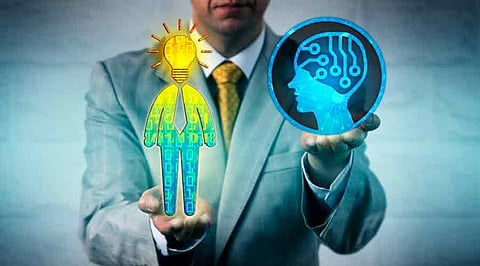
-------
The adoption of Artificial Intelligence is gaining momentum, but the fairness of the algorithmic structure is heavily scrutinized by the federal authorities. Despite many efforts made by the organizations to keep their AI-services and solutions fair, the permeating and pre-existing biases in AI has become challenging in recent years. Big tech organizations such as Facebook, Google, Amazon and Twitter amongst others have faced the wrath of federal agencies, over the recent months.
Owing to the death of George Floyd and the #blacklivesmatter movement, the organizations have become vigilant regarding the operational framework of their AI. With federal, national and international agencies constantly pointing at the discriminatory algorithms, the tech start-ups and organizations are struggling to make their AI-solutions fair.
But how can organizations keep clear from deploying discriminatory algorithms? What solutions will thwart such biases? The legal and statistical laws, articulated by the federal agencies to a large extent help in quelling down the algorithmic biases. For example, the existing legal standards in the laws like the Equal Credit Opportunity Act, the Civil Rights Act and the Fair Housing Act and other chartered acts, alleviate the possibility of such biases.
Moreover, the effectiveness of these standards depend upon the nature of algorithmic discrimination that organizations are subjected to. Currently, organizations are faced by two types of discriminatory framework, which is either intentional or unintentional. These are known as Disparate Treatment and Disparate Impact respectively.
Disparate Treatment is intentional employment discrimination with the highest legal penalties. Organizations must avoid getting engaged with such discrimination while adopting AI. Moreover, by analyzing the record of employee behavior, disparate treatment can be avoided.
Disparate Impact, also the unintentional discrimination occurs when policies, practices, rules or other systems that appear to be a neutral result in a disproportionate impact. For example, certain test results eliminate minority applicants unintentionally or disproportionately is Disparate Impact.
Disparate Impact is heavily influenced by the inequalities of society, and it becomes extremely difficult to avoid them as it exists in almost all areas of the societal framework. Unfortunately, organizations do not have a specific solution that can aid in immediate rectification of disparate impact. The tenants of disparate impact are so deeply engraved that identifying them becomes tedious, and often organizations do not want to indulge in. For example, there is no proper definition of 'fairness' in society. The word is discriminatory in terms of racial context, but in the organizational set up it signifies accuracy. These two concepts, along with two dozen more, complicate the process of algorithmic training.
Additionally, a Google blog explains the fairness in machine learning systems to be derived from the lending problem. Hansa Srinivasan, Software Engineer, Google Research states, "This problem is a highly simplified and stylized representation of the lending process, where we focus on a single feedback loop in order to isolate its effects and study it in detail. In this problem formulation, the probability that individual applicants will pay back a loan is a function of their credit score. These applicants also belong to one of an arbitrary number of groups, with their group membership observable by the lending bank."
A paper named "Delayed Impact of Fair Machine Learning" by the Berkeley Artificial Intelligence Research points that machine learning systems trained to minimize prediction error may often exhibit discriminatory behavior based on sensitive characteristics such as race and gender. Lydia T. Liu, the lead researcher and the author of the paper states that "One reason could be due to historical bias in the data. In various application domains including lending, hiring, criminal justice, and advertising, machine learning has been criticized for its potential to harm historically underrepresented or disadvantaged groups."
The researchers and Statisticians have formulated many methodologies that could abide by the legal standards. One such methodology proving comparatively effective while dealing with algorithm discrimination is 80% rule. Formulated in the year 1978, by EEOC, Department Of Labor, Department of Justice, and the Civil Service Commission, it setups guidelines for Employee Selection Procedures.
The four-fifths or 80% rule is described by the guidelines as "a selection rate for any race, sex, or ethnic group which is less than four-fifths (or 80%) of the rate for the group with the highest rate will generally be regarded by the Federal enforcement agencies as evidence of adverse impact, while a greater than four-fifths rate will generally not be regarded by Federal enforcement agencies as evidence of adverse impact. A ratio below 80% would help in identifying the adverse effects of discrimination. Other metrics such as standardized mean difference and marginal effects can also be effective for identifying unfair AI outcomes.
However, often companies get entangled in the legal investigation due to disparate impact, despite employing the 80% rule or the analysis of the marginalized effects.
As 80% rule is often regarded as insufficient to identify the disparate impact, the organizations must also abide by the standardised norms for the regulated companies. They must constantly monitor for any signs indicating Disparate Impact and document all their attempts to reduce the algorithmic unfairness. And they must formulate coherent and comprehensive clarification about the AI-models they deploy.
Discrimination in Artificial Intelligence models is inevitable. Since there is no definitive model to retain the discriminatory impact, the companies must deploy smart and intelligent strategies to rectify the unfairness and discrimination of their AI.
Join our WhatsApp Channel to get the latest news, exclusives and videos on WhatsApp
_____________
Disclaimer: Analytics Insight does not provide financial advice or guidance. Also note that the cryptocurrencies mentioned/listed on the website could potentially be scams, i.e. designed to induce you to invest financial resources that may be lost forever and not be recoverable once investments are made. You are responsible for conducting your own research (DYOR) before making any investments. Read more here.