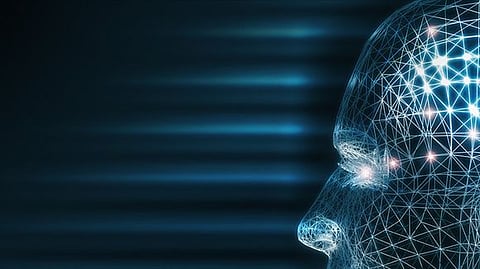
More than most different fields, AI has been put to use in healthcare to check whether it can discover new solutions for old issues in the information. Up until now, it has demonstrated promise in improving medical procedures, expanding services, and finding novel ways of offering care.
As much as AI will undoubtedly change the business, in any case, doctors and nurses will stay an essential part of the healthcare experience. Daniel K. Sodickson, a radiology teacher at New York University working with Facebook on a project to accelerate imaging from MRIs, says the most common misperception that he experiences is that a doctor's main responsibility is to glance through the data and discover the issue. It's a little part of what doctors do.
Analyzing MRI images has demonstrated to be an especially beneficial area of AI research. Over and over, technologists have indicated that algorithms can spot abnormalities in X-rays as well as, if worse than, radiologists. In a now notorious New Yorker story, computer scientist Geoffrey Hinton, one of the individuals generally answerable for the current AI boom, was cited saying that "we should quit training radiologists at this moment."
Artificial intelligence is getting progressively sophisticated at doing what people do, yet more productively, more rapidly and at a lower cost. The potential for both AI and robotics in healthcare is tremendous. Much the same as in our consistent lives, AI and robotics are progressively a part of our healthcare ecosystem.
During medical surgery and other critical care procedures, ceaseless checking of blood pressure to recognize and maintain a strategic distance from the beginning of arterial hypotension is crucial. New machine learning innovation created by Edwards Lifesciences has demonstrated to be a viable method for doing this.
In the prodromal phase of hemodynamic instability, which is described by inconspicuous, complex changes in various physiologic factors one of a kind dynamic arterial waveform "signatures" are formed, which require machine learning and complex element extraction methods to be used.
His team's success offers true proof regarding how best in class advanced analytics can be utilized to educate clinical practice by training and approving machine learning algorithms utilizing complex physiological information. Machine learning approaches were applied to arterial waveforms to build up an algorithm that observes subtle signs to predict hypotension episodes.
What's more, real-world evidence and advanced data analytics were utilized to quantify the relationship between hypotension exposure duration for various thresholds and basically sick sepsis patient morbidity and mortality outcomes. This innovation has been in Europe for the last three years, and it has been utilized on a large number of patients and has been accessible in the US for about a year now.
Technology applications energize healthier behaviour in people and help with the proactive management of a solid way of life. It places consumers in charge of health and prosperity. Moreover, AI builds the ability for healthcare professionals to more readily comprehend the everyday patterns and necessities of the individuals they care for, and with that understanding, they can give better criticism, guidance and support for remaining healthy.
Artificial intelligence is as of now being utilized to detect diseases, such as cancer, all the more precisely and in their early stages. As indicated by the American Cancer Society, a high extent of mammograms yield bogus outcomes, prompting 1 of every 2 healthy ladies being told they have cancer. The utilization of AI is empowering review and interpretation of mammograms multiple times quicker with 99% precision, lessening the requirement for pointless biopsies.
The expansion of consumer wearables and other medical devices combined with AI is likewise being applied to oversee early-stage heart disease, empowering doctors and different parental figures to all the more likely monitor and recognize potentially life-threatening episodes at earlier, more treatable stages.
Machine learning models could furnish doctors and masters with data that will help prevent readmissions or other treatment options, or help forestall things like delirium, current areas of active improvement. Notwithstanding blood pressure, machine learning could locate an extraordinary use in the ICU, in predicting sepsis, which is critical for patient survival. Having the option to process that data in the ICU or in the emergency department, that would be a critical zone to utilize these machine learning analytics models.
The manner in which you mark the information, and what information you consider for the training is critical. Regardless of whether you have so many patients and incorporate inappropriate information, that won't help, it's a little bit of an art to finding the right information to place into the model. On the clinical side, it's essential to mention to the clinician what the issue is, in this case, what is causing hypertension.
Artificial intelligence and machine learning permit those in training to experience naturalistic simulations such that simple computer-driven algorithms cannot. The advent of natural speech and the ability of an AI computer to draw in a split second on an enormous database of situations implies the response to questions, decisions or advice from a trainee can challenge in a way that a human cannot. What's more, the training program can gain from past responses from the student, implying that the difficulties can be ceaselessly adjusted to meet their learning needs. What's more, training should be possible anyplace; with the power of AI implanted on a cell phone, quick catch up sessions, after a tricky case in a clinic or while traveling, will be possible.
Join our WhatsApp Channel to get the latest news, exclusives and videos on WhatsApp
_____________
Disclaimer: Analytics Insight does not provide financial advice or guidance. Also note that the cryptocurrencies mentioned/listed on the website could potentially be scams, i.e. designed to induce you to invest financial resources that may be lost forever and not be recoverable once investments are made. You are responsible for conducting your own research (DYOR) before making any investments. Read more here.