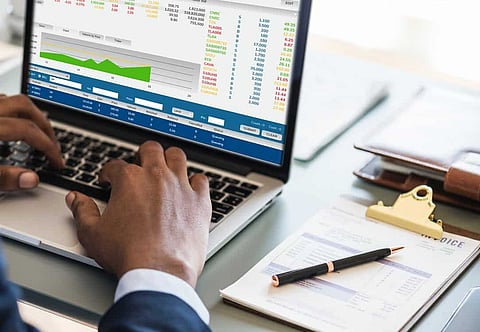
Data analytics whether big or small is to get deepest insights resulting in smarter decisions and better outcomes. The business insights required will vary according to the technology and the data analysis processes used. It is of prime importance to design and build a data warehouse or business intelligence architecture that provides a flexible, multi-faceted analytical ecosystem optimized for efficient ingestion and analysis of diverse datasets. This article will walk through the three most important analyses.
Descriptive analysis looks into the data and analyze the past events for insights which helps to approach the future. Descriptive analysis considers the past performance and understands the nature of the performance by mining historical data to look for the reasons behind the past success or failure. Descriptive models solidify the relationships in data in a way that is often used to classify the parameters or prospects into groups. Say, for example, a predictive model which emphasize on predicting a single customer behavior, descriptive models identify different relationship between customers and products
In marketing descriptive models can be used to categorize customers by their product preference and life stage. Descriptive modeling tools can be used to develop further models that can simulate a large number of individualized agents and forecast the predictions. For example, descriptive analytics studies the historical electricity usage data to plan the power requirement in advance and allow companies to set an optimum price.
Diagnostic Analytics is an advanced level of analytics which dissects the data to answer the question "Why did it happen". It is characterized by methods such as drill down, data discovery, data mining and correlations. Most companies go for diagnostic analytics, as it gives a deep insight into a particular problem. At the same time, a company should have detailed information at their disposal; otherwise, data collection may turn out to be individual for every issue and time-consuming.
Say, for example, a healthcare provider compares patients' response to a promotional campaign in different regions to perform promotional activities; a retailer drills the sales down to subcategories. Another example widely used in the healthcare industry is customer segmentation coupled with several filters applied (like diagnosis and prescribed medications) allowed measuring the risk of hospitalization.
Predictive Analytics converts data into valuable insights or actionable information. It uses data to determine the probable future outcome of an event or a chance of situation occurring. Predictive analytics engulfs a variety of statistical techniques from modeling, machine learning, data mining and game theory that analyze current and historical facts to make predictions about future events. In the complex business scenario, predictive models exploit the pattern in historical data to recognize the risk and opportunities. The model reveals relationship among many factors to allow assessment of risk or potential associated with a particular set of conditions, which helps in decision-making for the candidate transactions.
There are three stepping stones for predictive analysis–predictive modeling, decision analysis and optimization and transaction profiling.
The ubiquitous example of predictive analysis is optimizing the customer management system. This enables the organization to analyze all the customer data and finally revealing the patterns that predict customer behavior. Another example which is mostly used in business that offers an array of products is that predictive analytics can analyze customer spending and other behavior leading to efficient cross sales or selling additional products to current customers. This directly leads to higher profitability per customer and stronger customer relationships.
Join our WhatsApp Channel to get the latest news, exclusives and videos on WhatsApp
_____________
Disclaimer: Analytics Insight does not provide financial advice or guidance. Also note that the cryptocurrencies mentioned/listed on the website could potentially be scams, i.e. designed to induce you to invest financial resources that may be lost forever and not be recoverable once investments are made. You are responsible for conducting your own research (DYOR) before making any investments. Read more here.