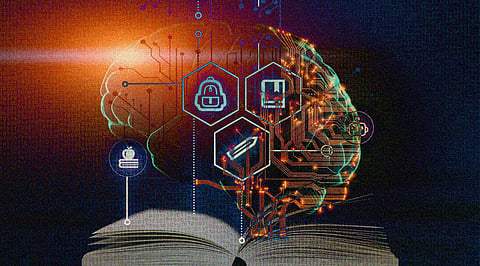
As artificial intelligence technologies have gained popularity, creators and users have used and modified a variety of terms and phrases to describe and characterize their work. One may probably have heard terms like "narrow AI", "deep learning", "neural networks", and other new descriptors being used to distinguish between distinct types and roles of AI, different sections of AI solutions, and so on. Another result of the rapid expansion of AI's availability and use has been the demand for "explainable AI," or approaches and procedures that make Artificial Intelligence more accessible to all types of humans.
And, while explainable AI is important, it's not really the answer always. The ambition to understand how computers make decisions is admirable, but XAI technologies or approaches will never be sufficient. Instead, we should be considering how to guarantee full confidence for these systems' conclusions by discussing how to deliver "understandable AI".
From insurance claims and loans to medical diagnostics and employment, businesses are increasingly relying on AI and machine learning (ML) systems. Consumers, on the other hand, have grown increasingly suspicious of artificial intelligence.
The concept of explainability in AI systems is nearly as ancient as the discipline itself. Many interesting XAI techniques have arisen from academic research in recent years, and many software businesses have surfaced to bring XAI tools to the consumer. The problem is that all of these methods regard explainability as a purely technical issue. In truth, the demand for Artificial Intelligence explainability and interpretability is a far wider economic and social issue that necessitates a more comprehensive answer than XAI can provide.
Most crucially, the widespread availability of AI solutions has made them more accessible to workers with only rudimentary expertise in data science and artificial intelligence. Only people with technical competence could or would develop an AI solution in the past. Many of these solutions are ready to use right out of the box for someone like a department manager to deploy on their own, or you can download them straight to your phone. Artificial Intelligence currently has a significant impact on our lives on both a daily and a long-term basis. Today, Artificial Intelligence could prevent you from getting some tedious, time-consuming chores at work, and it could potentially make a life-saving diagnosis at a medical consultation.
1. If someone wants to feel comfortable while using AI for their work, they have to first understand how it works, personalize it, choose the best solution from a variety of options, or tune it to operate more successfully for them.
2. People have a right to know how Artificial Intelligence works for ethical reasons, as it is used to make choices in areas such as healthcare, law, finance, and other areas where moral and ethical limits are vitally important to most people. Explainability is a method of delving into what is commonly referred to as "AI's black box" and explaining solutions in words that are accessible to the general public. It also aids us in answering vital communal concerns such as "How was this critical decision made?" and "Were there any other viable options?"
Our solution's adoption hinged on its capacity to be explained more clearly. Explainable AI can only help us get closer to our objective of using AI to execute the tasks it's best at while humankind continues to innovate and progress. We can better spend our resources and generate tremendous efficiency on every level if we can identify when our Artificial Intelligence works when it fails, and why. The truth is that most "explainable" AI products can only be understood by someone with a sound knowledge of technology and a thorough understanding of how the model works.
XAI is a crucial part of a technologist's toolset, but it isn't a realistic or scalable technique to "explain" AI and machine learning systems' judgments.
Transparency is the cornerstone of comprehension. Every decision taken by the model they supervise should be accessible to non-technical persons. They ought to be able to check a database based on important factors to assess judgments both individually and collectively. They should be able to do a counterfactual analysis of individual decisions, altering particular factors to see if the outcomes are as predicted.
The greater environment in which the models' function must also be included in understandable Artificial Intelligence. Business owners should be able to see that human decision-making both preceded and accompanied a model throughout its lifecycle to develop trust.
"User-first" approaches should be applied to AI-driven solutions. Understandable AI blends engineers' technical skills with UI/UX specialists' design usability understanding as well as product developers' people-centric design. People may participate in the decision-making procedure in an AI-driven organization if the AI is intelligible. The incorporation of non-data scientists into the creation and design of AI products is also crucial to the Understandable AI process, demonstrating the importance of workforce upskilling for the future AI economy.
To assess whether a financial transaction is fraudulent, for example, an algorithm could be utilized. An algorithm is a logical solution to this challenge, given the millions of transactions that take place every day. AI can wrongly identify transactions as fraudulent (false positive) or miss a fraudulent activity (false negative) which carries the risk of losing a customer's confidence. For this Understandable AI needs to be in place.