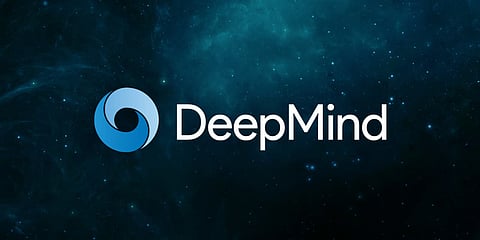
A report published by Google's DeepMind researchers revealed that the state-of-art of DeepMind technology does not perform fairly well at High School Maths. The researchers then experimented to train the neural network to solve simple problems including arithmetic, calculus, and algebra. These are the basic plot over which a high school student easily be tested. In the standard test comprised of 40 questions when presented in front of DeepMind's neural network, it managed to get 14 out of 40 in it which is equivalent to E grade for a British high schooler.
This clearly shows that AI is going through a rough phase in learning some basic math.
As ImageNet was designed as an image recognition set test, similarly the paper for analyzing the mathematical ability of the neural network was set as a benchmark test. The paper named "Analysing Mathematical Reasoning Abilities of Neural Models" was created by David Saxton, Edward Grefenstette, Felix Hill and Pushmeet Kohli of DeepMind which is posted on an arXiv preprint server.
The authors argue for research and investigation into the fact that why humans are sound enough to perform well in discrete compositional reasoning about objects which is algebraically generalized.
The authors also offered a diverse pool of math problems that should compel neural network to gain a level of reasoning which includes things like planning and identifying the functions in correct order to compose when the mathematical problem is divided into parts which can be either associative, distributive or commutative.
The authors came up with a range of questions which did not include geometry and none of them were verbal like the following pattern:
Solve -42*r + 27*c = -1167 and 130*r + 4*c = 372 for r.
Answer: 4
The test paper was based on a National Mathematics Curriculum which can be solved by a student of 16 years of age. The paper was restricted to textual questions excluding geometry. The textual questions gave a comprehensive range of mathematics topics that collaboratively work as a piece of a learning curriculum.
The training of the neural network model could have done on neural net math abilities but the whole idea behind the experiment was to train it from nothing to build a basic mathematical ability which resulted in the creation of less standard neural network test paper.
The concept behind the testing was to evaluate general knowledge of the neural network rather than inbuilt knowledge.
The researchers said – "What makes such models (which are invariably neural architectures) so ubiquitous from translation to parsing via image captioning is the lack of bias these function approximators present due to having relatively little (or no) domain-specific knowledge encoded in their design."
Generally, neural networks perform fairly well when it comes to finding place value in a long number, rounding off decimal numbers and sorting sequences and arranging a series of numbers in proper order.
For such systems, the toughest problems are those including number-theoretic questions let's say fractionation, breaking down numbers into constituent parts and guessing if the number is prime or not.
Although human's also face issues while going through these problems which are not that surprising.
The other problems that which become an issue with the neural network are a combination of mixed arithmetic where four operations occur in an array. This results in a drop in the machine's accuracy percentage to 50%.
Overall, a bunch of real-world problem from a high school curriculum in which the neural network depicted performance equivalent to E grade is disappointing and moderate. The researchers conclude that – "While the Transformer neural net we build performs better than the LSTM variant, neither of the networks are doing much "algorithmic reasoning," and the models do not learn to do any algebraic/algorithmic manipulation of values, and are instead learning relatively shallow tricks to obtain good answers on many of the modules."
As for now, the researchers have a data set which can be a baseline for training more kinds of networks. These data sets are easily extended which would let researchers go straight up to university level mathematics.