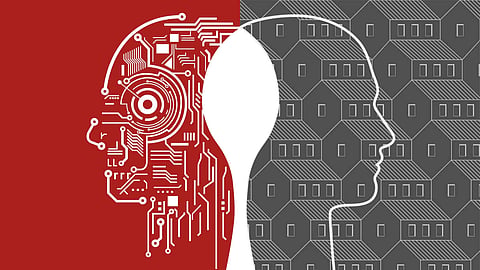
According to the International Institute for Analytics, by 2020, businesses using data will see US$430 billion in productivity benefits over competitors who are not using data. Therefore, as noted by Bernard Marr, an AI influencer, it's clear that data is now a key business asset, and it's revolutionizing the way companies operate, across most sectors and industries. In effect, every business, regardless of size, now needs to be a data business. And if every business is a data business, every business, therefore, needs a robust data strategy.
The idea behind developing a data strategy is to make sure all data resources are positioned in such a way that they can be used, shared, and moved easily and efficiently. Data is no longer a byproduct of business processing – it's a critical asset that enables processing and decision making. A data strategy helps by ensuring that data is managed and used as an asset. It provides a common set of goals and objectives across projects to ensure data is used both effectively and efficiently. A data strategy establishes common methods, practices, and processes to manage, manipulate, and share data across the enterprise in a repeatable manner.
While most companies have multiple data management initiatives underways (metadata, master data management, data governance, data migration, modernization, data integration, data quality, etc.), most efforts are focused on point solutions that address specific project or organizational needs. A data strategy establishes a road map for aligning these activities across each data management discipline in such a way that they complement and build on one another to deliver greater benefits.
According to Bernard Marr, to create a robust data and analytics strategy, business leaders need to consider many factors. Here are the critical points I would expect to see in a strong data strategy:
Your data needs – In order to find the right data for you, you must first define how you want to use data. You may need certain types of data for some goals and different types of data for others.
How you will source and gather the data – Having identified what you are looking to achieve with data, you can now start to think about sourcing and collecting the best data to meet those needs. There are many ways to source and collect data, including accessing or purchasing external data, using internal data, and putting in place new collection methods.
How that data will be turned into insights – As part of any solid data strategy, you need to plan how you will apply analytics to your data to extract business-critical insights that can inform decision making, improve operations and generate value.
Technology infrastructure requirements – Having decided how you want to use data, what kind of data is best for you, and how you might want to analyze that data, the next step in creating a robust data strategy is considering the technology and infrastructure implications of those decisions. Specifically, this means deciding on the software or hardware that will take your data and turn it into insights.
Data competencies within the organization – In order to get the most out of data it is essential to cultivate certain skills. There are two main routes to developing data-related competencies in your organization: boosting your in-house talent and outsourcing the data analysis.
Data governance – Collecting and storing data, especially personal data, brings serious legal and regulatory obligations. Therefore, it is vital any organization factor data ownership, privacy, and security issues into their data strategy. Ignoring these issues, or failing to properly address them, could see data go from a huge asset to a huge liability.
Furthermore, the best data strategies can flexibly apply to individual analytics applications as well as long-term, multiphase analytics programs.
Enterprise data standards are a strategy for managing data. The standards prescribe metrics for the quality of data in most data sets, methods for modeling data in certain contexts, and the preferred interfaces for data in motion.
Data governance policies are a strategy for assuring regulatory compliance and data privacy. A policy specifies which users or user roles can access which data sets, domains, and applications.
A policy may also specify preferred techniques for protecting sensitive data (such as encryption and masking) or methodologies for executing governance (such as a data strategy establishes a road map for aligning these activities across each data management discipline in such a way that they complement and build on one another to deliver greater benefits and rewards & curation).
Technical data strategies relative to data platforms can be driven by data's structure or use case. For example, relational data for analytics is typically managed by a relational database management system (RDBMS), whereas file-based, large volume, raw source, and unstructured data may be managed by Hadoop. As another example, TDWI sees many organizations storing analytics data for reporting, dashboards, and OLAP in a traditional data warehouse on-premises, whereas data for advanced forms of analytics is progressively managed on public clouds. Such preferences can constitute a data strategy.
Join our WhatsApp Channel to get the latest news, exclusives and videos on WhatsApp
_____________
Disclaimer: Analytics Insight does not provide financial advice or guidance. Also note that the cryptocurrencies mentioned/listed on the website could potentially be scams, i.e. designed to induce you to invest financial resources that may be lost forever and not be recoverable once investments are made. You are responsible for conducting your own research (DYOR) before making any investments. Read more here.