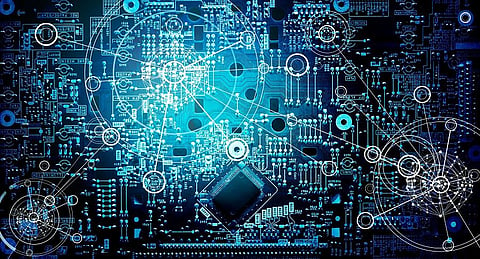
According to Figure Eight's Annual Data Science Report, 89% of data scientists love their activity, up from 67% in 2015. 49% of data scientists get reached in any event once every week for a new job. Data Scientists are essentially more inclined by almost 75% to trust that AI will be great on the planet when compared with 39% of morals experts.
A ton has changed since the organization's unique Data Science Report in 2015. Machine learning ventures are increasing and an ever-increasing number of data is required to drive them. Data Science and machine learning employments are LinkedIn's more quickly developing occupations. Also, the web is making 2.5 quintillion bytes of information every day to power every last bit of it.
Until a couple of years back, just a bunch of us had known about data science, which is extremely popular at this point. As per GreenBookBlog, Danish PC researcher Peter Naur is credited for introducing the term data science. Throughout the years, data science has developed to wind up more persistent, and we are currently seeing novice business analysts and students looked with the possibility of building their own analysis programming and strategies. Another key pattern that has been witnessed is the means by which the remarkable development of information has pushed conventional factual methods to the edges and how deep learning has reformed software engineering.
Be that as it may, many appear to have overlooked the essential meaning of a data scientist. American mathematician and computer scientist DJ Patil characterized it basically: "A data scientist is that one of a kind mix of abilities that can both open the experiences of data and recount an awesome story by means of the data." Yet, the passion and energy around data science has turned out to be intense to the point that working experts are hurrying in to learn machine learning, computer vision, text mining. Then again, they are getting searched for gold key statistical ideas, for example, distribution or certainty that frame the premise of data science.
Over these three decades, we've seen patterns and moves, an advancement of sorts, in the central underpinnings of improvement and application inside this intriguing profession. Let's have a look at the accompanying resolutions that show the advancement of the data science work in the course of recent decades.
What can be built and fit over a genuine situation has the terrifying prerequisite of making a difference. Modeling for demonstrating purpose is never again a thing, and best-fit diagnostics are less critical than best-fit for the circumstance. In the event that a model goes unused, it fills no need. We can never again endure or bear the cost of the advantage of building models only for R&D purposes without thought of use.
The current focal point of enterprises is on using big data which develops analytics solutions for satisfying client goals. Be that as it may, the genuine substance of what data scientists do stays equivocal. For instance, a research refers that progressively, researchers are looked with the challenge of working with expansive, heterogeneous, and noisy datasets. Most new contestants have practically zero involvement with cutting-edge data science strategies and advancements. These people need to discover chances to connect beyond their present range of abilities and current disciplinary methodologies.
Understanding the inside operations of the black box has turned out to be less imperative, except if you are the maker of the black box. Less data scientists with genuinely deep learning of statistical strategies are kept in the lab making the secret elements that ideally get coordinated within tools. This is to some degree baffling for long time data experts with thorough statistical foundation and understanding, however, this way might be important to genuinely scale modeling endeavors with the volume of information, business questions, and complexities we currently should reply.
As organizations progress from data poor enterprises to data-rich, wide experience and an intensive foundation in both data science and pure sciences will be required. With institutes hurrying to overcome any issues and adjusting educational programs to current industry request, the supply gap will steadily diminish. However, as individuals in their late 20s, 30s and even 40s hope to turn towards a profession in data science, they ought to essentially expand on critical, applied learning and get genuine hands-on understanding. One can't turn into a data analyst with only one analytics track or online accreditation, one needs to augment a solid applied statistics program. Hands-on experience can go far in clearing the most troublesome ideas of data science.
Understanding the significance of the human – machine mix and correlative basic decision-making aptitudes from each seems to have advanced all the more decisively into our field of comprehension.
As the field develops, the job of data scientists will evolve. One of the definitions being bandied around is that data scientists are experts in statistics. In any case, it may not be the situation with the current part which has floated from the engineering field. We have frequently heard that data science can't be more than statistics. Sean Owen, Director of Data Science at Cloudera noticed that statistics and numerical processing have been associated for a considerable length of time, and as in every aspect of computing, we generally ache for approaches to analyse somewhat more data. As indicated by John Tukey's paper The Future of Data Analysis, statistics must wind up worried about the dealing with and processing of data, its size, and perception. In any case, today many individuals from different background, even economics, guarantee to be data scientists.
Truth be told, the research additionally spread out a couple of characterized occurrences where a portion of the data science-related tasks could turn out to be totally automated, robotized selection and tuning. The tasks that will end up being the center range of abilities later on are, highlight building and model approval, comprehension of the area, machine learning.
In the long run, there will be more spotlight on parallel and dispersed code as experts who were dependent on spreadsheet examination will move to Python and R.
Join our WhatsApp Channel to get the latest news, exclusives and videos on WhatsApp
_____________
Disclaimer: Analytics Insight does not provide financial advice or guidance. Also note that the cryptocurrencies mentioned/listed on the website could potentially be scams, i.e. designed to induce you to invest financial resources that may be lost forever and not be recoverable once investments are made. You are responsible for conducting your own research (DYOR) before making any investments. Read more here.