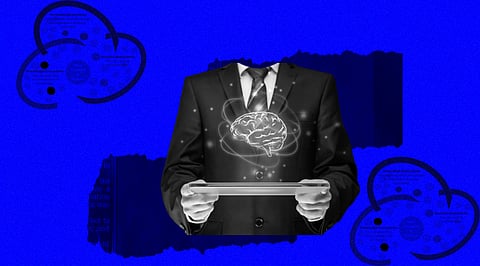
AI tools and systems that can learn to solve problems without human intervention have proven to be useful developments so far, but often companies have a hybrid approach called hybrid AI and you can benefit from it. Hybrid AI is a new development that combines non-symbolic AI, such as machine learning and deep learning systems, with symbolic AI or the embedding of human intelligence. As digital transformation initiatives drive the mainstream growth of AI, it's best to choose the right AI tools or methods for the right job. In many cases, you will need a combination of both. This is where hybrid AI applications come into play.
Hybrid AI is most commonly considered a combination of symbolic and non-symbolic AI, but the definition should include expertise. By injecting expert context into good algorithms, these algorithms are much more effective and powerful in solving real problems.
Here is a common use for hybrid AI in web search. When the user types "1GBP to USD", the search engine detects the currency conversion problem (symbolic AI) and runs machine learning to get, rank, and convert the web results (non-symbolic AI) before displaying and providing a widget to run.
There are dozens of such query classes processed by both symbolic and non-symbolic AI, such as weather, travel, and sports results. A major area of current development is self-driving cars. Self-driving cars need to understand the basic rules and process environmental signals to make real-time decisions.
People who have developed computer vision and language processing capabilities using deep learning are now rethinking their implementation with hybrid AI in mind. This is because some of these applications capture bias and identification signals from the underlying data and knowledge base. Insurance companies are also taking advantage of hybrid AI.
You can take a customer photo of the accident and use deep learning to "check" if the airbag has been deployed or what part of the vehicle is damaged. In many cases, this data is not directly available, so we use a deep computer vision model to generate the data. Traditional symbolic models that don't allow direct use of photos allow you to use the same symbols as if someone manually collected the data.
In such hybrid AI applications, deep learning models can learn to perform simpler tasks such as airbags and human detection, leaving complex inferences in traditional models that are more controllable by humans.
In-home insurance use cases, there may be models that warn customers about the most likely risks of their assets or recommend how AI handles claims based on the magnitude of the damage seen in the photo. So far, the two biggest benefits are a more reliable and easy-to-understand model and more data for modeling.
Intelligent AI hybrid systems can solve many complex problems related to the inaccuracy, uncertainty, ambiguity, and high dimensionality. Instead of learning everything from the data automatically, it combines both knowledge and data to solve the problem.
Intelligent hybrid systems can solve many complex problems related to inaccuracy, uncertainty, ambiguity, and high dimensionality. Instead of learning everything from the data automatically, it combines both knowledge and data to solve the problem. This type of problem requires on-the-fly humans to obtain weather forecasts and combine them with actual data such as location, wind speed, wind direction, and temperature to determine indoor travel. The logic of such a decision is not complicated. The missing part is this actual context.
Some people mistakenly believe that buying a graph database essentially provides a context for Artificial Intelligence. Most companies do not understand the intellectual, computational, carbon, and financial challenges of transforming real-world turmoil into contexts and connections that can be used for machine learning.
All interconnectivity produces an unprecedented amount of data. As organizations digitize, the use of AI tends to increase, allowing them to do more in less time. This can be to provide a better customer experience, reduce operating costs, or increase sales and profitability. However, success usually results in a clear understanding of the problem and the use of appropriate data and techniques to achieve the desired results.
Hybrid AI is a compromise. It turns out that deep learning is not universally superior because of all its power. Techniques are often combined to take advantage of the strengths and weaknesses of each approach, depending on the exact problem you want to solve and the constraints needed to solve it.