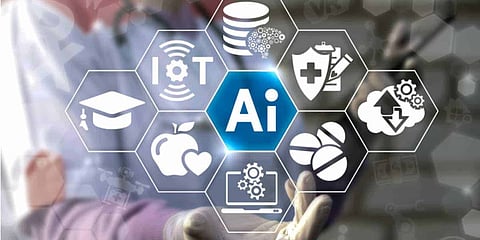
For quite a while, artificial intelligence and machine learning models are leveraged in the healthcare industry to improve patient outcomes. They have been utilized in various scans, for diagnosing various diseases, for the drug manufacturing and planning the treatment for various diseases. The involvement of these AI/ML models is observed in the surgical process as well.
With the amount of data being generated nowadays, the traditional AI/M- based software models are often scrutinized under the lens of performance and accuracy. As new advances are shaping the future of healthcare, the modification of the existing software models has been recognized by healthcare professionals.
Now to improve the patient's outcome, the FDA has proposed a regulatory framework for Artificial Learning and Machine Learning technologies for compliance by the manufacturers. As AI/ML models demand training the datasets, the new modification provides options on which SaMD can be trained by the manufacturers.
A study named "Proposed Regulatory Framework for Modification to Artificial Intelligence/Machine Learning Model (AI/ML)- Based as a Medical Device (SaMD), suggests developing policies that are appropriately tailored for SaMD to ensure that safe and effective technology reaches users, including patients and healthcare professionals.
James Smith, the Study Lead from the University of Oxford, said "The process for developing regulations is, roughly, to get feedback from the public on its initial proposal, make changes and draft regulations or guidance, get more feedback, and eventually finalize,"
The team welcomed recommendations and comments associated with the proposed framework and analyzed all the 125 publicly available comments from the manufacturers on the FDA proposal from April 2, 2019 to August 2019.
The FDA's Centre for Devices and Radiological Health (CDRH) has also referred to the need for software modification for the risk-based approach in treating diseases.
In this article, we will observe the relevant points that are made for successful modification in the framework.
The paper used the definition proposed by James McCarthy for AI and ML models to be the basis of the medical devices. It says that the AI/ML-based software is intended to treat diagnose, cure, mitigate and even cure the pre-existing diseases and hence, are termed as Software-as-a- Medical Device. The intended use of the SaMD depends upon the risk categorization Framework.
The AI/ML-based SaMD is governed by locked algorithms and continuously learning algorithms. The locked algorithms produce the same type of results each time, and apply fixed-function to a given set of inputs. These algorithms are manually operated for updates and validation.
The adaptive learning model, however, alters its behavior using a defined learning process, and the algorithms' output becomes different when input is implemented. The algorithms processes are automated which aims to improve performance based on the data added.
There are three types of AI/ML-based SaMD modification.
1. Modification related to performance, with no change to the new use or new input type-
This type of modification results into the improvement in analytical and critical performance due to a number of factors. This includes re-training with new data sets, within the intended use population from the same type of input signal, a change in the AI/ML architecture and other means.
2. Modification related to inputs, with no change to the intended use- This modification involves the change in the inputs of AI/ML algorithm, with new types of input but without any alteration in the product use claims. The report cites the examples of this modification as in the compatibility of SaMD with CT Scanners from additional manufacturers.
3. Modifications related to the SaMD's intended use: This type of modification assists in concluding a definitive diagnosis. The outputs of this SaMD is governed by the healthcare situation or condition with limited scope due to pre-specified performance objectives change protocols.
The FDA has demonstrated certain examples where the SaMD applications can be applied. These are:
The paper proposes two modifications that can be made for this SaMD:
With the proposed modification, the researchers are hopeful for a possible breakthrough in the healthcare services with the help of the machine learning model. As they demand compliance by the manufacturers, the FDA is optimistic for the potential use of this model in the future.