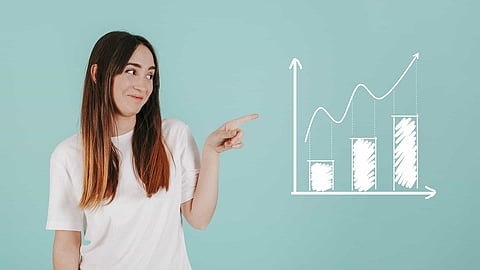
Artificial intelligence, machine learning and augmented analytics are terms we're currently observing everyday. Consistently, organizations worldwide make and gather new information, so organizations can't stand to sit inactive as they seek to turn out to be more data-driven companies to advance their business intelligence (BI) techniques. There has been demand from organizations, of all shapes and sizes, to become data-driven and their requirement for additional resources to accomplish that. There's a rising demand for data scientists and analysts who play a significant role in data wrangling, discovery, analysis, structuring, cleaning, validating and communicating data for projects and company needs.
Finding exceptionally trained data scientists to build the systems has demonstrated to be a troublesome task. Presently numerous companies are looking to citizen data scientists to assist them with finding significant bits of knowledge within big data.
While the idea of citizen data science isn't new, the training is still genuinely new to numerous organizations. The whole order of data science is still in its early stages, which implies the citizen data science movement is much earlier in its advancement. In spite of the curiosity of citizen data science, the adoption is developing rapidly on account of various factors, including trouble for discovering undeniable data scientists and consistent improvement in the quality of tools.
These users have data science-like aptitudes, however, aren't as cutting edge as data scientists. They're power users, for example, business analysts, who can perform both basic and decently complex tasks. Citizen data scientists hold a one of a kind point of view in their particular business territories to give context on what the visualizations and models highlighted in a dashboard mean. Any data-driven business, paying little mind to size, demands assets for extracting and acting on meaningful insights to facilitate the position of their organization.
Citizen data scientists do huge numbers of indistinguishable tasks from their higher trained partners, as indicated by Gartner examiner Carlie Idoine. In one of her blogs – "Resident Data Scientists and Why They Matter," she mentions that a citizen data scientist is someone who "makes or produces models that utilize advanced diagnostic analytics or predictive and prescriptive capabilities, however, whose essential job responsibility is outside the field of statistics and analytics. They don't supplant the specialists, as they don't have the particular, advanced data science expertise to do so. However, they unquestionably bring their OWN mastery and extraordinary aptitudes to the procedure."
Citizen data scientists are being acquainted with complex dashboards and requested to perform tasks that stretch their insight and ease with information. Visualization platforms outfit them with the devices to portray the information they're analyzing, yet they're still simply making the best conjecture dependent on their level of data expertise. NLG meets them there and fills the gap to separate the hindrance, guaranteeing that every user has a full logical comprehension of the data and the confidence to drive action.
NLG bolsters citizen data scientists by permitting any employee, paying little heed to the role, position or level of data expertise to decipher the data being introduced, what it implies and what action to take in a manner that interprets visuals utilizing the most natural form of communication, the written word.
DataRobot helps citizen data scientists swing over their weight through the power of automation. The organization's foundation basically sets up "guard rails" that manage analysts, power users, and even application developers through the machine learning process, including algorithms selection, feature engineering, model training, and deployment and ideally keeps them from committing errors.
While DataRobot empowers citizen data science by automating model management and accelerating training, it regularly leaves different parts of data science, explicitly the time-escalated data preparation and transformation tasks to other people. That is the place its partner Trifacta comes in.
Trifacta's Data Wrangler programming utilizes an assortment of methods (counting machine learning as well) to automate the data cleansing tasks. It's been very much reported that data scientists spend as much as 80% of their time physically preparing information to ensure the machine learning algorithms offer the most precise responses. What better approach to turbo-charge data science progress in an organization by putting the information-prep stage in the hands of citizen data scientists?
It's simpler to train subject matter experts to use data science software and BI platforms than it is to train data scientists to comprehend the complexities of numerous business areas. Raising up and engaging citizen data scientists, holding their master level of industry information, makes critical contributions to an inaccessible field of data science.
As you may expect, the challenge is less around the technology and more on human difficulties. Obviously, having human-focused technology that is easy to utilize and instinctive will go far towards managing the beginner to the "more profound end" of the analytic spectrum. However, it is likewise discerning that veritable success requires a mix of training programs to raise capable citizen data scientists, a culture of analytics and ability of the company to change – by trading out obsolete and dynamic procedures for a data-centric approach.
Blockbuster didn't go out of business since individuals there couldn't perform analytics, however rather, likely experienced the trouble in changing their present procedures fast enough. What's more, with that, Netflix, at last, beat them with analytics and data-driven processes. This is the top challenge in many organizations and for most information workers, the challenge of change.