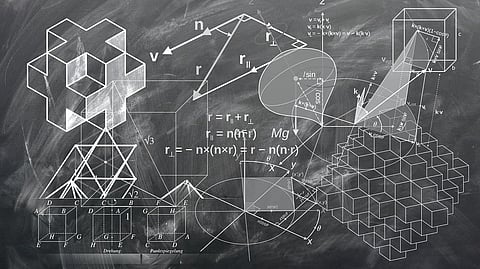
Data science has been a buzzword for almost a decade now. Yet the fact that it is coming up with new features every year never ceases to leave a lasting impression. As companies rapidly are beginning to realize the importance of data as an asset, there occurs a paradigm shift towards data-oriented businesses. More is data generated; more is the hunger to handle it. And this is necessary to draw meaningful and actionable insights from the database of varied formats. Hence to stay in the competition and align with market demands, tech giants must roll out new trends to retain the engagement and innovative factor. Here are 5 top data science trends to be a witness in 2020.
Most of the companies have relied on spreadsheets or SQL for their analytics. But now these companies are slowly reaching an inflection point while data becomes more and more complex. These may include blending data from multiple applications, data of different formats and parameters, etc. Since this is beyond the abilities of the ordinary spreadsheet, graph analytics can be the new normal. It can help firms by connecting dispersed and diverse data points. This will augment data preparation and enable more intricate and flexible data science.
This year may see the integration of different verticals like IT using artificial intelligence and machine learning as a bridge to data science. This can automate finding and surfacing vital analytics and repetitive patterns in an iota of time. Also, it will lighten the dependency of data experts and drive data literacy of the whole organization. With this, there will increased purchase of business analytics tools and platforms.
The cost of In-memory is decreasing. This will drive more analytics to real-time environments. The demand for real-time analytics needs fast CPUs and in-memory processing. As the applications to provide an instantaneous response, services, and alerts to customer increases, the companies will want to opt for faster assistance. They are thereby piloting sudden changes in financial markets and portfolios.
This has the potential to replace reliance on cloud computing and Big Data analytics. Furthermore, it can resolve problems regarding bandwidth costs, eliminates latency, and provides secured connectivity. It can process data locally, allow filtration of sensitive data at the source itself.
Previously commercial vendors were slow to respond to market data analytics. Now being equipped with connectors into the open-source platforms using commercial ML, it can reap benefits like project and model management, reuse, transparency, and integration which open vendors lacked—thus boosting the disposition of models in production, which will push business value to new heights.
Along with this AutoML will help power the development and management of ML templates for added accessibility across the organization. It is thus making the whole process cheaper and easier to deal with. As a result, it can accommodate companies of smaller or non-technical scale.
Therefore both these features will enable minor firms to gain success and higher profitable productivity at a fraction of costs.
Join our WhatsApp Channel to get the latest news, exclusives and videos on WhatsApp
_____________
Disclaimer: Analytics Insight does not provide financial advice or guidance. Also note that the cryptocurrencies mentioned/listed on the website could potentially be scams, i.e. designed to induce you to invest financial resources that may be lost forever and not be recoverable once investments are made. You are responsible for conducting your own research (DYOR) before making any investments. Read more here.