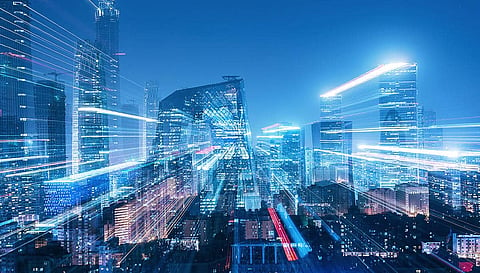
The energy sector that forms an integral part of our daily lives collects magnanimous amounts of data on a continuous basis through sensors, network communication, wireless transmission, and cloud computing technologies. The amount of data collected on a daily basis on the supply and demand side is quite staggering. With the increased adaptability of intelligent devices and services, power giants are sitting on a gold mine of data. This data, when put into analysis, will help power giants and administration to plan energy needs for the next generation. Data is created not only on the consumption end but during the whole process of power generation, transmission and distribution.
Data is of value only if businesses and utilities use this "Big Data" in an efficient manner. Utilities are using big data analytics deploying predictive analytics models to drive down costs, reduce carbon emissions and improve operational efficiencies.
Energy Sector is increasingly harnessing the power of the mammoth data through Big Data Analytics to optimize power generation and improve planning. The two most important decision-making processes in the power industry are power generation planning and economic load management. Putting into simpler words, analytics through predictive data is focussing to match power supply with the demand for energy harnessed from the grid over a period of time. Matching energy supply and demand on the grid has been a tough challenge and analytics has a big role to play. Energy companies are taking advantage of the collected energy data to leverage predictive analytics techniques to improve distribution and lessen production costs.
If you thought that Predictive Analytics models are deployed to non-renewable sources of energy like coal, petroleum, and natural gas you are wrong! Predictive Analytics is also maneuvering wind power and solar power to significantly affect their outputs. Through data analytics, renewable energy power generation forecasting has a huge potential to be more accurate and efficient. The integration of data from energy production like the weather data including temperature, cloud cover, wind speed, atmospheric pressure, humidity, and wind direction can be assessed for effective power planning.
Geographical data is another aspect of predictive analytics which includes geographical information from satellite imagery that aids spatial planning. Power management has a scope for improvement through the analysis of topography, location and solar irradiation etc.
The energy utility industry has been an asset-intensive industry since time immemorial. The industry is faced with asset management challenges like resource sharing, operation and maintenance management, asset retirement monitoring, inventory management and procurement monitoring. The efficiency of asset management and collaborative operation energy has a long way to go with big data analytics.
Data analytics automates the process of managing Energy Demand. This process is important since it is the core of energy consumption, from a factory, an office block, farm or even a retail store. Much of the advances that have been witnessed in the energy sector have been on the demand side. It is the technology behind the energy efficient design of electrical devices to reduce power requirements. In the times to come, energy efficiency will play a big role as the solution to reduce global greenhouse emissions. Energy giants are looking for solutions to reduce energy costs and contribute to sustainable energy usage. Enter predictive analytics, the data generated by meters, costs, business policies, production figures, asset operations when integrated will prove to be a sustainable solution to manage the energy demand for future generations. Powerful inferences like how to minimize energy leakages and reduce consumers energy bills will soon be a reality.
With advances in technology and analytics, energy consumption can even be forecasted, to predict customer's energy savings with a lot of energy management software packages available in the market. Analysis of this data will show patterns in energy consumption and can be put forward to forecast the energy trends of the future. Data analytics also play a dominant role in identifying projects that have the potential to save the maximum energy and investment. The data analytic results from these energy management systems are real-time and provide energy managers with proactive tools to identify live energy issues.
Major research analysis has come to a conclusion that combining Big Data and predictive analytics could point to cheap energy at some point in time. Matching energy supply and demand has the potential to supply cost-effective power and this is only the beginning. The concept of cheaper energy starts with empowering customers to store unused power and sell it back to the grid, implying to recycle energy itself. Harnessing the full potential of predictive analytics, free energy may be still far off, but is a probable reality soon.