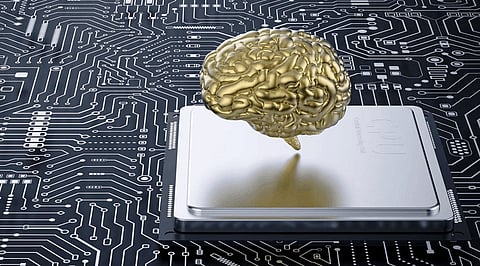
Artificial Intelligence and Machine Learning are being used interchangeably as a term across all segments of technological applications. Due to their close relation, AI is often confused with ML but one should not forget the distinction between the two. Out of all the differences, one is surely the biggest – that machine learning is a subset of AI. Technology professionals must understand the trivial difference both possess. Lacking the clarity between AI and ML, professionals as well as their companies may get misguided and eventually lose their relevance in the market with fake or misleading AI solutions.
According to an award-winning writer, Stephanie Overby, the most significant misunderstanding is how AI relates to ML. Where Artificial Intelligence is the umbrella term used to shelter many technologies, ML is one of its subsets. "AI is the broad container term describing the various tools and algorithms that enable machines to replicate human behavior and intelligence," explains JP Baritugo, director at management and IT consultancy Pace Harmon. There are numerous subsets of AI including Machine learning, natural language processing (NLP), deep learning, computer vision, and more.
For those who prefer analogies, Timothy Havens, the William and Gloria Jackson Associate Professor of Computer Systems in the College of Computing at Michigan Technological University and director of the Institute of Computing and Cybersystems, likens the way AI works to learning to ride a bike: "You don't tell a child to move their left foot in a circle on the left pedal in the forward direction while moving your right foot in a circle… You give them a push and tell them to keep the bike upright and pointed forward: the overall objective. They fall a few times, honing their skills each time they fail," Havens says. "That's Artificial Intelligence in a nutshell."
Machine learning is one way to accomplish that. The technology uses statistical analysis to learn autonomously and improve its function, explains Sarah Burnett, executive vice president, and distinguished analyst at a management consultancy and research firm Everest Group.
"[ML] uses various algorithms to analyze data, discern patterns, and generate the requisite outputs," says Pace Harmon's Baritugo, adding that machine learning is the capability that drives predictive analytics and predictive modeling.
Techies must understand that AI is a single thing. "AI is a collection of hundreds of different strands," says Wayne Butterfield, director of cognitive automation and innovation at ISG. "ML is a core component of many AI uses. It is the part of AI that enables a strand of AI to improve, whether that's improving an image recognition algorithm to recognize a cat versus a car, or speech recognition being able to understand multiple accents in a given language."
He further added, "While people in your organization may be sold to by salespeople who position Artificial Intelligence as a single thing, you need to push back on that notion."
This isn't easy, Butterfield notes. But the more IT leaders can clarify within their organizations what AI, ML, and other branches of cognitive capabilities are and aren't, and – even better ¬– do so within the context of business solutions, the fewer misunderstandings will recur.
"It will come as Artificial Intelligence becomes more commonly understood," Butterfield says. "The catch-all terms become less relevant as the nuances of the AI spectrum become much wider-known, and it is these aspects that we start to discuss in the future."
Join our WhatsApp Channel to get the latest news, exclusives and videos on WhatsApp
_____________
Disclaimer: Analytics Insight does not provide financial advice or guidance. Also note that the cryptocurrencies mentioned/listed on the website could potentially be scams, i.e. designed to induce you to invest financial resources that may be lost forever and not be recoverable once investments are made. You are responsible for conducting your own research (DYOR) before making any investments. Read more here.